Robust multi-view non-negative matrix factorization with adaptive graph and diversity constraints.
Inf. Sci.(2023)
摘要
Multi-view clustering (MVC) has received extensive attention due to its efficient processing of high-dimensional data. Most of the existing multi-view clustering methods are based on non-negative matrix factorization (NMF), which can achieve dimensionality reduction and interpretable representation. However, there are following issues in the existing researches: (1) The existing methods based on NMF using Frobenius norm are sensitive to noises and outliers. (2) Many methods only use the information shared by multi-view data, while ignoring the diverse information between views. (3) The data graph constructed by the conventional K Nearest Neighbors (KNN) method may misclassify neighbors and degrade the clustering performance. To address the above problems, we propose a novel robust multi-view clustering method. Specifically, l(2,1)-norm is introduced to measure the factorization error to improve the robustness of NMF. Additionally, a diversity constraint is utilized to learn the diverse relationship of multi-view data, and an adaptive graph method via information entropy is designed to overcome the shortcomings of misclassifying neighbors. Finally, an iterative updating algorithm is developed to solve the optimization model, which can make the objective function monotonically non-increasing. The effectiveness of the proposed method is substantiated by comparing with eleven state-of-the-art methods on five real-world and four synthetic multi-view datasets for clustering tasks.
更多查看译文
关键词
Robust non-negative matrix factorization,Multi-view clustering,Diversity constraint,Adaptive graph constraint
AI 理解论文
溯源树
样例
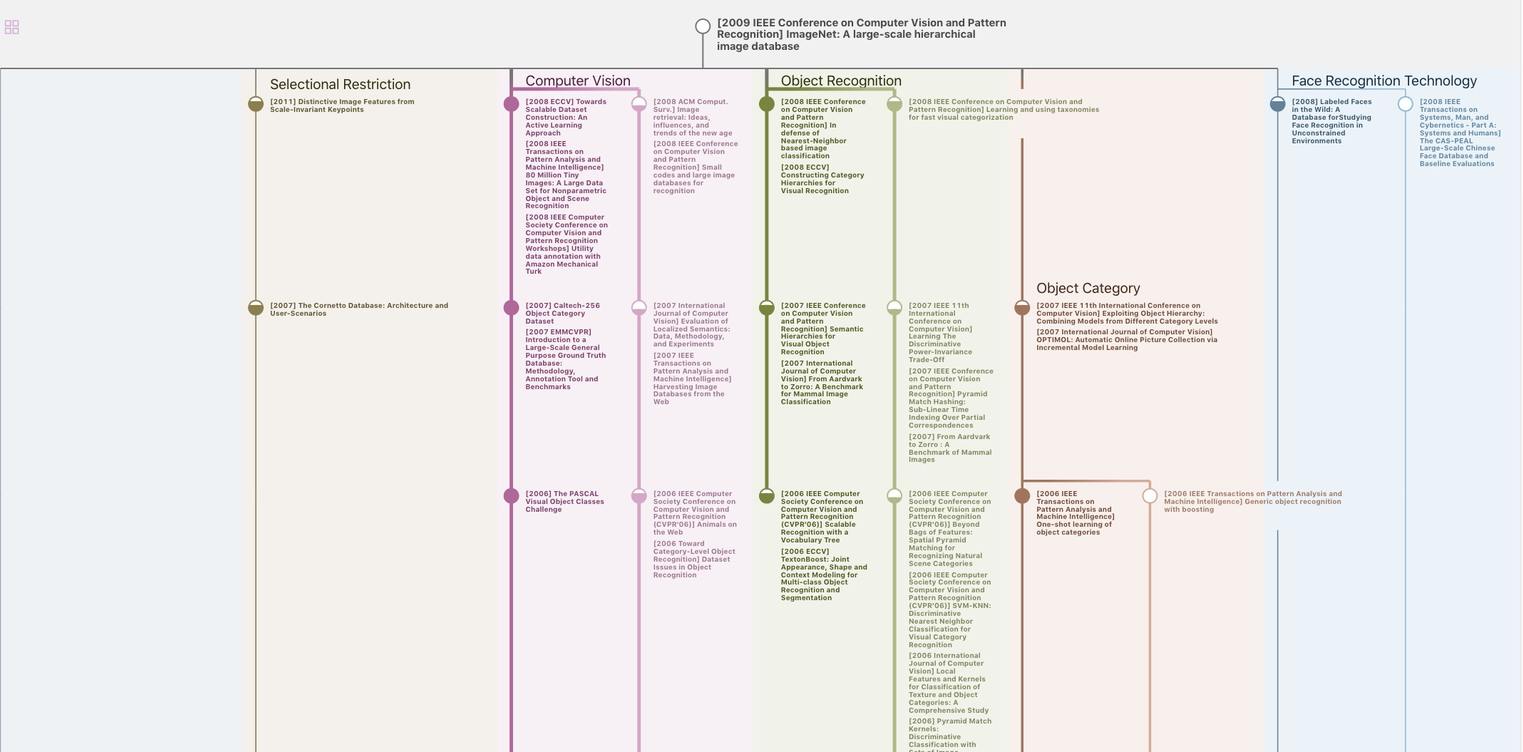
生成溯源树,研究论文发展脉络
Chat Paper
正在生成论文摘要