Two-stage framework for diabetic retinopathy diagnosis and disease stage screening with ensemble learning.
Expert Syst. Appl.(2023)
摘要
Diabetic retinopathy (DR), a consequence of diabetes, is among the most common causes of vision loss. Due to a lack of symptoms in the early stages, achieving a firm diagnosis is time-consuming and difficult, even for experts. Hence, a reliable machine learning (ML) model is essential in facilitating an early and timely diagnosis. Although several deep learning (DL) models have recently been developed for DR diagnosis, no real-world interpretation is available for the features considered for classification. Therefore, we propose a fully automatic, end-to-end, conventional ML framework to perform DR diagnosis and precise disease-stage screening. To the best of our knowledge, the proposed framework represents the first implementation of a two-stage framework for DR stage screening that is accurate, reliable, simple, and fast and in which the relevant features responsible for accurate diagnosis are identified in the first stage, and a reliable ML classification technique is identified from the second stage. Ensemble learning helps to achieve a robust ML model, even with a diverse and imbalanced database, which is essential in proving model applicability for real-world computer-aided diagnosis (CAD) applications. Indeed, our model is able to identify DR degrees: normal, mild, moderate, severe, and proliferative. The presented DR disease-stage identification model achieves classification accuracy, F1-score, and precision of 0.99 (95% confidence interval, 0.98–1) using a bagging ensemble learning technique, a specificity and sensitivity of 0.99 (95% confidence interval, 0.97–1), and an Area Under the ROC Curve (AUC) of 1.00 (95% confidence interval, 0.99–1).
更多查看译文
关键词
Diabetic retinopathy, Computer -aided diagnosis, Ensemble model, Machine learning, Classification, Deep learning
AI 理解论文
溯源树
样例
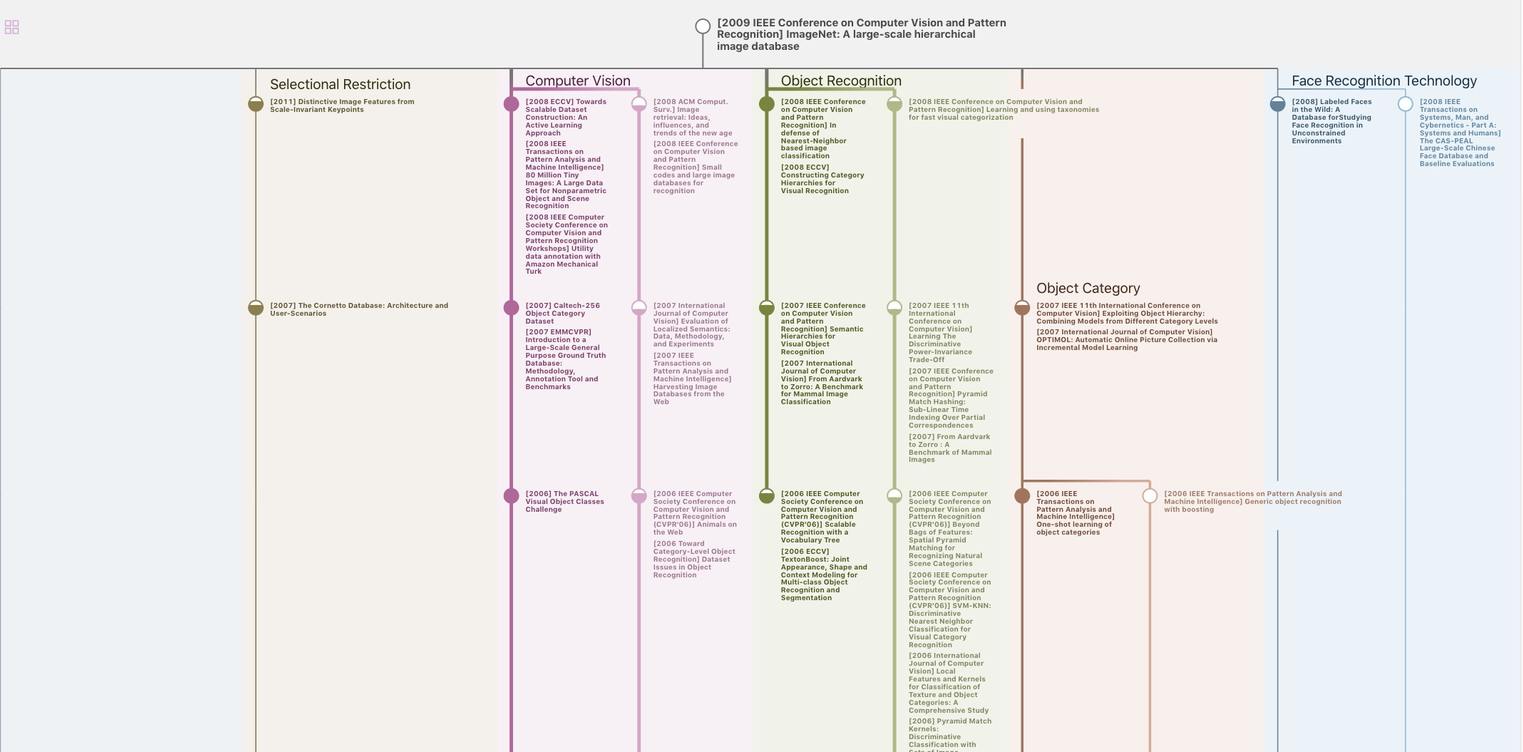
生成溯源树,研究论文发展脉络
Chat Paper
正在生成论文摘要