Chaos persists in large-scale multi-agent learning despite adaptive learning rates
CoRR(2023)
摘要
Multi-agent learning is intrinsically harder, more unstable and unpredictable than single agent optimization. For this reason, numerous specialized heuristics and techniques have been designed towards the goal of achieving convergence to equilibria in self-play. One such celebrated approach is the use of dynamically adaptive learning rates. Although such techniques are known to allow for improved convergence guarantees in small games, it has been much harder to analyze them in more relevant settings with large populations of agents. These settings are particularly hard as recent work has established that learning with fixed rates will become chaotic given large enough populations.In this work, we show that chaos persists in large population congestion games despite using adaptive learning rates even for the ubiquitous Multiplicative Weight Updates algorithm, even in the presence of only two strategies. At a technical level, due to the non-autonomous nature of the system, our approach goes beyond conventional period-three techniques Li-Yorke by studying fundamental properties of the dynamics including invariant sets, volume expansion and turbulent sets. We complement our theoretical insights with experiments showcasing that slight variations to system parameters lead to a wide variety of unpredictable behaviors.
更多查看译文
关键词
chaos,multi-agent multi-agent,learning rates,large-scale
AI 理解论文
溯源树
样例
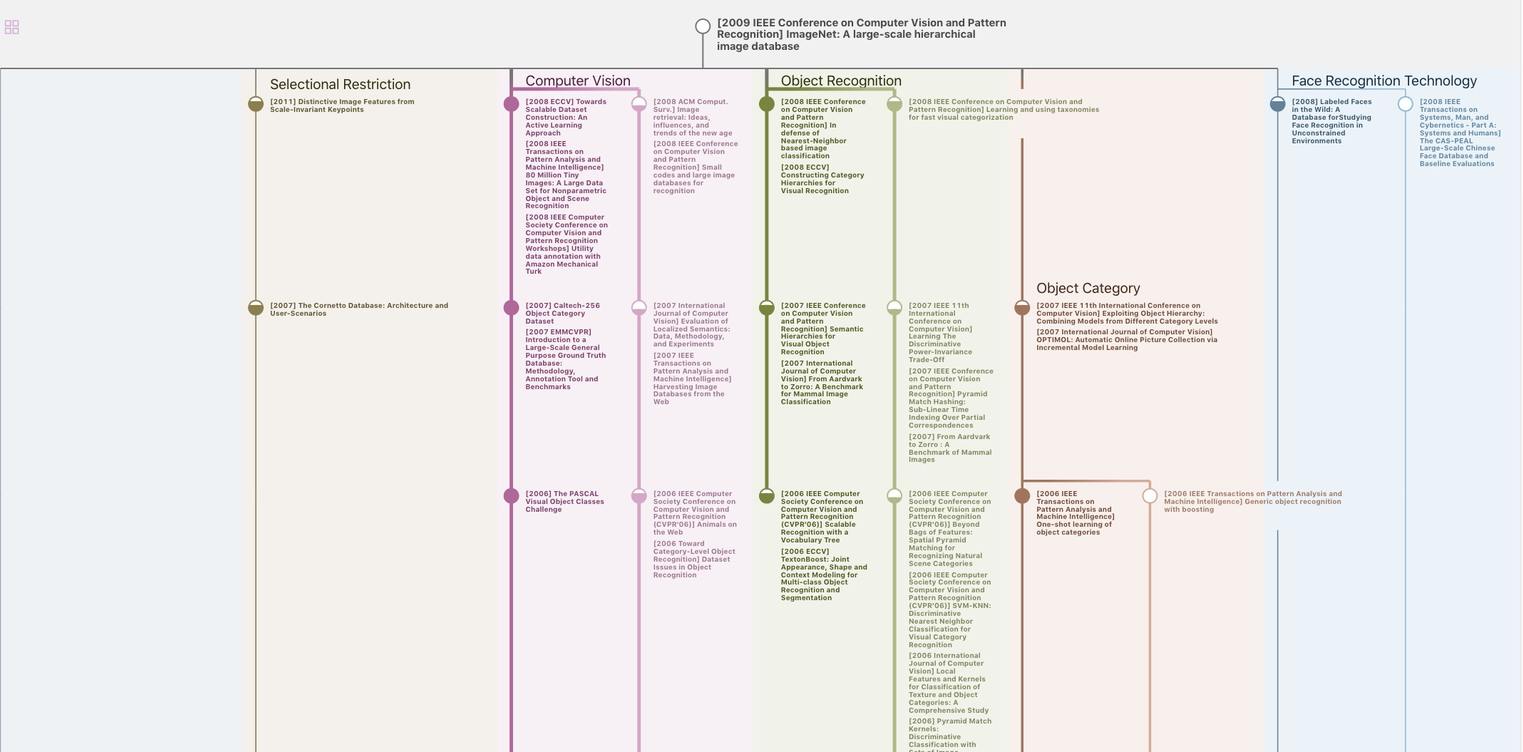
生成溯源树,研究论文发展脉络
Chat Paper
正在生成论文摘要