Causal Inference in Data Analysis with Applications to Fairness and Explanations.
Reasoning Web(2022)
摘要
Causal inference is a fundamental concept that goes beyond simple correlation and model-based prediction analysis, and is highly relevant in domains such as health, medicine, and the social sciences. Causal inference enables the estimation of the impact of an intervention or treatment on the world, making it critical for sound and robust policy making. However, randomized controlled experiments, which are typically considered as the gold standard for inferring causal conclusions, are often not feasible due to ethical, cost, or other constraints. Fortunately, there is a rich literature in Artificial Intelligence (AI), Machine Learning (ML), and Statistics on observational studies, which are methods for causal inference on observed or collected data under certain assumptions. In this paper, we provide an overview of popular formal and rigorous techniques for causal inference on observed data from the AI and Statistics literature. Furthermore, we discuss how concepts from causal inference can be used to infer fairness and enable explainability in machine learning models, which are critical in responsible data science when ML is used in making high-stake decisions in various contexts. Our discussion highlights the importance of using causal inference in ML models and provides insights on how to develop more transparent and responsible AI systems.
更多查看译文
关键词
fairness,explanations,data analysis
AI 理解论文
溯源树
样例
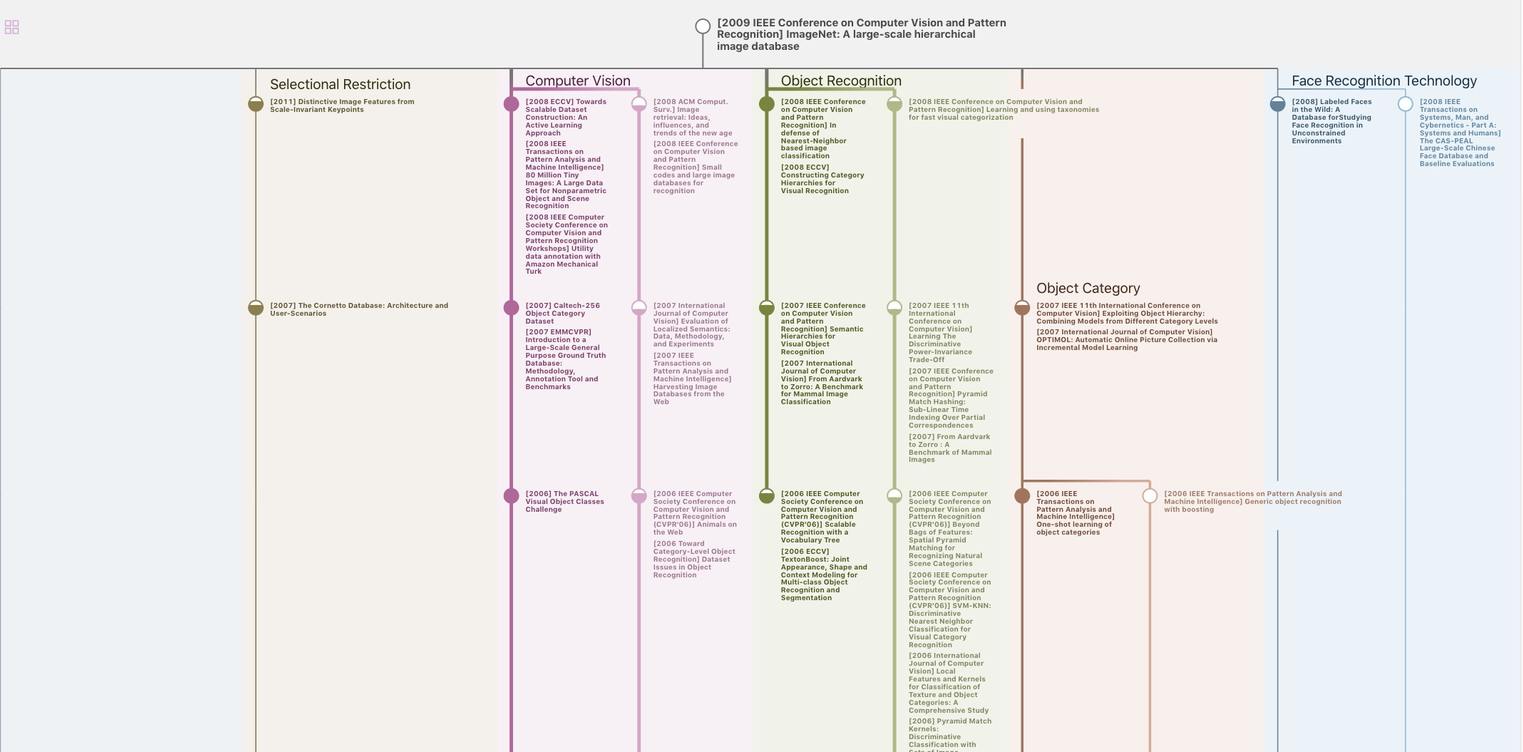
生成溯源树,研究论文发展脉络
Chat Paper
正在生成论文摘要