Revisiting Hate Speech Benchmarks: From Data Curation to System Deployment
PROCEEDINGS OF THE 29TH ACM SIGKDD CONFERENCE ON KNOWLEDGE DISCOVERY AND DATA MINING, KDD 2023(2023)
摘要
Social media is awash with hateful content, much of which is often veiled with linguistic and topical diversity. The benchmark datasets used for hate speech detection do not account for such divagation as they are predominantly compiled using hate lexicons. However, capturing hate signals becomes challenging in neutrally-seeded malicious content. Thus, designing models and datasets that mimic the real-world variability of hate warrants further investigation. To this end, we present GOTHate, a large-scale code-mixed crowdsourced dataset of around 51k posts for hate speech detection from Twitter. GOTHate is neutrally seeded, encompassing different languages and topics. We conduct detailed comparisons of GOTHate with the existing hate speech datasets, highlighting its novelty. We benchmark it with 10 recent baselines. Our extensive empirical and benchmarking experiments suggest that GOTHate is hard to classify in a text-only setup. Thus, we investigate how adding endogenous signals enhances the hate speech detection task. We augment GOTHate with the user's timeline information and ego network, bringing the overall data source closer to the real-world setup for understanding hateful content. Our proposed solution HEN-mBERT is a modular, multilingual, mixture-of-experts model that enriches the linguistic subspace with latent endogenous signals from history, topology, and exemplars. HEN-mBERT transcends the best baseline by 2.5% and 5% in overall macro-F1 and hate class F1, respectively. Inspired by our experiments, in partnership with Wipro AI, we are developing a semi-automated pipeline to detect hateful content as a part of their mission to tackle online harm.
更多查看译文
关键词
Online Hate Speech,Hindi-Codemixed Dataset,Benchmark Comparision,Endogenous Signal Modeling,Content Moderation Tool
AI 理解论文
溯源树
样例
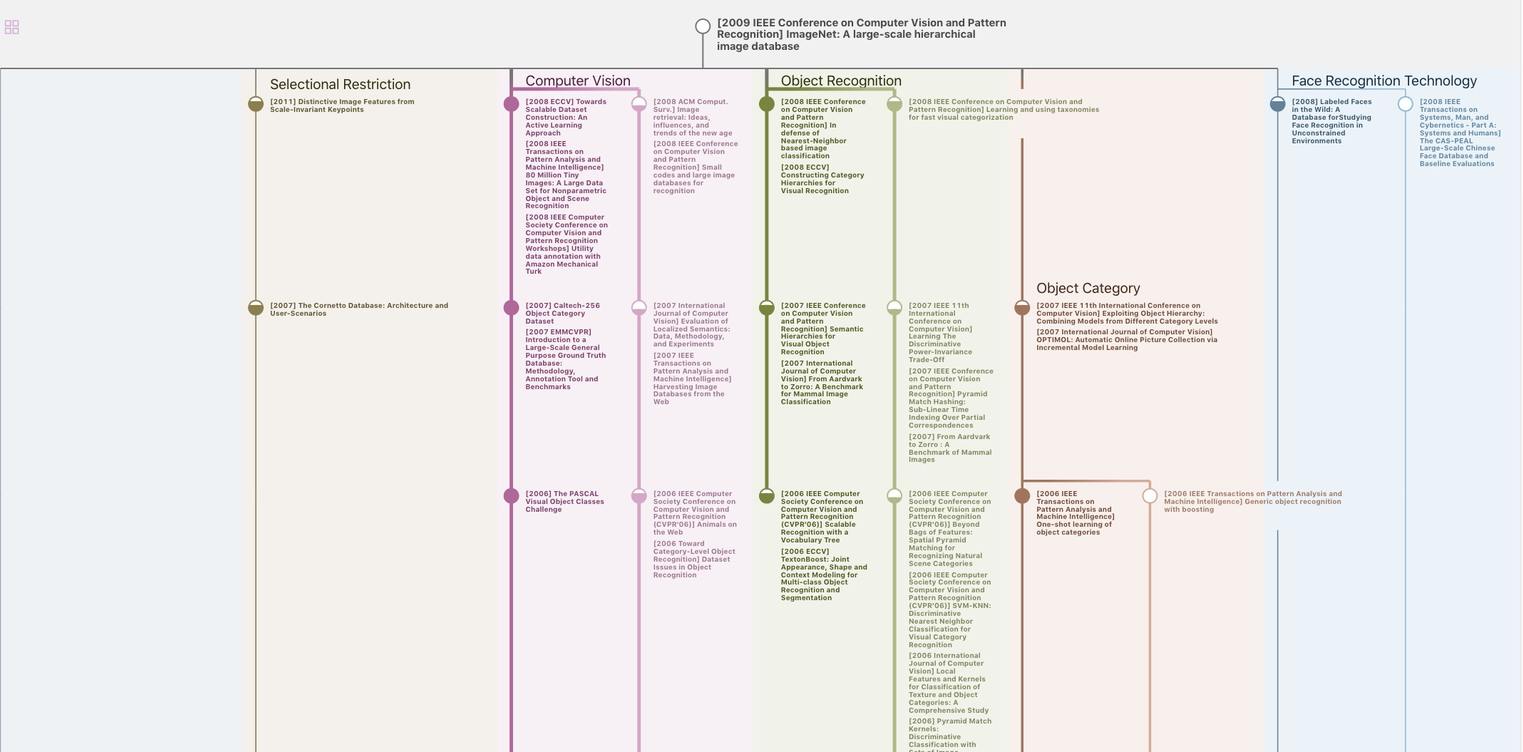
生成溯源树,研究论文发展脉络
Chat Paper
正在生成论文摘要