4DSR-GCN: 4D Video Point Cloud Upsampling using Graph Convolutional Networks
PROCEEDINGS OF THE 1ST INTERNATIONAL WORKSHOP ON MULTIMEDIA CONTENT GENERATION AND EVALUATION, MCGE 2023: New Methods and Practice(2023)
摘要
Time varying sequences of 3D point clouds, or 4D point clouds, are now being acquired at an increasing pace in several applications (e.g., personal avatar representation, LiDAR in autonomous or assisted driving). In many cases, such volume of data is transmitted, thus requiring that proper compression tools are applied to either reduce the resolution or the bandwidth. In this paper, we propose a newsolution for upscaling and restoration of time-varying 3D video point clouds after they have been heavily compressed. Our model consists of a specifically designed Graph Convolutional Network that combines Dynamic Edge Convolution and Graph Attention Networks for feature aggregation in a Generative Adversarial setting. We present a different way to sample dense point clouds with the intent to make these modules work in synergy to provide each node enough features about its neighbourhood in order to later on generate new vertices. Compared to other solutions in the literature that address the same task, our proposed model is capable of obtaining comparable results in terms of quality of the reconstruction, while using a substantially lower number of parameters (similar or equal to 300KB), making our solution deployable in edge computing devices.
更多查看译文
关键词
Time varying 3D point clouds,3D upscaling,Graph Attention Network,Generative Adversarial setting,Super Resolution
AI 理解论文
溯源树
样例
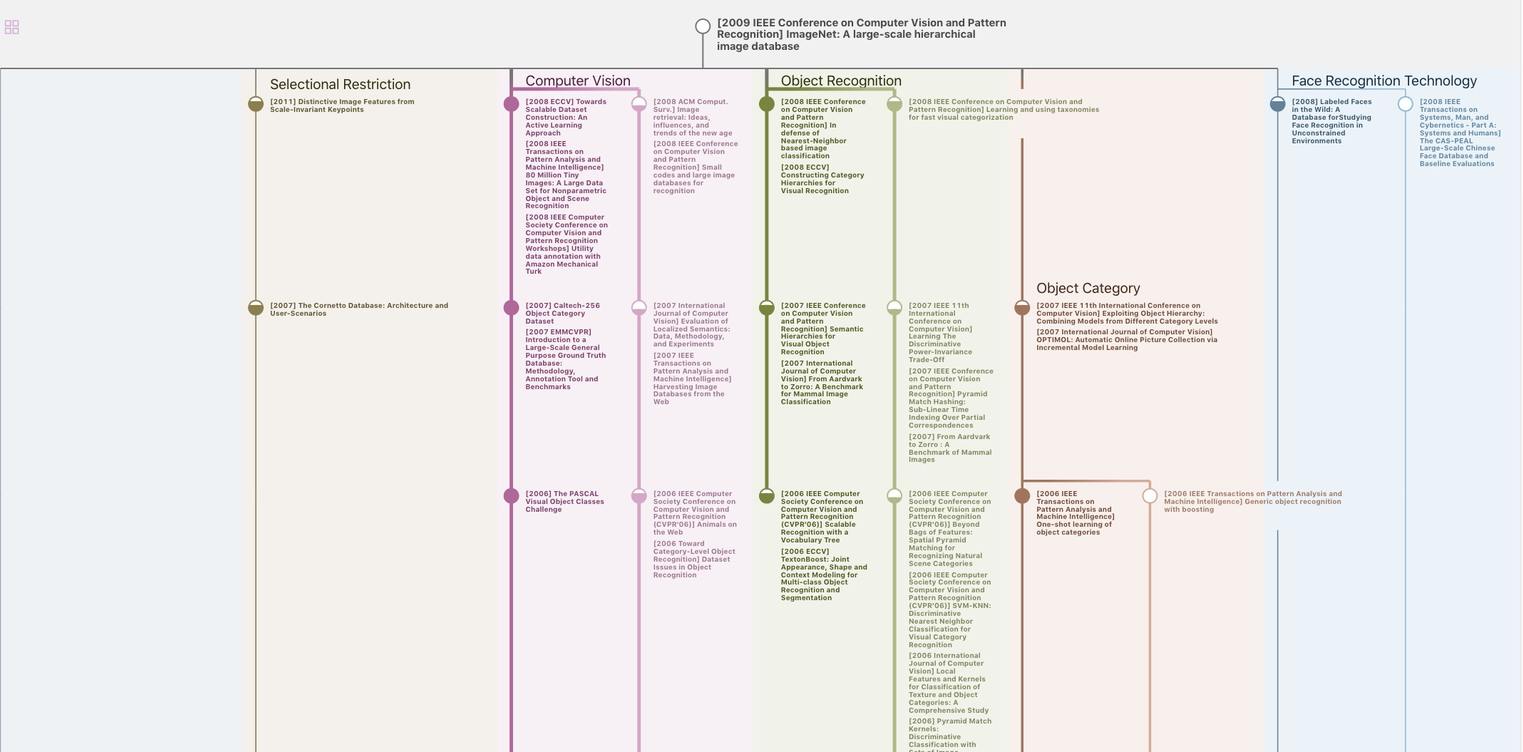
生成溯源树,研究论文发展脉络
Chat Paper
正在生成论文摘要