Gene expression data classification with robust sparse logistic regression using fused regularisation.
Int. J. Ad Hoc Ubiquitous Comput.(2023)
摘要
Microarray technology has become popular and is extensively used for gene classification. It is essential to identify a proper set of gene expressions that help to classify cancer data. However, microarray data comprises large number of genes with small set of samples. A penalised logistic regression (PLR) is good for variable selection in high dimensional microarray data. The techniques like Lasso, ridge and elastic net are suitable to reduce irrelevant features. However, they failed to produce properties like oracle property and sparsity resulted over fitting. To retain sparsity and oracle property, the weighted L1 and L2 penalties are used in logistic regression for gene expression data. In this paper, a new fused logistic regression (FLR) has been introduced using weighted L1 and L2 penalties for better gene selection. Regression algorithms were tested over the simulated as well as the real gene data sets.
更多查看译文
关键词
robust sparse logistic regression,fused regularisation,gene expression,classification
AI 理解论文
溯源树
样例
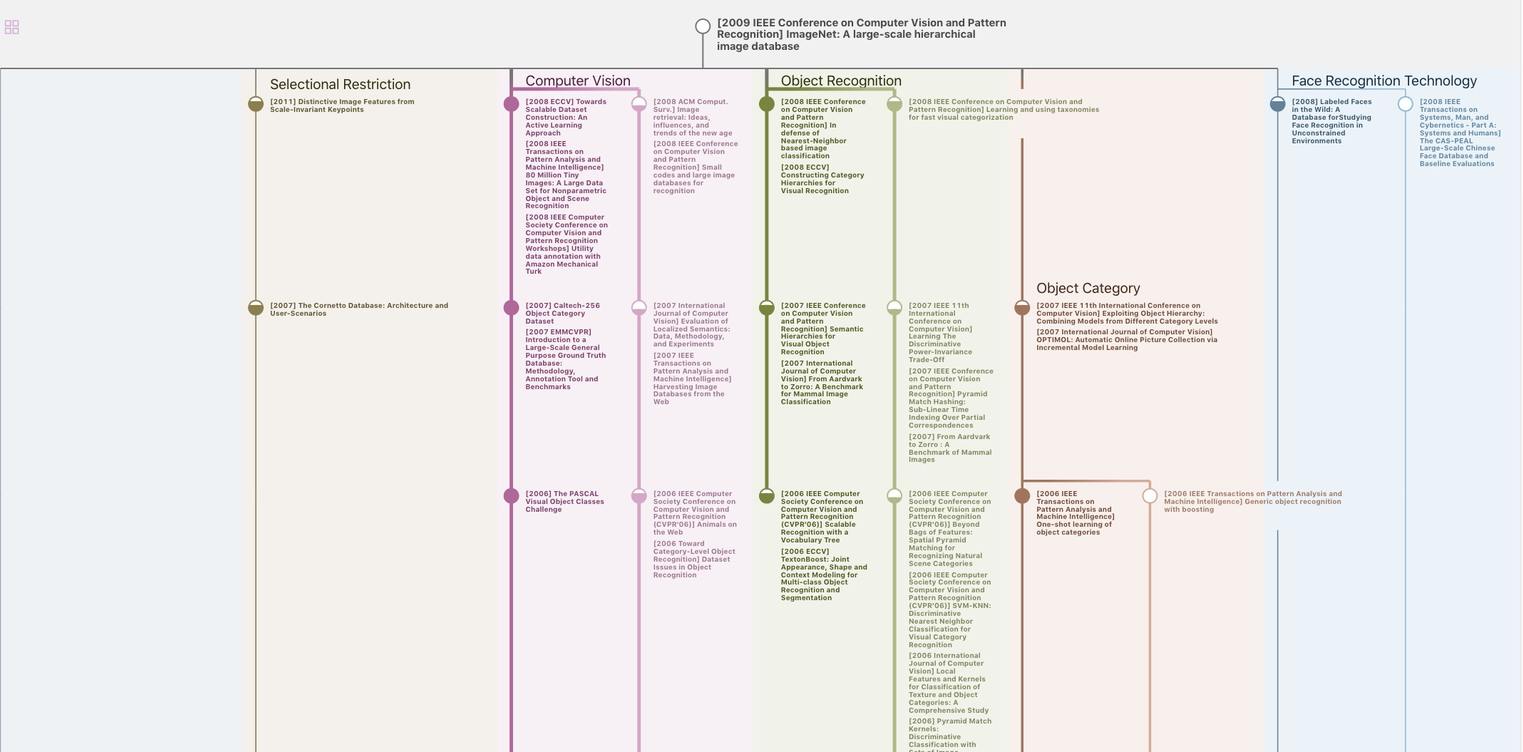
生成溯源树,研究论文发展脉络
Chat Paper
正在生成论文摘要