Approximate k-Nearest Neighbor Query over Spatial Data Federation.
DASFAA (1)(2023)
摘要
Approximate nearest neighbor query is a fundamental spatial query widely applied in many real-world applications. In the big data era, there is an increasing demand to scale these queries over a spatial data federation, which consists of multiple data owners, each holding a private, disjoint partition of the entire spatial dataset. However, it is non-trivial to enable approximate k -nearest neighbor query over a spatial data federation. This is because stringent security constraints are often imposed to protect the sensitive, privately owned data partitions, whereas naively extending prior secure query processing solutions leads to high inefficiency ( e.g. , 100 s per query). In this paper, we propose two novel algorithms for efficient and secure approximate k -nearest neighbor query over a spatial data federation. We theoretically analyze their communication cost and time complexity, and further prove their security guarantees and approximation bounds. Extensive experiments show that our algorithms outperform the state-of-the-art solutions with respect to the query efficiency and often yield a higher accuracy.
更多查看译文
关键词
spatial,federation,k-nearest
AI 理解论文
溯源树
样例
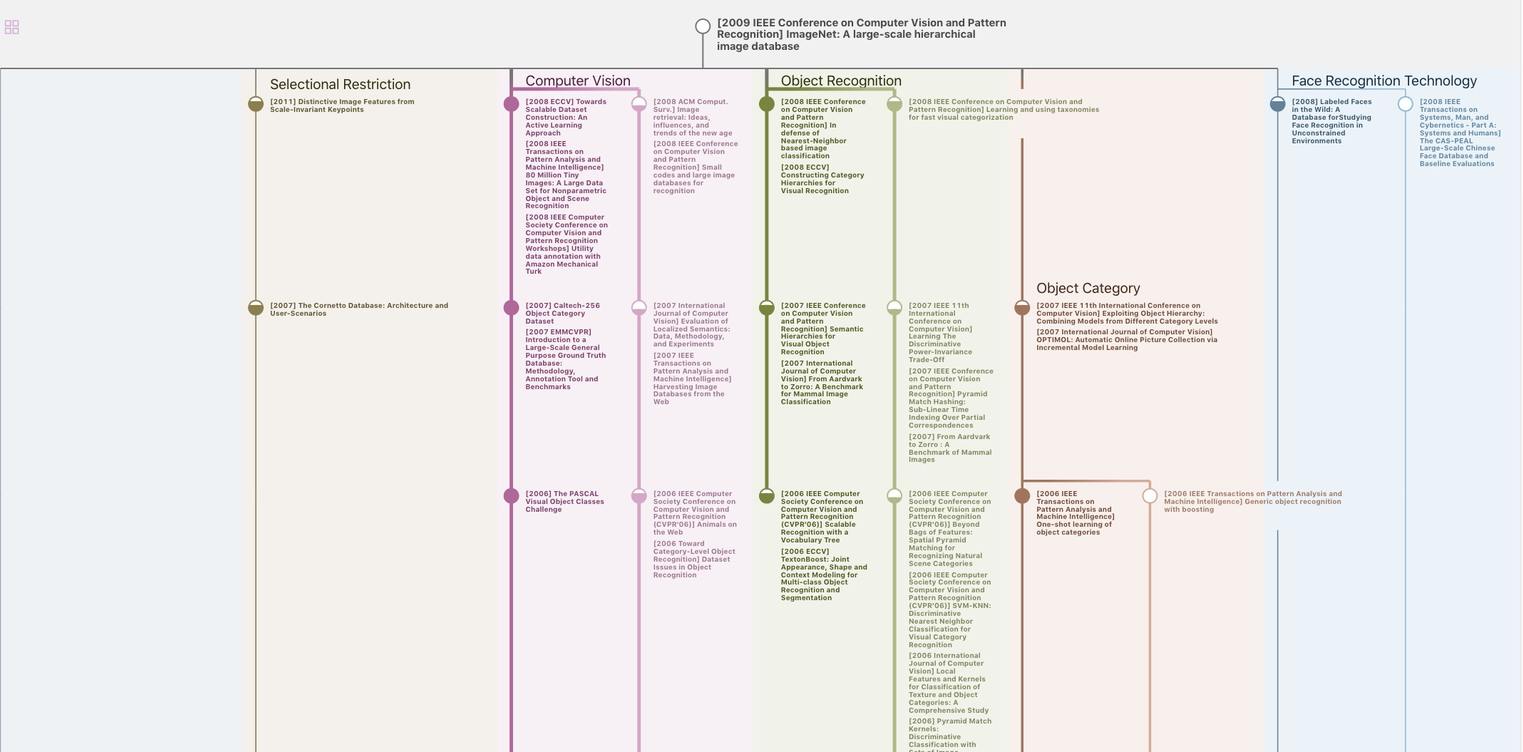
生成溯源树,研究论文发展脉络
Chat Paper
正在生成论文摘要