Physics-Augmented Data-EnablEd Predictive Control for Eco-Driving of Mixed Traffic Considering Diverse Human Behaviors
IEEE TRANSACTIONS ON CONTROL SYSTEMS TECHNOLOGY(2024)
摘要
Data-driven cooperative control of connected and automated vehicles (CAVs) has gained extensive research interest as it can utilize collected data to generate control actions without relying on parametric system models that are generally challenging to obtain. Existing methods mainly focused on improving traffic safety and stability, while less emphasis has been placed on energy efficiency in the presence of uncertainties and diversities of human-driven vehicles (HDVs). In this brief, we employ a Data-EnablEd Predictive Control (DeePC) scheme to address the eco-driving of mixed traffic flows with diverse behaviors of human drivers. Specifically, by incorporating first-order physics, we propose the physics-augmented DeePC (PA-DeePC) approach to handle substantial system variations and uncertainties. Then, a novel optimization framework, avoiding conventional reference trajectories during the receding optimization process, is proposed to further reduce the holistic energy consumption of mixed traffic flows. Simulation results demonstrate the effectiveness of our approach in accurately capturing random human driver behaviors and reducing holistic energy consumption, while ensuring driving safety and traffic efficiency. Furthermore, the proposed optimization framework achieves substantial reductions in energy consumption, i.e., average reductions of $4.14\%$ , $4.97\%$ , and $9.29\%$ when compared to the benchmark algorithms.
更多查看译文
关键词
Connected and automated vehicles (CAVs),data-driven control,diverse driving behaviors,eco-driving
AI 理解论文
溯源树
样例
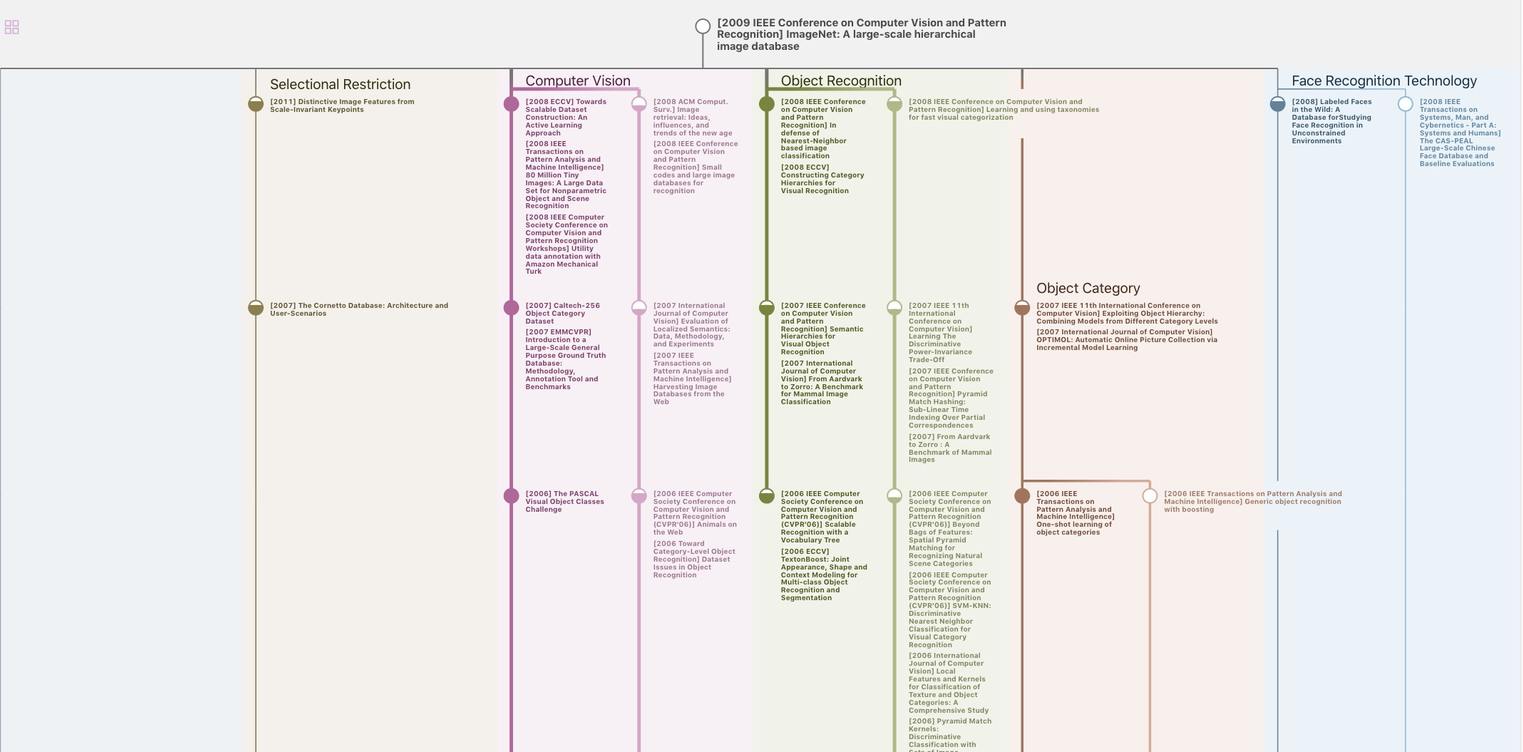
生成溯源树,研究论文发展脉络
Chat Paper
正在生成论文摘要