Fresh Content Needs More Attention: Multi-funnel Fresh Content Recommendation
PROCEEDINGS OF THE 29TH ACM SIGKDD CONFERENCE ON KNOWLEDGE DISCOVERY AND DATA MINING, KDD 2023(2023)
摘要
Recommendation system serves as a conduit connecting users to an incredibly large, diverse and ever growing collection of contents. In practice, missing information on fresh (and tail) contents needs to be filled in order for them to be exposed and discovered by their audience. We here share our success stories in building a dedicated fresh content recommendation stack on a large commercial platform. To nominate fresh contents, we built a multi-funnel nomination system that combines (i) a two-tower model with strong generalization power for coverage, and (ii) a sequence model with near real-time update on user feedback for relevance. The multi-funnel setup effectively balances between coverage and relevance. An in-depth study uncovers the relationship between user activity level and their proximity toward fresh contents, which further motivates a contextual multi-funnel setup. Nominated fresh candidates are then scored and ranked by systems considering prediction uncertainty to further bootstrap content with less exposure. We evaluate the benefits of the dedicated fresh content recommendation stack, and the multi-funnel nomination system in particular, through user corpus co-diverted live experiments. We conduct multiple rounds of live experiments on a commercial platform serving billion of users demonstrating efficacy of our proposed methods.
更多查看译文
关键词
Hybrid Recommendation Systems,Cold-start Recommendation,Real-time Learning,Content Generalization
AI 理解论文
溯源树
样例
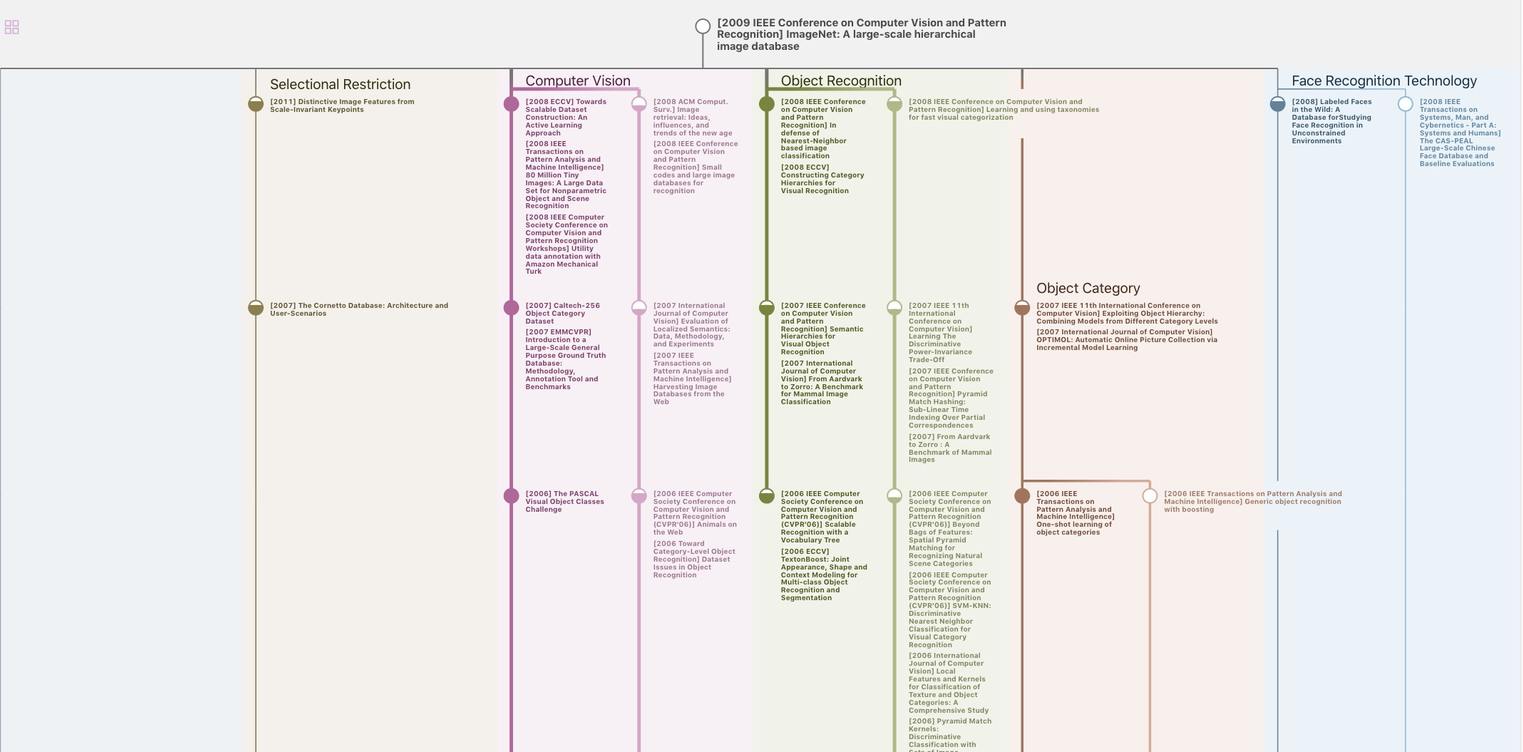
生成溯源树,研究论文发展脉络
Chat Paper
正在生成论文摘要