Towards Robust FastSpeech 2 by Modelling Residual Multimodality
CoRR(2023)
摘要
State-of-the-art non-autoregressive text-to-speech (TTS) models based on FastSpeech 2 can efficiently synthesise high-fidelity and natural speech. For expressive speech datasets however, we observe characteristic audio distortions. We demonstrate that such artefacts are introduced to the vocoder reconstruction by over-smooth mel-spectrogram predictions, which are induced by the choice of mean-squared-error (MSE) loss for training the mel-spectrogram decoder. With MSE loss FastSpeech 2 is limited to learn conditional averages of the training distribution, which might not lie close to a natural sample if the distribution still appears multimodal after all conditioning signals. To alleviate this problem, we introduce TVC-GMM, a mixture model of Trivariate-Chain Gaussian distributions, to model the residual multimodality. TVC-GMM reduces spectrogram smoothness and improves perceptual audio quality in particular for expressive datasets as shown by both objective and subjective evaluation.
更多查看译文
关键词
robust fastspeech,residual multimodality
AI 理解论文
溯源树
样例
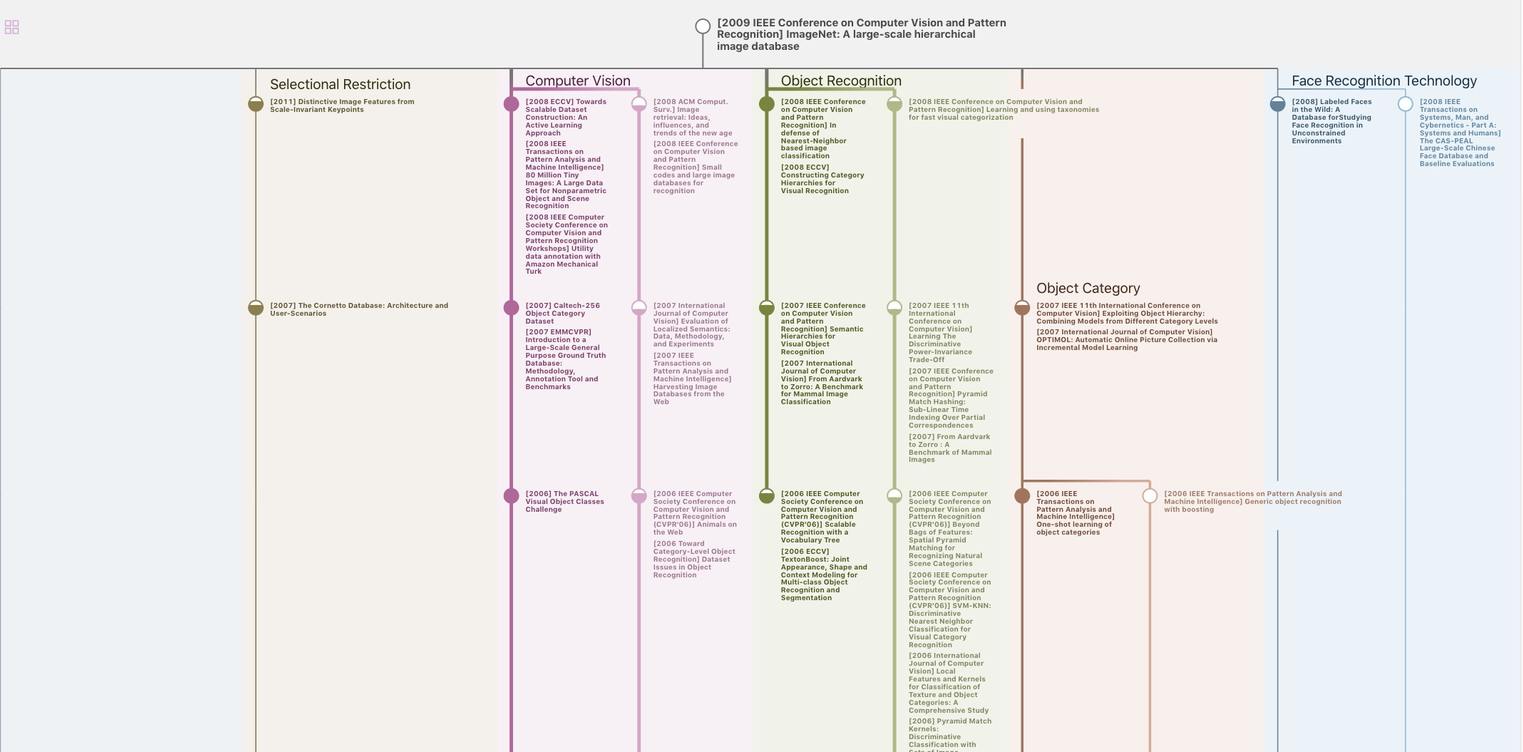
生成溯源树,研究论文发展脉络
Chat Paper
正在生成论文摘要