Subject Generalization in Classifying Imagined and Spoken Speech with MEG
NER(2023)
摘要
Speech decoding-based brain-computer interfaces (Speech-BCIs) decode speech directly from brain signals, which have the potential to offer faster and natural communication to patients with locked-in syndrome than the current BCI-spellers. On account of the huge cognitive variance among subjects, most of the current speech-BCI models have focused on subjectdependent decoding where the training and evaluation of the decoding algorithms use data from the same participants. These models do not generalize across individuals and, thus, are limited by the small data size that can be obtained from a single participant. Few studies have attempted subject-independent decoding but the performances are sub-par at best and significantly lower than subject-dependent models. To address this issue, we evaluated imagined and overt speech decoding with magnetoencephalography (MEG) recordings of eight speakers in a generalizable subject-independent setting. We used recent domain adaptation techniques including feature augmentation and curriculum learning to introduce generalizability to the decoding model. Our results indicated that domain adaptation techniques can be efficient in subject-independent decoding. The best performance was obtained with a curriculum learning based adaptation technique that resulted in decoding accuracy was close to that in subject-dependent decoding. Our findings show the possibility of subject generalization in neural speech decoding.
更多查看译文
关键词
curriculum learning,MEG,speech-BCI
AI 理解论文
溯源树
样例
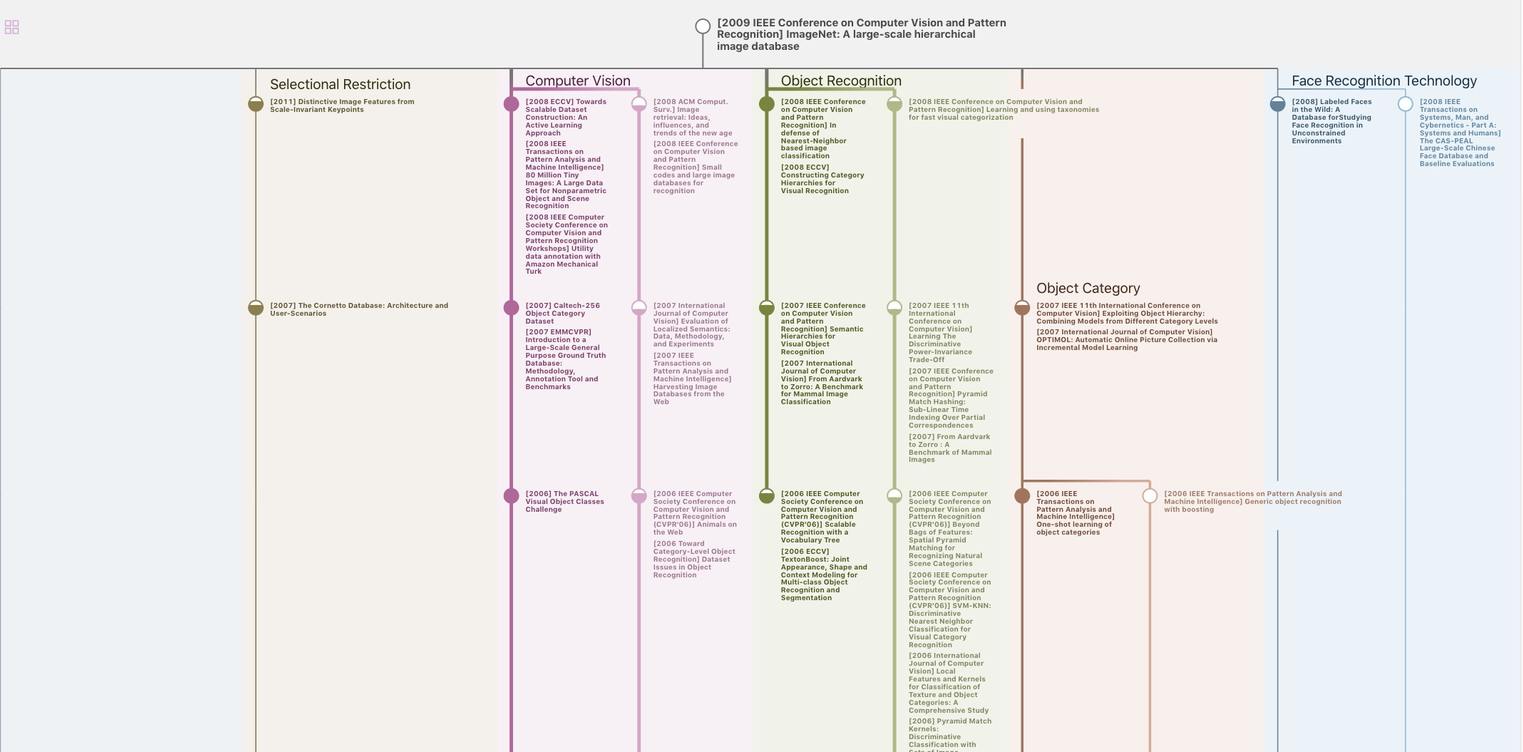
生成溯源树,研究论文发展脉络
Chat Paper
正在生成论文摘要