Deep Reinforcement Learning Based Resource Allocation with Heterogeneous QoS for Cellular V2X.
WCNC(2023)
摘要
Cellular vehicle-to-everything (C-V2X) communication is a crucial fundamental technology to serve diverse vehicular applications. However, the diversity of communications services in vehicular networks poses a challenge for designing an intelligent and efficient resource allocation framework. In this paper, a deep reinforcement learning (DRL)-based resource allocation framework is developed to meet the heterogeneous Quality of Service (QoS) requirements in heterogeneous vehicular communications networks, by training an agent to jointly optimize the sub-band and transmission power allocation. In particular, the resource allocation framework is formulated by considering the requirements of two key communication modes, the latency and reliability requirements of direct communication mode and the high-capacity requirements of cellular communication mode. Moreover, the interference of fast channel variations in high mobility vehicular environments is fully considered and modeled. Simulation results show that the proposed algorithm outperforms other baseline algorithms while achieving near-optimal performance.
更多查看译文
关键词
C-V2X,resource allocation,deep reinforcement learning,heterogeneous quality of service
AI 理解论文
溯源树
样例
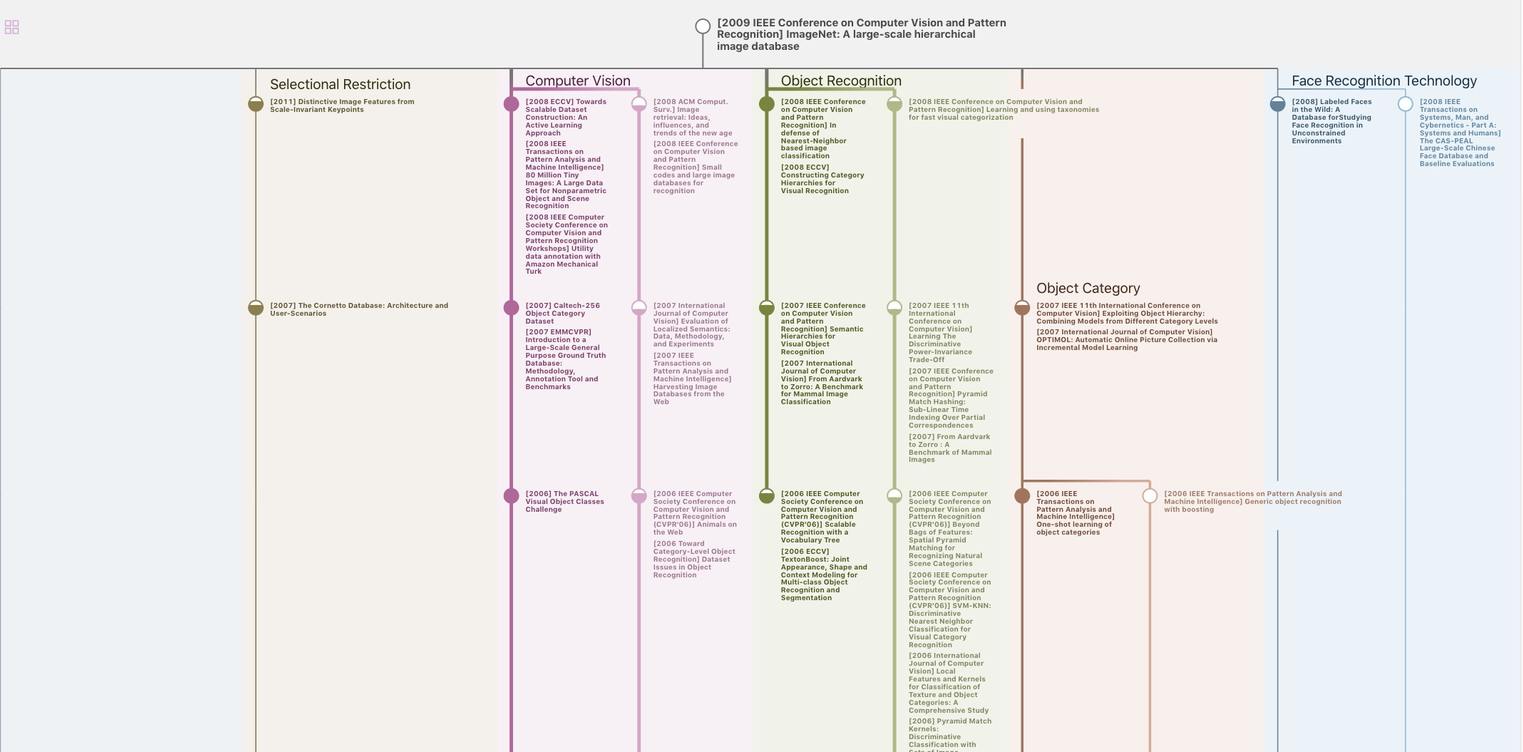
生成溯源树,研究论文发展脉络
Chat Paper
正在生成论文摘要