Masking-enabled Data Protection Approach for Accurate Split Learning.
WCNC(2023)
摘要
Split learning is an emerging distributed machine learning framework for enabling edge intelligence, especially for training sophisticated AI models at resource-constrained Internet-of-Things (IoT) devices. In split learning, the full AI model is partitioned to the client-side (e.g., input/privacy-sensitive layers) and server-side (e.g., computation-intensive layers) portions to be trained collaboratively at the devices and edge server. Only intermediate data at the split layer are exchanged during the training process for data privacy. However, the intermediate data may still cause security concerns to reconstruct the raw data from the partial gradients. This paper proposes the masking-enabled data protection approach for split learning without compromising the model accuracy. The devices are designed to perturb the reported results via masks, and the adversary can only retrieve the global information of all the devices (instead of individual devices). We mathematically prove that the masking-enabled perturbation mechanism would not compromise the learning accuracy. Experimental results validate the effectiveness of the proposed approach in terms of successful data protection and up to 10% model accuracy gain, compared to vanilla split learning and differential privacy.
更多查看译文
关键词
Split learning, edge intelligence, data privacy, double-masking perturbation
AI 理解论文
溯源树
样例
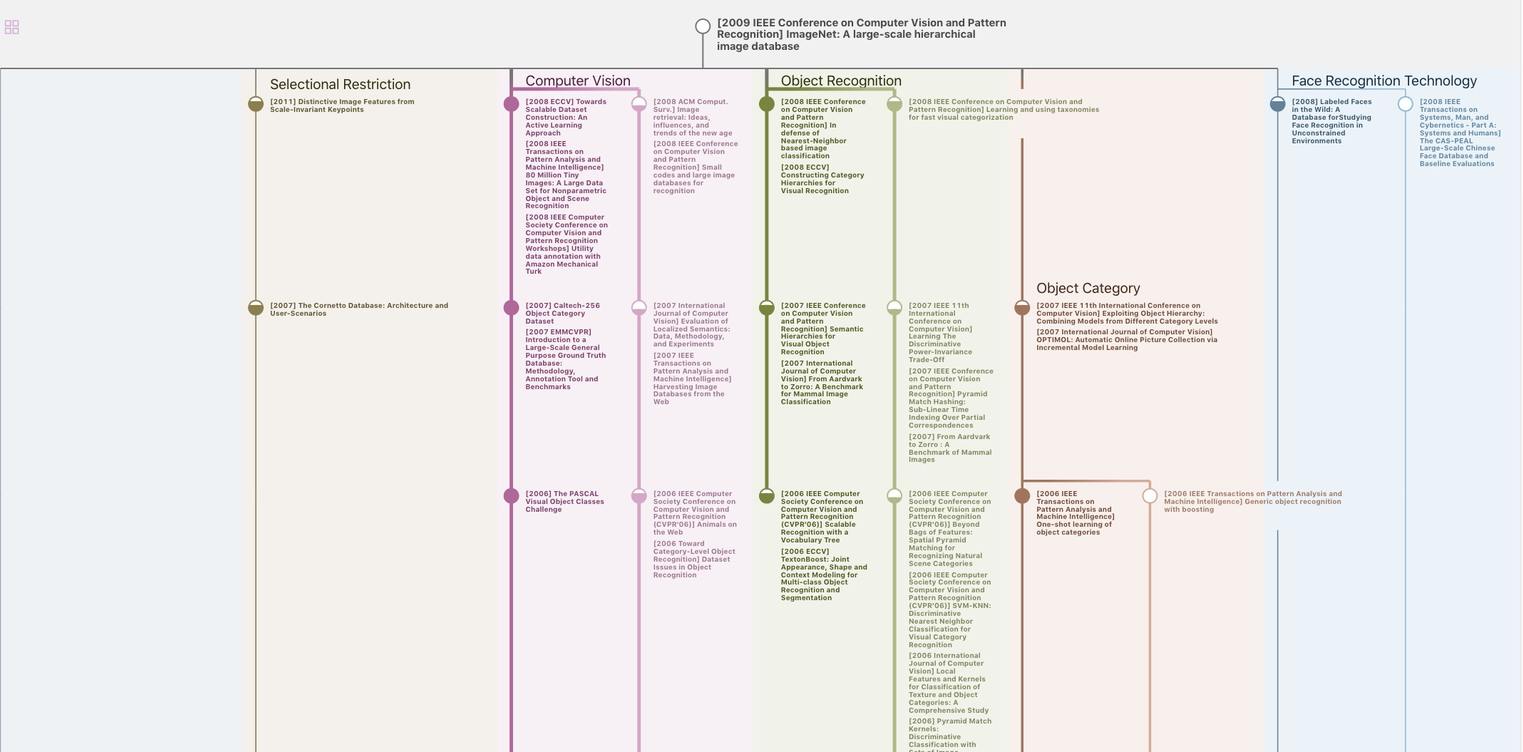
生成溯源树,研究论文发展脉络
Chat Paper
正在生成论文摘要