Occluded Scene Classification via Cascade Supervised Contrastive Learning.
IEEE J. Sel. Top. Appl. Earth Obs. Remote. Sens.(2023)
摘要
Occlusion handling is crucial for improving the performance of convolutional neural networks (CNNs) in real-world remote sensing images, which are often captured in complex and unconstrained environments. In particular, occlusion scene classification has received significant attention due to its usefulness in various remote sensing tasks. However, existing methods are limited by their dependence on close-world learning, which assumes that all test cases are included in the training set. This is problematic because occlusion is too complex to be thoroughly annotated. To address this issue, we propose a novel contrastive learning-based CNN that can classify out-of-distribution occluded scenes without the need for occlusion annotation. Our approach uses a two-branch subnetwork to learn representations of unoccluded anchor images and occlusion-augmented images. We then employ cascade supervised contrastive learning to make the network's representations invariant to occlusion. Unlike standard contrastive learning, our method leverages category information to avoid incompact intraclass distribution and uses a cascade strategy to hierarchically learn occlusion-invariant representations. Finally, we use a multilayer perceptron to classify the learned representations and assess representation quality. We evaluate our method on the UAVDT dataset and two simulated datasets, and the results demonstrate that our approach accurately characterizes occluded objects and achieves more precise classification in occlusion scenarios.
更多查看译文
关键词
Convolutional neural network (CNN),contrastive learning,occlusion images,remote sensing
AI 理解论文
溯源树
样例
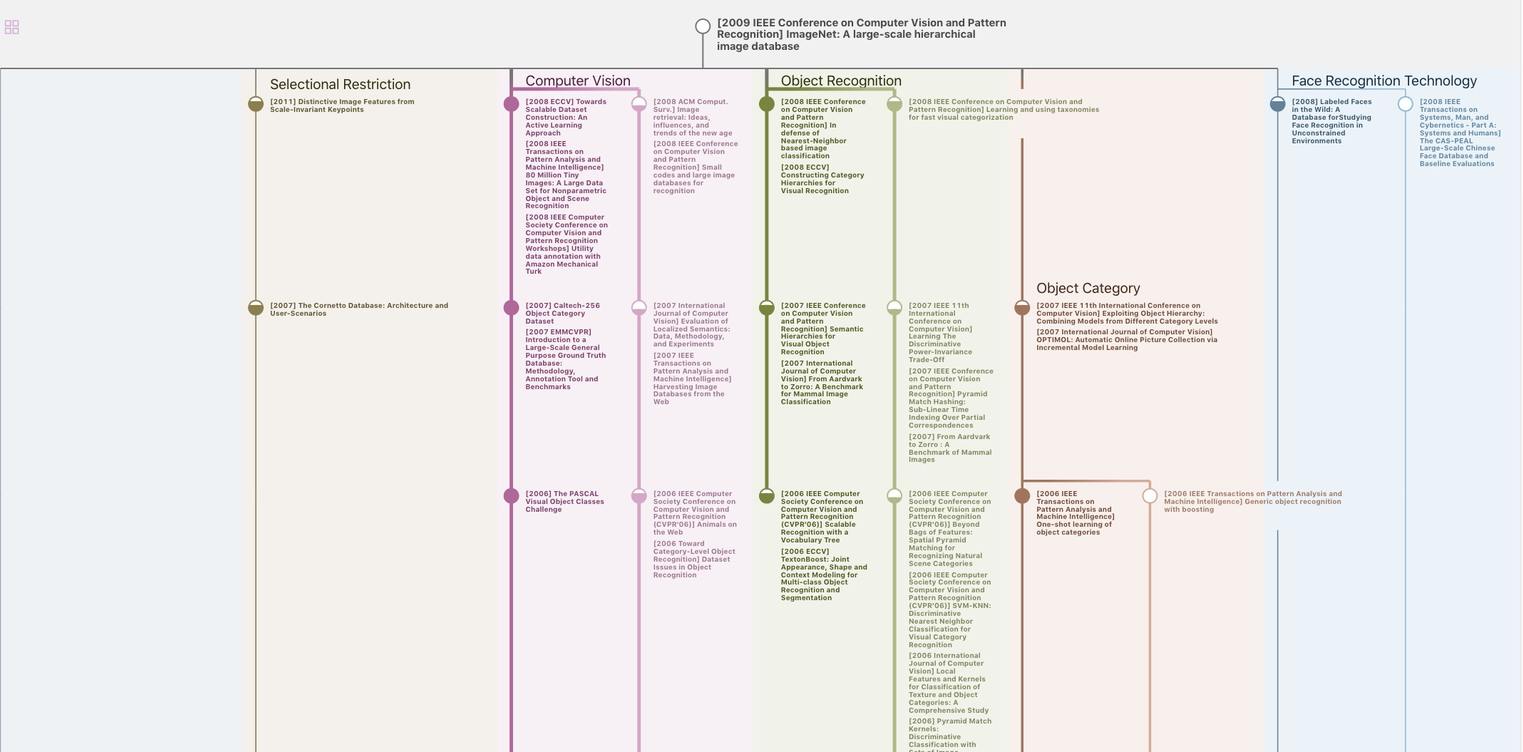
生成溯源树,研究论文发展脉络
Chat Paper
正在生成论文摘要