SR-HGN: Semantic- and Relation-Aware Heterogeneous Graph Neural Network.
Expert Syst. Appl.(2023)
摘要
Graph Neural Networks (GNNs) have received considerable attention in recent years due to their unique ability to model both topologies and semantics in the graphs. In this paper, we explore the use of GNNs in learning low-dimensional node representations in Heterogeneous Information Networks (HINs), which retain rich semantic information across multiple types of nodes and relations. Existing methods for HINs generally focus on modeling heterogeneity at either the node level or the relation level, but not both. As a result, these methods often fall short of optimal performance. To address this issue, we propose a novel Semantic -and Relation-aware Heterogeneous Graph neural Network, dubbed SR-HGN, which jointly incorporates rich semantics preserved on nodes and relations. Our approach involves projecting the HINs into a low-dimensional vector space through two steps: node-level aggregation and type-level aggregation. The node-level aggregation employs an attention mechanism to create relation vectors by aggregating messages from neighborhoods connected via the same type of relation. The type-level aggregation leverages relation vectors to aggregate node representations. In particular, we introduce semantic-aware attention and relation-aware attention in the type-level aggregation to model the contributions of relation vectors, in order to simultaneously gain knowledge from node semantics and relational information. Unlike other approaches that rely on pre-defined meta-paths, our model can be readily applied to most real-world applications without requiring any domain knowledge. To validate the effectiveness of our proposed approach, we conducted extensive experiments on three public datasets. Experimental results demonstrate that the SR-HGN significantly outperforms state-of-the-art baselines on node classification and node clustering tasks.
更多查看译文
关键词
graph,neural network,sr-hgn,relation-aware
AI 理解论文
溯源树
样例
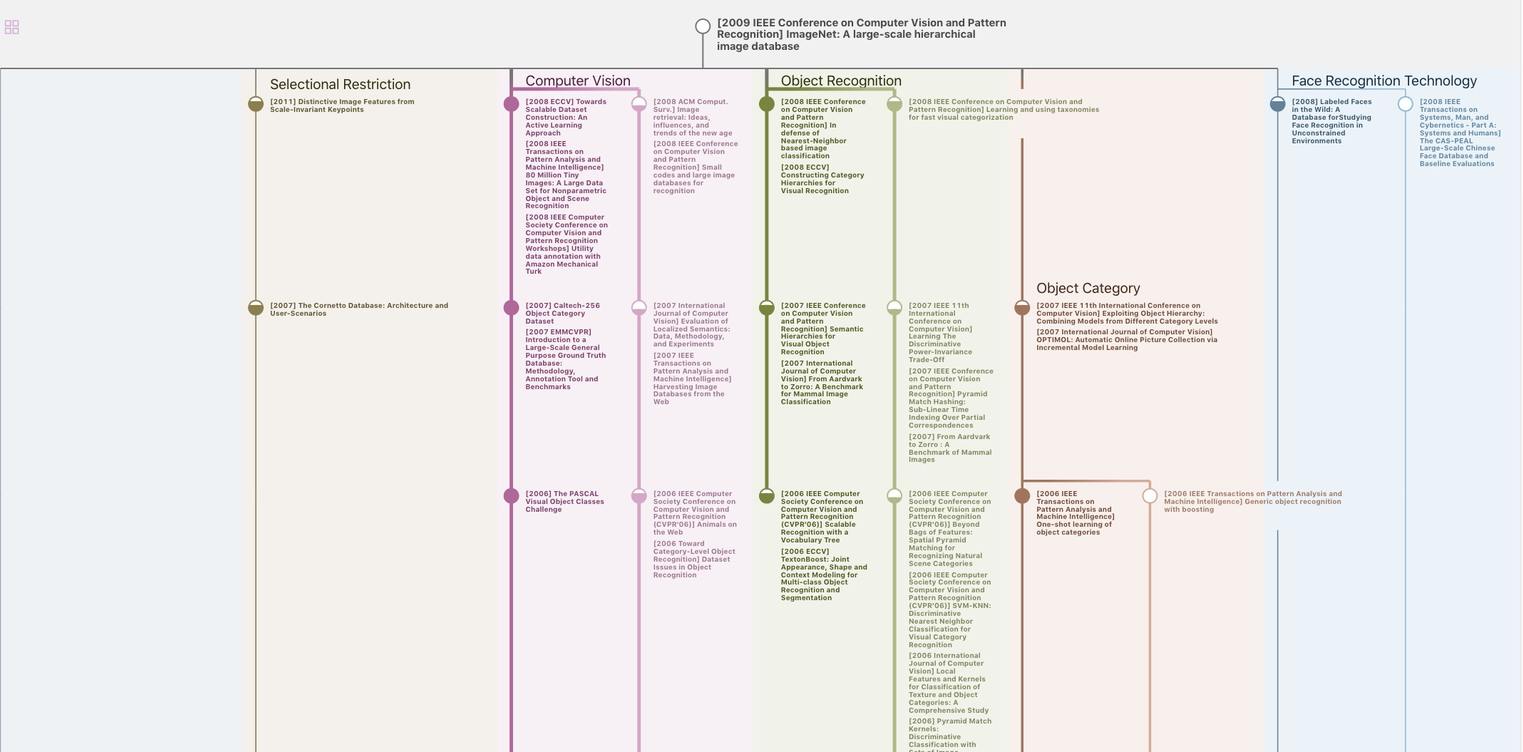
生成溯源树,研究论文发展脉络
Chat Paper
正在生成论文摘要