MG-CR: Factor Memory Network and Graph Neural Network Based Personalized Course Recommendation.
DASFAA (2)(2023)
摘要
Course recommendations in universities select the most suitable courses for students according to their interests and academic requirements. However, existing works often focus on modeling course selection history, without paying sufficient attention to rich features contained in students’ personal information and courses’ teaching attributes, and various relationships between them. In order to realize the personalization and dynamics of course recommendation, we consider students and courses as two types of nodes to construct a heterogeneous information network (HIN), and propose a factor Memory network and Graph neural network based personalized Course Recommendation (MG-CR) on top of HIN. MG-CR captures multiple features of students and courses simultaneously in the input module, thereby maximizing the retention of personalized information. The feature transfer module optimizes course features by considering various types of relationships between courses in the HIN, and together with the input module, it ensures high recommendation accuracy in the cold-start scenario. The updating module explicitly stores the student’s interest level for each factor in the state matrix, which is then used to dynamically recommend courses for students in the predicting module. Experimental results show that MG-CR provides accurate, dynamic and personalized recommendations, and has superior performance in the cold-start scenario.
更多查看译文
关键词
factor memory network,recommendation
AI 理解论文
溯源树
样例
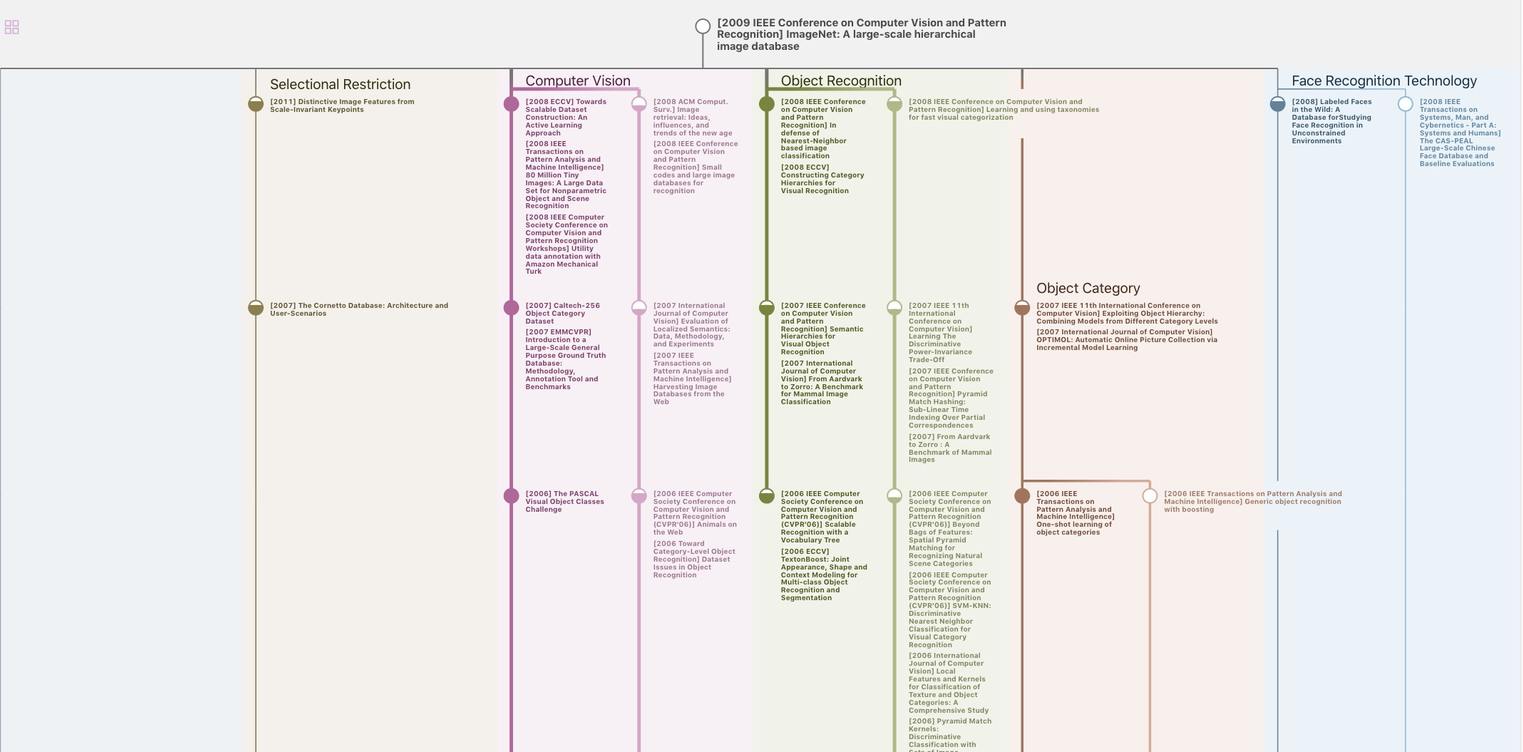
生成溯源树,研究论文发展脉络
Chat Paper
正在生成论文摘要