Decomposition and Reorganization of Phonetic Information for Speaker Embedding Learning.
IEEE ACM Trans. Audio Speech Lang. Process.(2023)
摘要
Speech content is closely related to the stability of speaker embeddings in speaker verification tasks. In this paper, we propose a novel architecture based on self-constraint learning (SCL) and reconstruction task (RT) to remove the influence of phonetic information on speaker embedding generation. First, SCL is used to reduce the divergence of frame-level features, which can avoid ambiguity between the resulting embeddings of the two utterances being compared. Second, RT is used to further remove phonetic information in frame-level layers, focusing on speaker-discriminative feature transformation. In our experiments, the speaker embedding models were trained on the VoxCeleb2 dataset and evaluated on the VoxCeleb1, Librispeech, SITW and VoxMovies datasets. Experimental results on VoxCeleb1 show that the proposed DROP-TDNN system reduced the EER by 7.5%, compared to the state-of-the-art ECAPA-TDNN system. Furthermore, the proposed DROP-TDNN system also outperformed the ECAPA-TDNN system in the experiments on SITW, Librispeech and VoxMovies under cross-dataset conditions. In the experiments on SITW, the proposed system reduced the EER by 3.4% compared to the ECAPA-TDNN system. In the experiments on Librispeech, the proposed system demonstrated the advantage of removing phonetic information under the clean speech condition, with a significant reduction of 25.5% in EER compared to the ECAPA-TDNN system. In the experiments on VoxMovies, the proposed system reduced the EER by up to 7.9% compared to the ECAPA-TDNN system under different pronunciation and background conditions.
更多查看译文
关键词
phonetic information,speaker,learning,reorganization
AI 理解论文
溯源树
样例
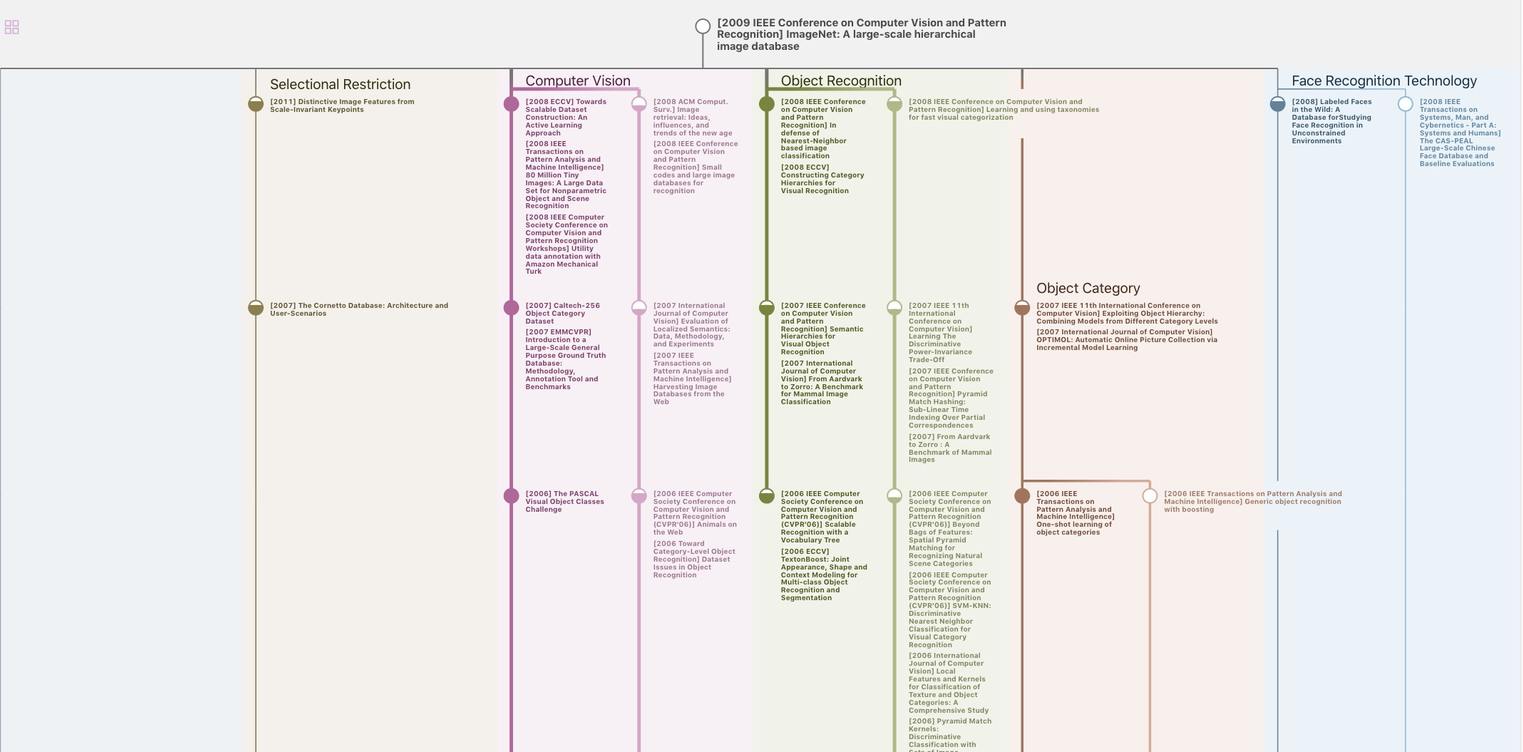
生成溯源树,研究论文发展脉络
Chat Paper
正在生成论文摘要