Structural redundancy reduction based efficient training for lightweight person re-identification
Inf. Sci.(2023)
摘要
Deploying person re-identification (Re-ID) models in edge devices is of great importance in the field of intelligent surveillance. It can not only relieve the computational pressure on cloud data centers, but also effectively protects data privacy (e.g., pedestrian identity). However, current Re-ID methods mostly rely on the large-scale backbone with huge parameters, making them impossible to deploy in edge devices. To solve this problem, light-weight Re-ID backbones came into being. The current proposed light-weight Re-ID backbones are all multi-branch parallel structures based on multi-scale ideas. Nonetheless, an excessive number of parallel branches tend to extract redundant information, which takes up too much storage resources and easily leads to overfitting of the model structure. These problems limit the performance of the current models. In this paper, we innovatively propose a blocks-by-blocks combine-and-expand (BB-CE) training method. First we construct a base architecture using the proposed single-branch multi -scale (SMS) module. Then, by combining and expanding the blocks in the base architecture stage by stage during training, BB-CE training method can reduce the redundancy of the structure, utilize the multi-scale information more efficiently, and thus improve the matching accuracy of re -identification. Extensive experimental results on four generic person Re-ID datasets demonstrate that the proposed method outperforms the state-of-the-art algorithms.
更多查看译文
关键词
Light-weight person re-identification,Combine-and-expand training,Structural re-parameterization,Multi-scale feature
AI 理解论文
溯源树
样例
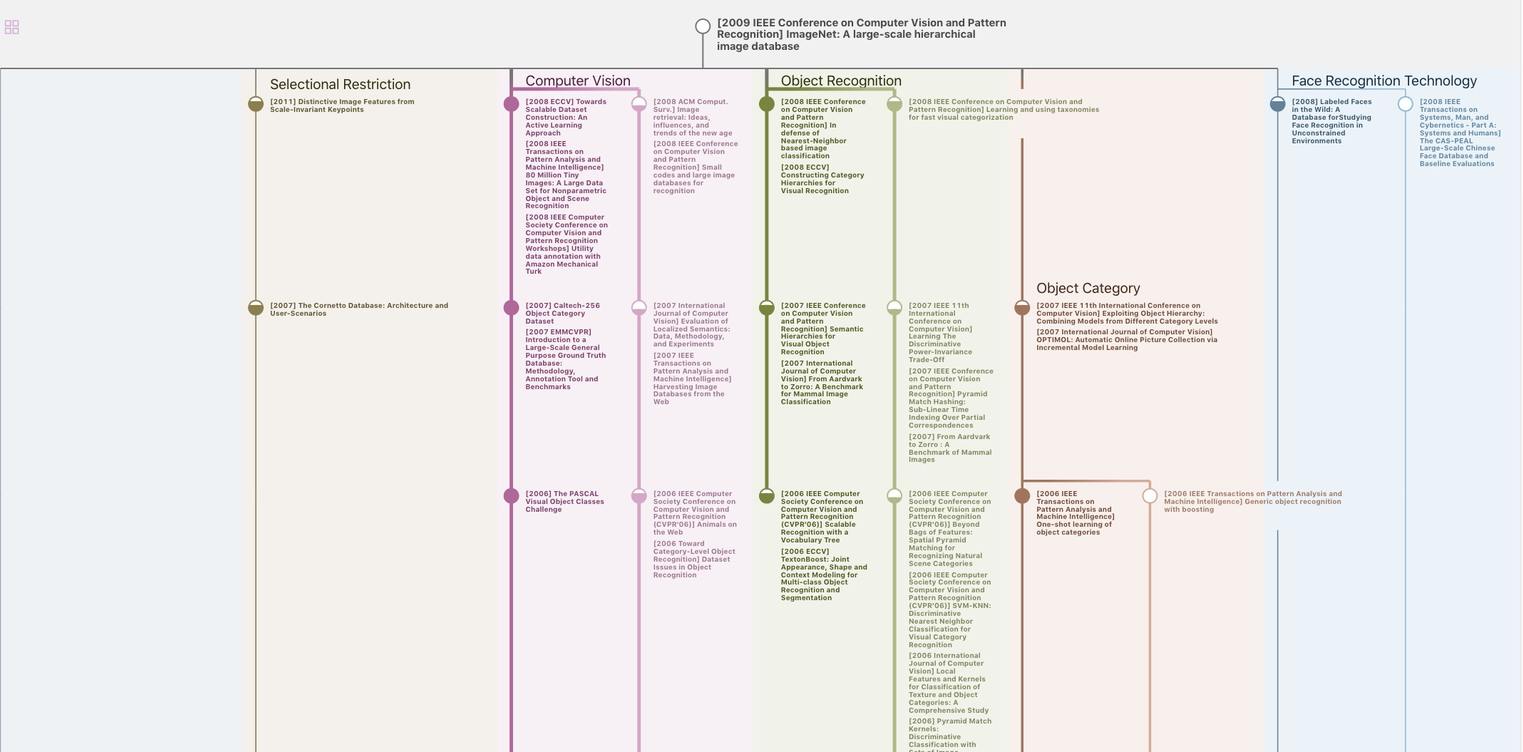
生成溯源树,研究论文发展脉络
Chat Paper
正在生成论文摘要