V2P-SSD: Single-Stage 3-D Object Detection With Voxel-to-Point Transformation
IEEE Geosci. Remote. Sens. Lett.(2023)
摘要
We study the problem of efficient object detection in 3-D point clouds with the voxel-point framework. Considering a large number of redundant and dense proposals are usually generated for small-sized objects during inference in voxel-based single-stage detectors, the existing detectors usually introduce extra subnetworks to filter and further refine the redundancy proposals. Albeit feasible, the computational and memory cost also increase during inference. In this letter, we introduce a novel voxel-to-point 3-D detector, termed V2P-SSD, which is a novel and lightweight pipeline that jointly integrates the voxel backbone and point head together in a single-stage framework. Different from dense predictions in feature maps, voxels related to objects in our framework are sampled with a fixed number and then transformed into points. Consequently, the point head is used to dynamically generate object proposals. Our voxel-to-point detection paradigm demonstrates a significant precision improvement on small-sized objects without introducing extra memory footprints. Extensive experiments conducted on KITTI and ONCE benchmarks validate the superiority of our method.
更多查看译文
关键词
Three-dimensional displays,Tensors,Point cloud compression,Proposals,Feature extraction,Semantics,Object detection,3-D object detection,LiDAR point clouds,voxel-to-point transformation
AI 理解论文
溯源树
样例
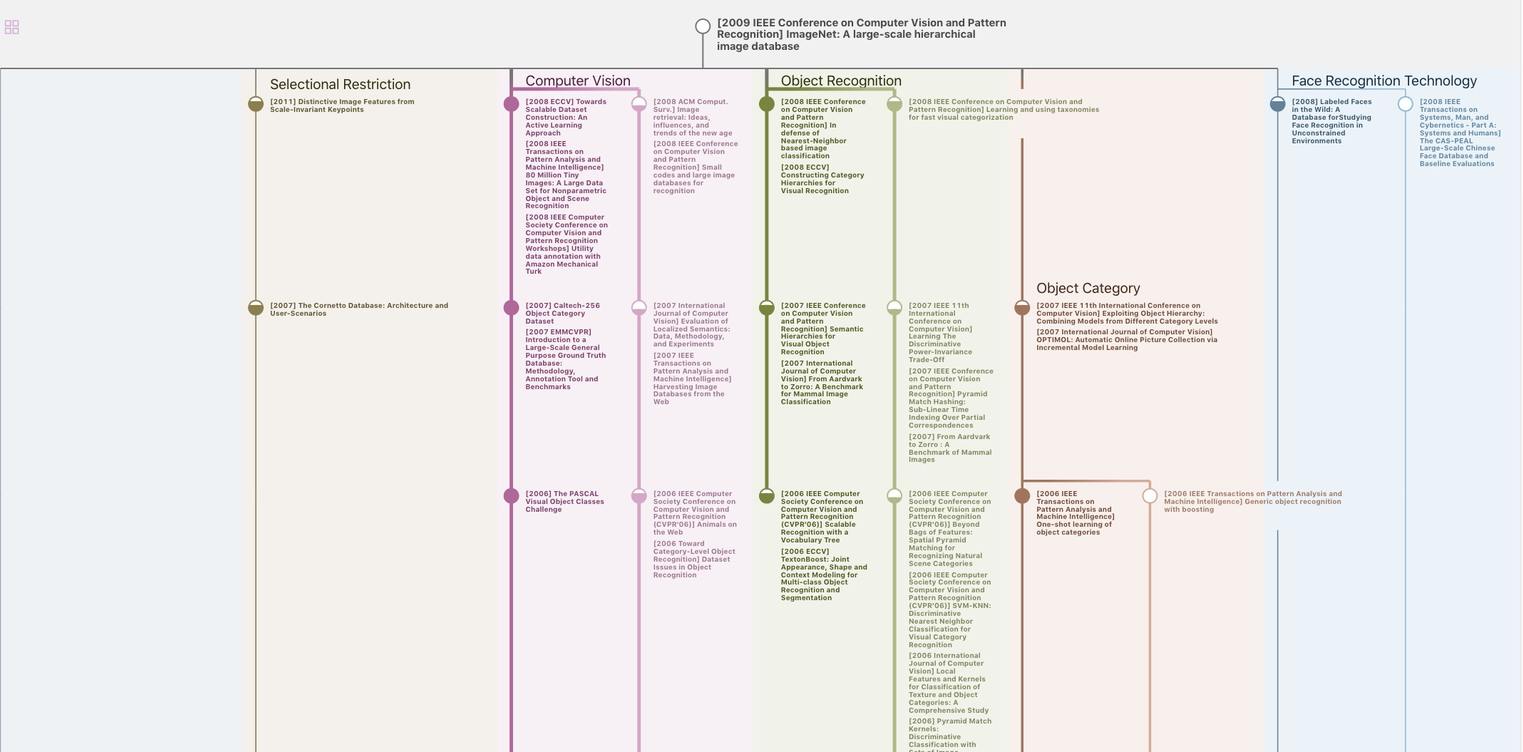
生成溯源树,研究论文发展脉络
Chat Paper
正在生成论文摘要