Regression to Classification: Ordinal Prediction of Calcified Vessels Using Customized ResNet50
IEEE Access(2023)
摘要
A substantial percentage of women die from cardiovascular disease (CVD). Computed tomography (CT) scan helps predict/monitor CVD-related diseases. However, previous studies found relation to assessing the risk of CVD by estimating severity of breast arterial calcification (BACs). In many countries, women undergo breast cancer screening using mammograms regularly, so it is easier to access vast datasets compared to CT-scan without additional cost and absorbing radiation. Earlier, measuring BACs severity was largely depends on the performance of segmentation of calcified vessel. Nevertheless, dataset maintenance for segmentation is expensive and tedious. As pixel-wise manual ground-truth (GT) marking can only be performed by multiple experienced experts. First time ever, to the best of our knowledge, a direct ordinal measurement of BACs severity is proposed without prior segmentation. Therefore, due to the complexity of calcified vessels, cascade classification performs better than end-to-end classification. In this proposed method, first, we imply Frangi filter to deduct background automatically without any prior ground-truth marking. Then, unlike other methods, interpolation is implied to shrink cropped images rather than splitting images. Also, left view images have flipped to reduce the complexity of different directions of breasts. In addition, among various residual network (ResNet) architecture, proposed method customized ResNet50 to adjust large image size and vessel complexity. The proposed method achieve, best AUC-score compare to state-of-art, above 90 and 85 percent for Normal vs Abnormal and Minor vs Severe patients, respectively. The proposed method reduces cost, time, and tedious job of researchers as this does not require segmenting calcified vessels and provides efficient prediction of BACs severity which is helpful to assess the risk of CVD in long run.
更多查看译文
关键词
Image segmentation,Residual neural networks,Deep learning,Breast cancer,Mammography,Complexity theory,Arteries,medical image,mammography,breast arterial calcification,coronary artery disease,cascade,ResNet
AI 理解论文
溯源树
样例
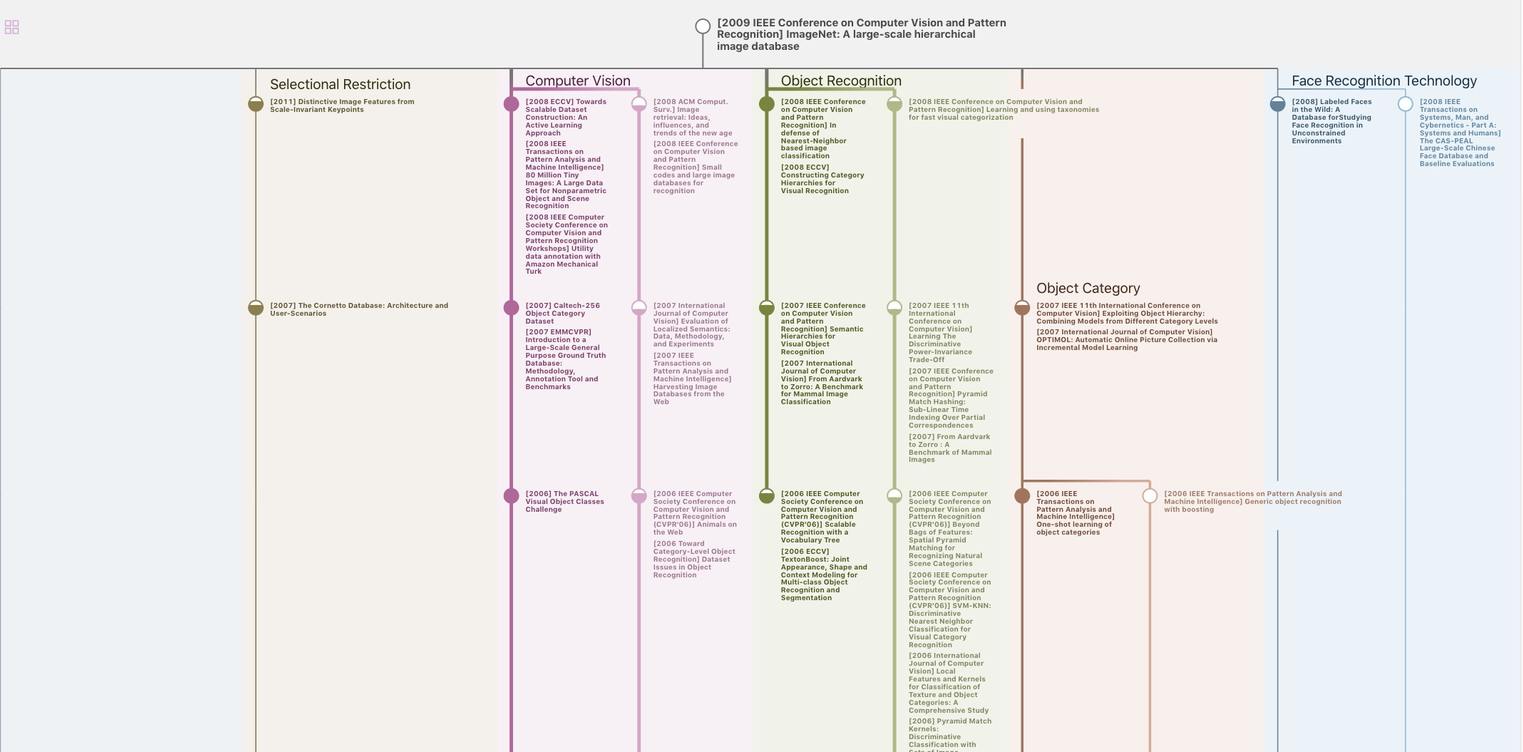
生成溯源树,研究论文发展脉络
Chat Paper
正在生成论文摘要