Learning relation-based features for fine-grained image retrieval
Pattern Recognit.(2023)
摘要
Fine-Grained Image Retrieval (FGIR) is a fundamental yet challenging task that has recently received considerable attention. However, two critical issues remain unresolved. On the one hand, convolutional neural networks (CNNs) trained with image-level labels tend to focus on the most discriminative im-age patches but overlook the implicit relation among them. On the other hand, existing large models developed for FGIR are computationally expensive and difficult to learn discriminative features. To ad-dress these issues without additional object-level annotations or localization sub-networks, we propose a novel unified framework for fine-grained image retrieval. Specifically, a novel Relation-based Convolu-tional Descriptor Aggregation (RCDA) method for extracting subtle yet discriminative features from fine-grained images is introduced. The RCDA method consists of a local feature generation network and a relation extraction (RE) module that models both explicit information and implicit relations. The explicit information is modeled by computing feature similarities, while the implicit relation is mined via an expectation-maximization algorithm. Moreover, we further leverage the knowledge distillation technique to optimize the parameters of the feature generation network and speed up the fine-tuning procedure by transferring knowledge from a large model to a smaller model. Experimental results on three benchmark datasets (CUB-200-2011, Stanford-Car and FGVC-Aircraft) demonstrate that the proposed method not only achieves a significant improvement over baseline models but also outperforms state-of-the-art methods by a large margin (6.4%, 1.3%, 23.2%, respectively).(c) 2023 Elsevier Ltd. All rights reserved.
更多查看译文
关键词
Fine-grained image retrieval,Implicit relation,Feature aggregation
AI 理解论文
溯源树
样例
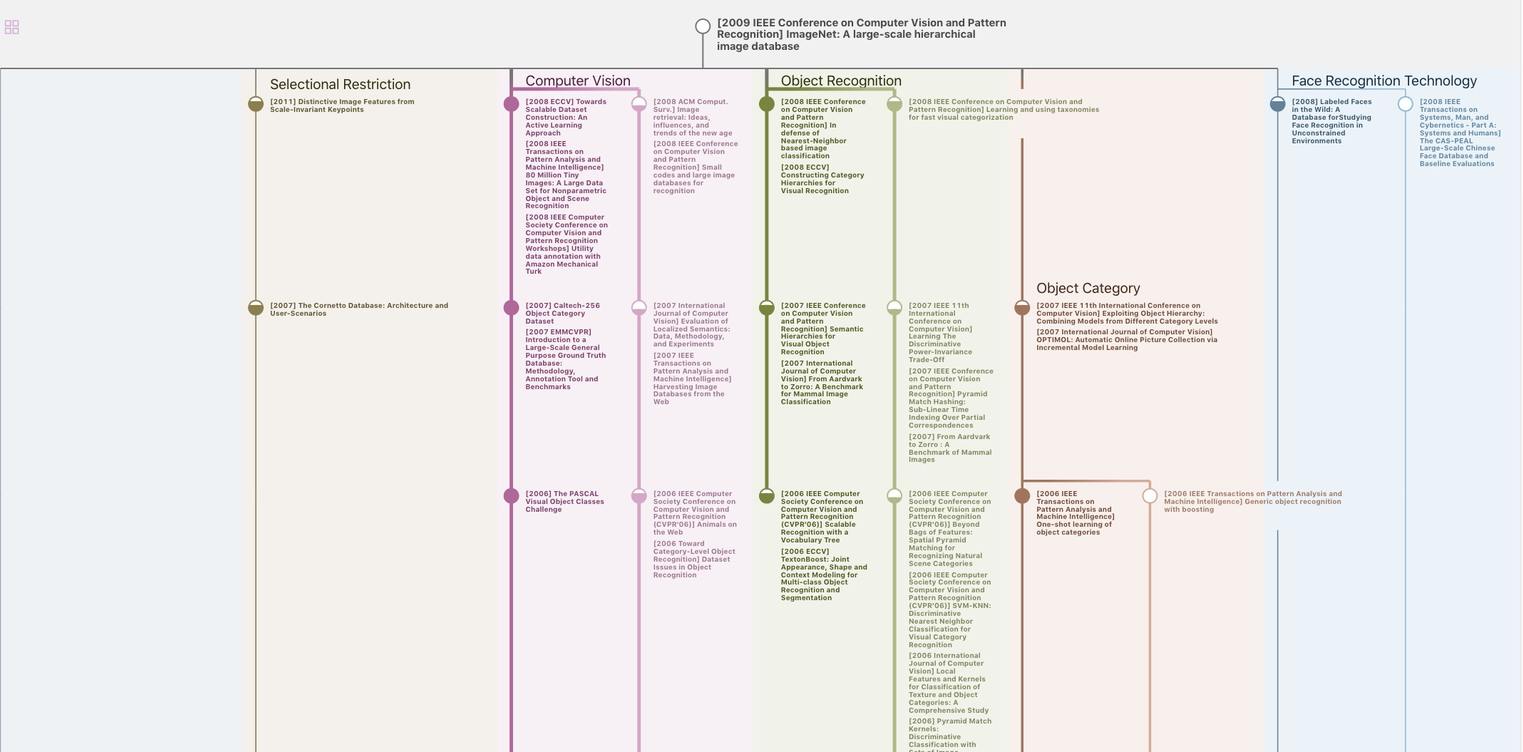
生成溯源树,研究论文发展脉络
Chat Paper
正在生成论文摘要