Double-branch fusion network with a parallel attention selection mechanism for camouflaged object detection.
Sci. China Inf. Sci.(2023)
摘要
To meet the challenge of camouflaged object detection (COD), which has a high degree of intrinsic similarity between the object and background, this paper proposes a double-branch fusion network (DBFN) with a parallel attention selection mechanism (PASM). In detail, a schismatic receptive field block (SRF) combined with an attention mechanism for low-level information is performed to learn texture features in one branch, and an integration of the SRF, a hybrid attention mechanism (HAM), and a depth feature polymerization module (DFPM) is employed for high-level information to extract detection features in the other branch. Then, both texture features and detection features are input into the PASM to acquire selective expression matrices. Eventually, the final result is obtained after further selective matrix optimization with atrous spatial pyramid pooling (ASPP) and a residual channel attention block (RCAB) being applied serially. Experimental results on three public datasets verify that our method outperforms the state-of-the-art methods in terms of four evaluation metrics, i.e., mean absolute error (MAE), weighted F fi measure (F-beta(omega)), structural measure (S-alpha), and E- measure (E )
更多查看译文
关键词
camouflaged object detection,attention mechanism,feature extraction,feature aggregation,texture information,fuzzy boundary
AI 理解论文
溯源树
样例
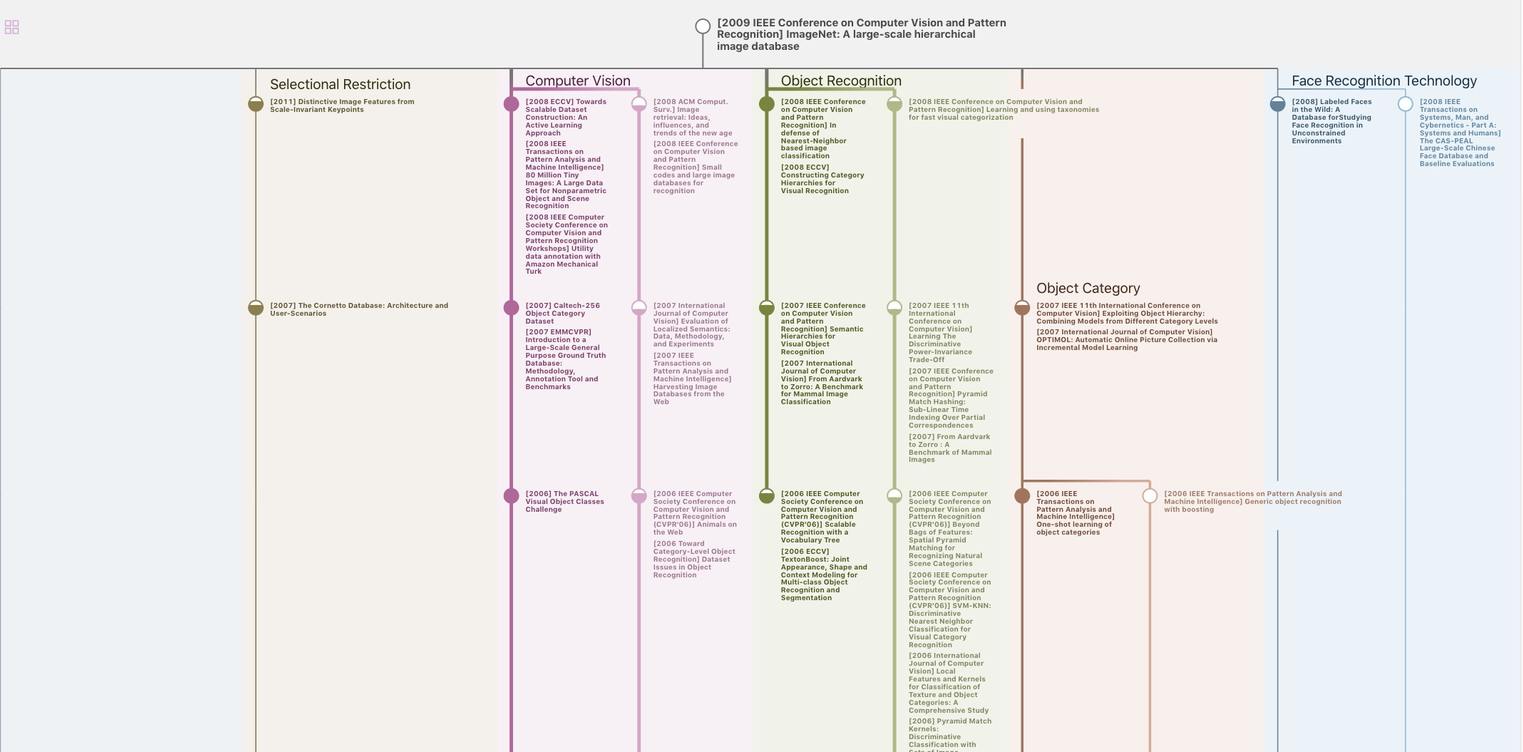
生成溯源树,研究论文发展脉络
Chat Paper
正在生成论文摘要