Distribution-free Phase-II monitoring of high-dimensional industrial processes via origin and modified interpoint distance based algorithms.
Comput. Ind. Eng.(2023)
摘要
Improvements in measuring devices, the development of sensing technologies, automated record-keeping and cloud storage facilities have offered us a large volume of data streams involving many variables for data-driven decision-making. Consequently, monitoring high-dimensional (HD) processes has become an essential and exciting topic in statistical process monitoring. HD monitoring poses a significant challenge because considerable uncertainty is involved in estimating process parameters when an adequately large reference sample is unavailable before monitoring. Thus, designing an effective, conveniently implementable monitoring scheme for the HD processes remains a challenging task that has yet to be effectively addressed. This paper develops two distribution-free exponentially weighted moving average (EWMA) schemes to monitor multivariate and HD processes based on origin and interpoint distances. Previous works on interpoint distance-based charts are generally not distribution-free unless only one reference point is used as a benchmark to compute the interpoint distances. We offer an improved and general way to achieve conditionally distribution-free properties closely. The proposal also combines the advantage of the nonparametric approaches for jointly monitoring the process location and scale parameters with the better shift detection performance of an EWMA scheme. The new EWMA schemes can be easily implemented even when the data dimension is large with a small number of Phase-I samples. Control limits using the median run length as a metric are evaluated and tabulated for some cases to implement the proposed chart quickly. Extensive comparative studies have been conducted to verify the efficacy of the proposed monitoring schemes. Finally, the proposed schemes are illustrated with a real problem of monitoring high-dimensional quality characteristics from semiconductor manufacturing processes.
更多查看译文
关键词
Distribution-free,High-dimensional processes,Interpoint distance statistical process monitoring,Semiconductor quality
AI 理解论文
溯源树
样例
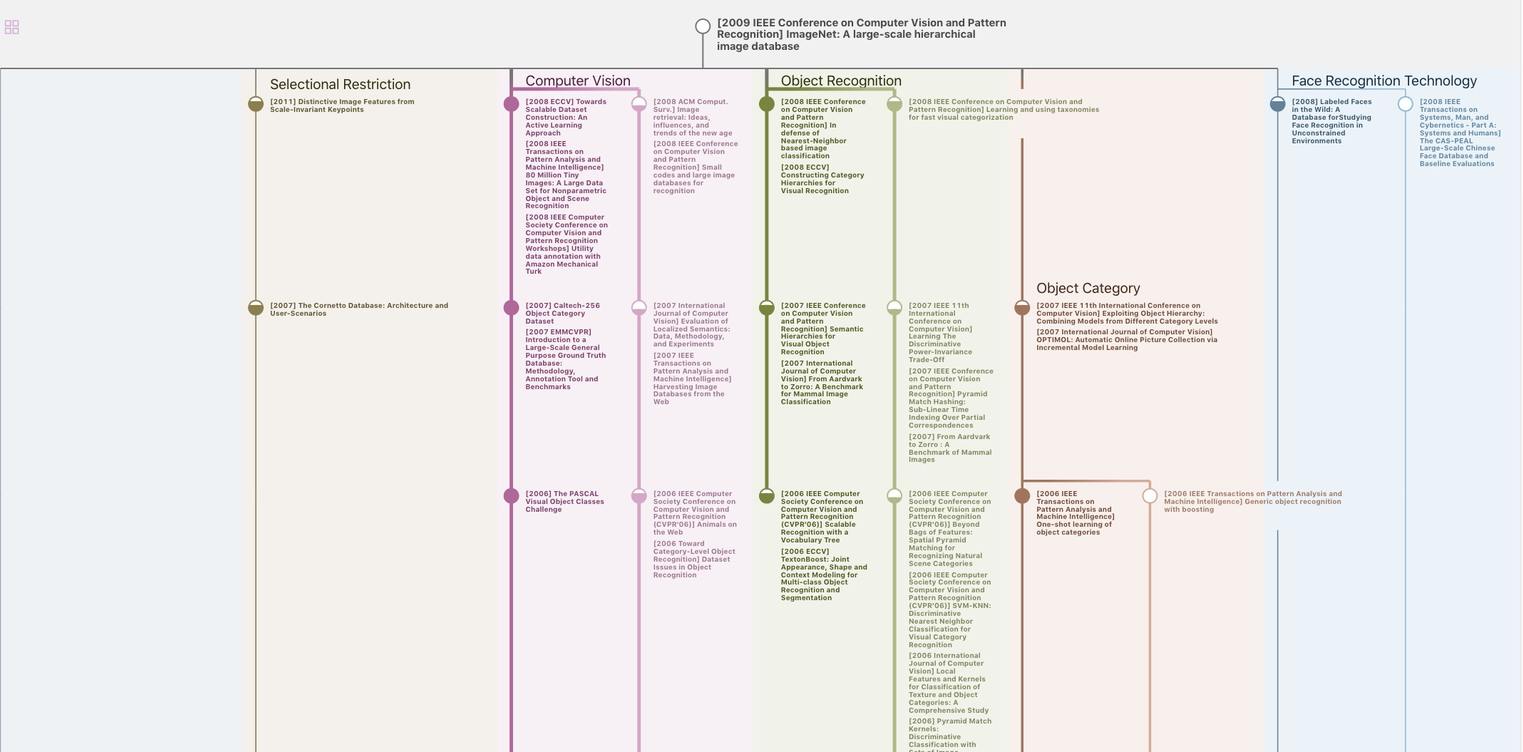
生成溯源树,研究论文发展脉络
Chat Paper
正在生成论文摘要