Deep Reinforcement Learning for Energy-Efficient Fresh Data Collection in Rechargeable UAV-assisted IoT Networks.
WCNC(2023)
摘要
The unmanned aerial vehicle (UAV) can act as the edge server in delay-sensitive monitoring for data collection and processing in the Internet of things (IoT) networks due to its flexibility and low operational cost. One of its major disadvantages is the limited battery level. This paper focuses on a problem with the rechargeable UAV-assisted energy-efficient and fresh data collection in the IoT networks. In particular, the UAV takes off from the initial position to collect data packets from sensor nodes (SNs) in the IoT networks and needs to reach the final position at a given time. Some charging stations (CSs) are in the IoT networks, which can recharge the UAV by the wireless power transfer technique to keep the UAV's energy level from falling below the threshold energy. To minimize the weighted sum of the average age of information (AoI) and the average recharging price, we design a Markov Decision Process (MDP) to determine the UAV's flight trajectory, the scheduling of SNs, and energy recharging. The MDP is then solved using a rechargeable UAV-assisted data collection algorithm based on dueling double deep Q-networks (D3QN). Numerous simulations show that the proposed D3QN algorithm can reduce the weighted sum of the average AoI and the average recharging price more effectively than the baseline algorithms.
更多查看译文
关键词
average recharging price,battery level,data packets,deep reinforcement,delay-sensitive monitoring,dueling double deep Q-networks,edge server,energy recharging,energy-efficient fresh data collection,initial position,low operational cost,Markov Decision Process,rechargeable UAV-assisted data collection algorithm,rechargeable UAV-assisted energy-efficient,rechargeable UAV-assisted IoT networks,things networks,threshold energy,UAV's energy level,UAV's flight trajectory,unmanned aerial vehicle,weighted sum,wireless power transfer technique
AI 理解论文
溯源树
样例
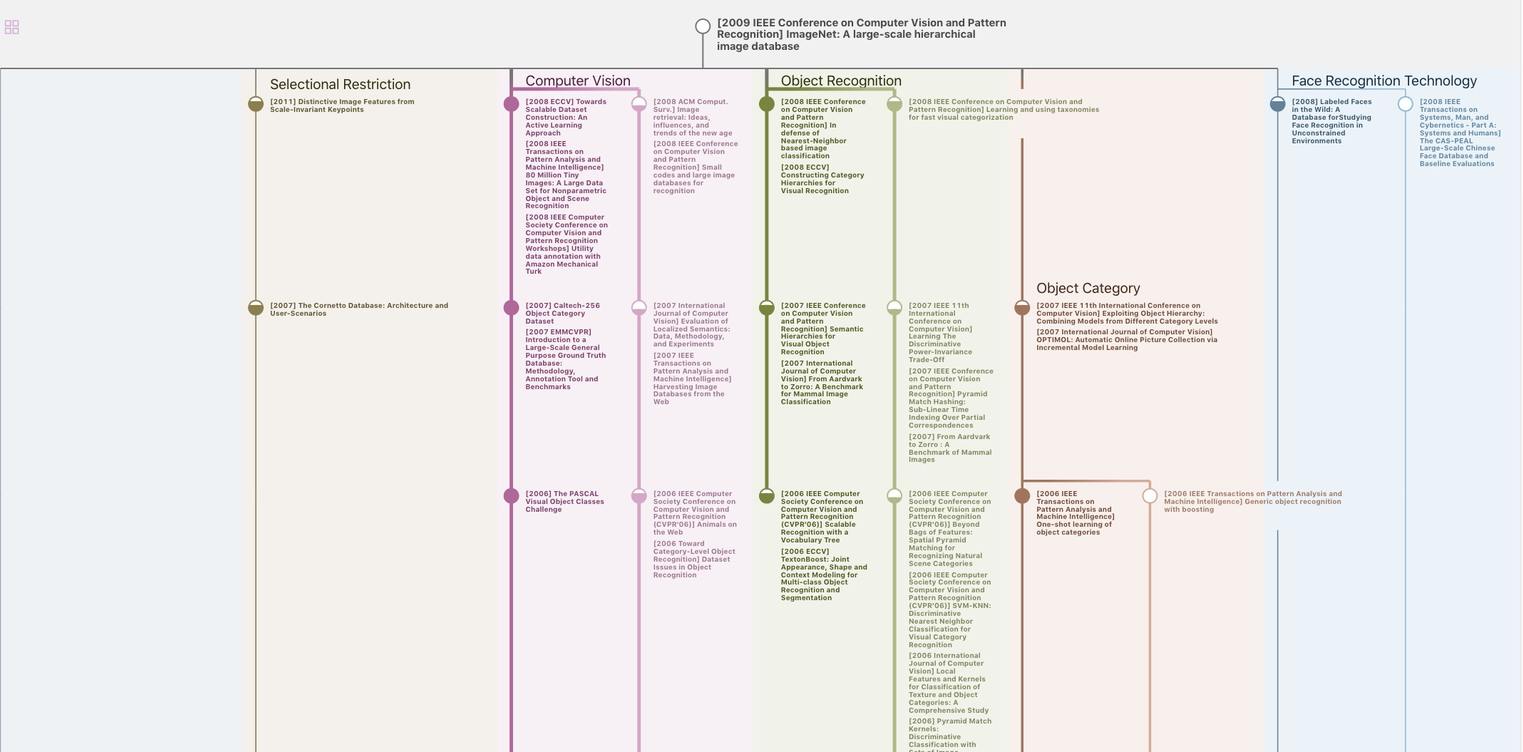
生成溯源树,研究论文发展脉络
Chat Paper
正在生成论文摘要