Machine Learning-Based Adaptive Outlier Detection for Underkill Reduction in Analog/RF IC Testing
2023 IEEE 41st VLSI Test Symposium (VTS)(2023)
摘要
We present a solution for reducing the number of defective analog/RF integrated circuits (ICs) that escape detection during manufacturing testing. Also known as underkill, these ICs may fail when deployed in their target application and eventually become customer returns, casting doubt on the effectiveness of the employed test solution and affecting the bottom line. To ameliorate this problem, we introduce an adaptive outlier detection solution that identifies ICs which are suspect of becoming customer returns and proactively bins them as failing. The outlier detection boundary used by our method is dynamically computed based on the performance distribution of devices on each wafer and the underlying model is updated when new ICs are returned from customers and failure analysis confirms that they are indeed defective devices. The effectiveness of our method in reducing underkill while minimizing the incurred yield loss is evaluated using an industrial dataset from Texas Instruments.
更多查看译文
AI 理解论文
溯源树
样例
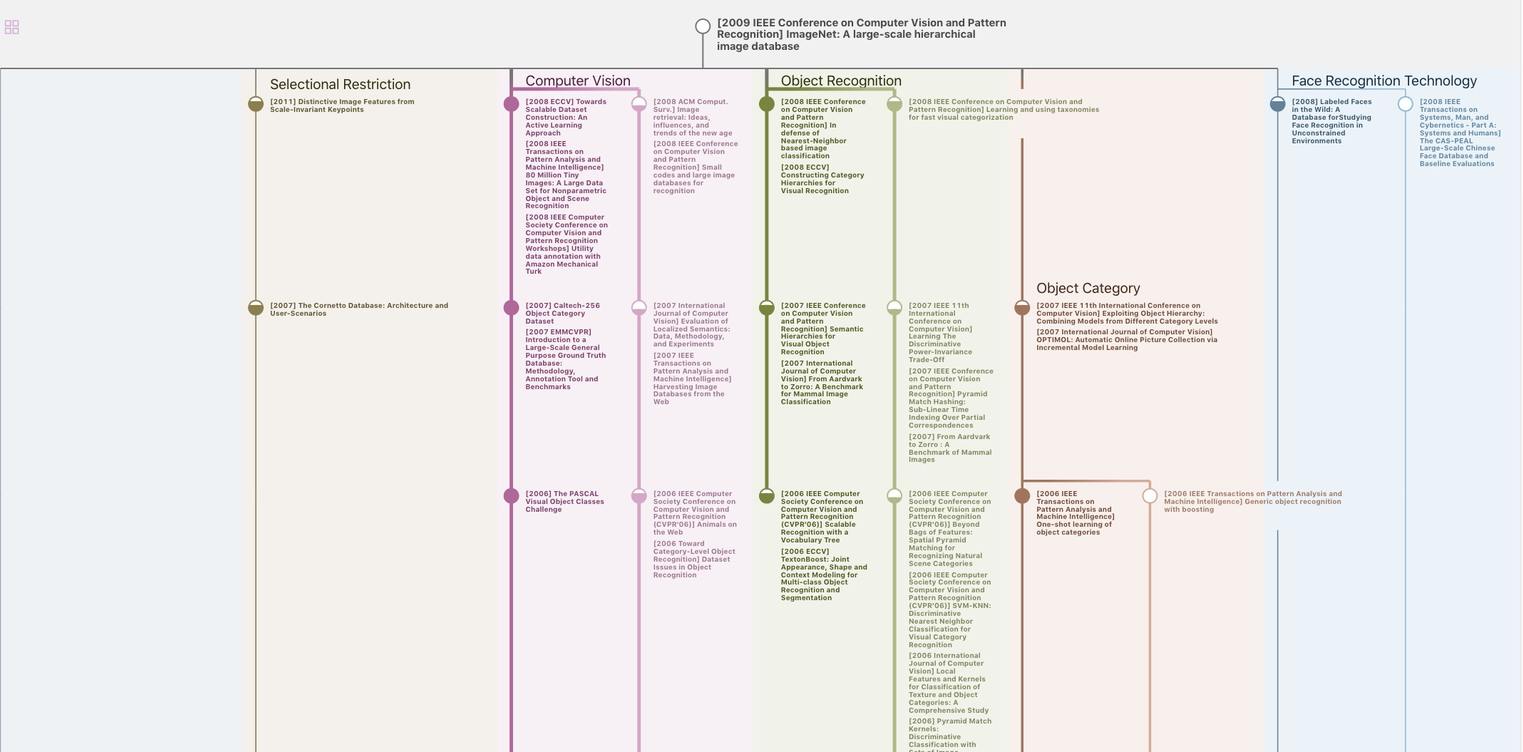
生成溯源树,研究论文发展脉络
Chat Paper
正在生成论文摘要