Assessing the influence of crop model structure on the performance of data assimilation for sugarcane.
Comput. Electron. Agric.(2023)
摘要
Process-base crop models (PBM) are important tools to describe how the agricultural system responds to envi-ronmental conditions. Sugarcane represents a major world source of sugar and ethanol and its PBMs had different levels of complexity in terms of structure, i.e. how detailed their processes were described. Yet, literature has widely demonstrated that data assimilation techniques (DA) represent a valuable option for reducing model uncertainty, but the inconsistency between PBM and the assimilated variable can significantly affect the per-formance of DA. Such limitation is strictly connected to model structure, and a hypothesis that arises from literature is that the use of more complex models would reduce model uncertainty after DA. We accessed the performance of using two different PBMs, one more detailed (DSSAT/SAMUCA, DS) and the other more general (WOFOST, WO), by assimilating leaf area index (LAI) retrieved from Landsat 7 ETM + and 8/OLI, using the Ensemble Kalman Filter (EnKF). Both PBMs were calibrated and evaluated with a robust database of 13 ex-periments and evaluated against a sugarmill database to evaluate the EnKF performance, compared with sim-ulations without DA (Open-loop, OP). Moreover, the processes involved in LAI simulations were analyzed to assess the EnKF performance. The DS had superior performance in the calibration and evaluation step with EF = 0.907, 0.878, 0.458 for stalk dry mass, stalk fresh yield (SFY), and LAI, while WO showed EF = 0.622, 0.610, 0.417 for the same variables, respectively. The calibration step affected the OP plot simulation, with DS having higher accuracy (RMSE = 31.678 Mg ha -1) and precision (R2 = 0.509), compared with WO (RMSE = 39.593 Mg ha -1; R2 = 0.458). However, after DA, both PBM presented error inconsistency with EnKF, despite the decrease in RMSE (-44.73% and-29.58%) and increase in R2 (22.15% and 36.50%) of DS and WO, respectively. The error inconsistency diverged from each PBM: the OP simulation of DS overestimated the Landsat LAI; after DA, simulated LAI decreased resulting in SFY underestimation (Bias =-11.469 Mg ha -1); WO showed OP simulations for LAI closer to Landsat's LAI values, despite the positive Bias in SFY estimation, and so EnKF slightly reduced the SFY overestimation (Bias = 22.944 Mg ha -1). Thus, the better descriptions of DS in terms of structure did not inhibit the error inconsistency. We suggested that new studies are required to understand how the assimilated variables impact on the other state variables of the PBM.
更多查看译文
关键词
Ensemble Kalman filter, DSSAT, SAMUCA, WOFOST, Landsat, Remote sensing, Leaf area index
AI 理解论文
溯源树
样例
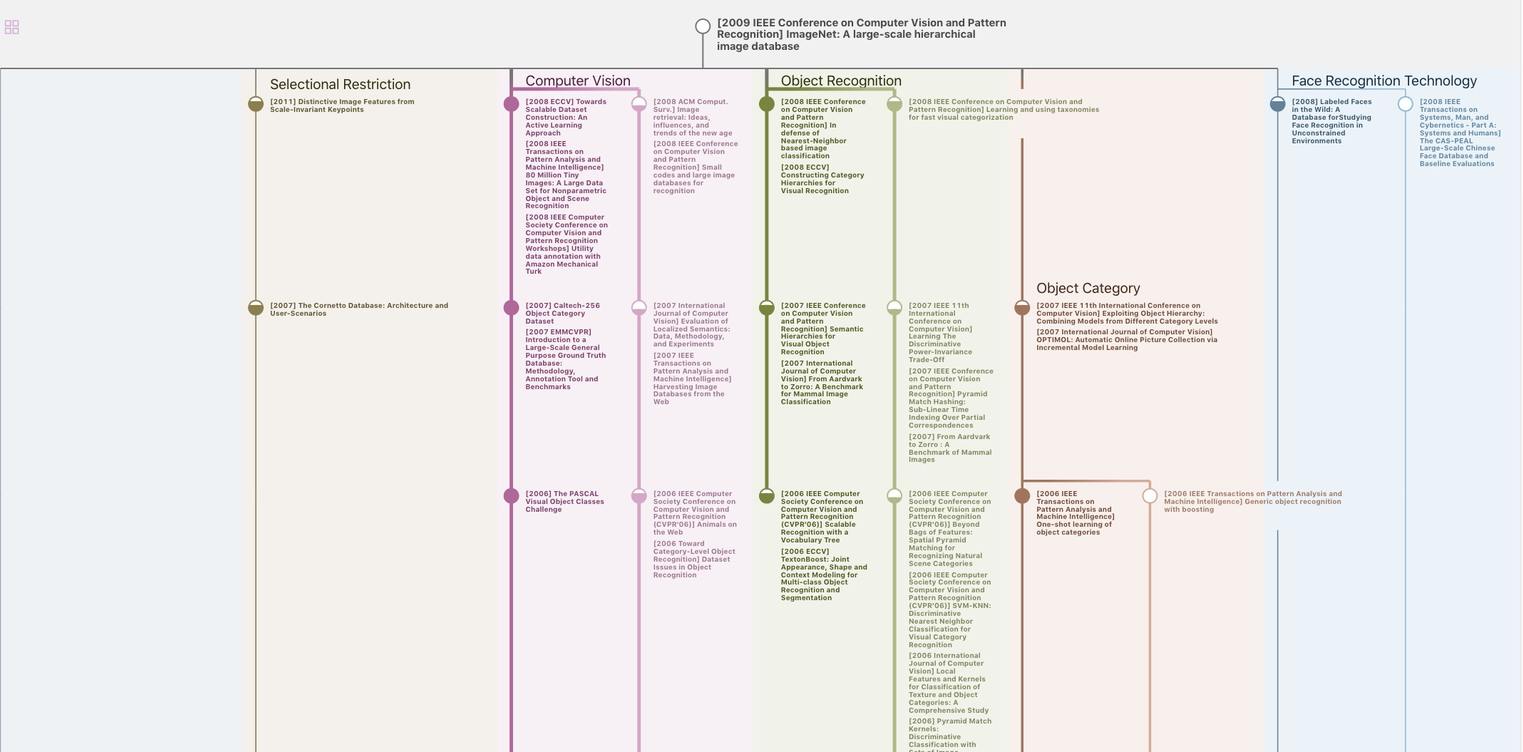
生成溯源树,研究论文发展脉络
Chat Paper
正在生成论文摘要