Large margin distribution multi-class supervised novelty detection.
Expert Syst. Appl.(2023)
摘要
As one of state-of-the-art supervised novelty detection models, support vector machine-supervised novelty detection (SVM-SND) can recognize whether a test instance is a novelty or which class the test instance comes from if it is a normal instance through a single model. The SVM-SND inherits the idea of maximizing minimum margin from one-class support vector machine. However, current research has proved that maximizing minimum margin is not the necessary condition to ensure the generalization in classification. This paper introduces margin distribution into multi-class supervised novelty detection and proposes a large margin distribution-supervised novelty detection (LMD-SND) model in which margin distribution is used to enhance the performance for multi-class supervised novelty detection. The margin distribution is represented by margin mean and margin variance. The LMD-SND maximizes the sum of margin means of all classes and minimizes the sum of margin variance of all classes through extra two terms, simultaneously. Compared with SVM-SND, LMD-SND inherits its merits for multi-class supervised novelty detection but has better generalization. The experimental results show that LMD-SND is superior to SVM-SND and can demonstrate comparative performance with shallow multi-class supervised novelty detection models, KNFST and KLMNN, and deep novelty detection models, DSVDD and DMSVDD.
更多查看译文
关键词
Novelty detection, Multi-class supervised novelty detection, Margin distribution, Margin theory, Large margin distribution-supervised novelty, detection
AI 理解论文
溯源树
样例
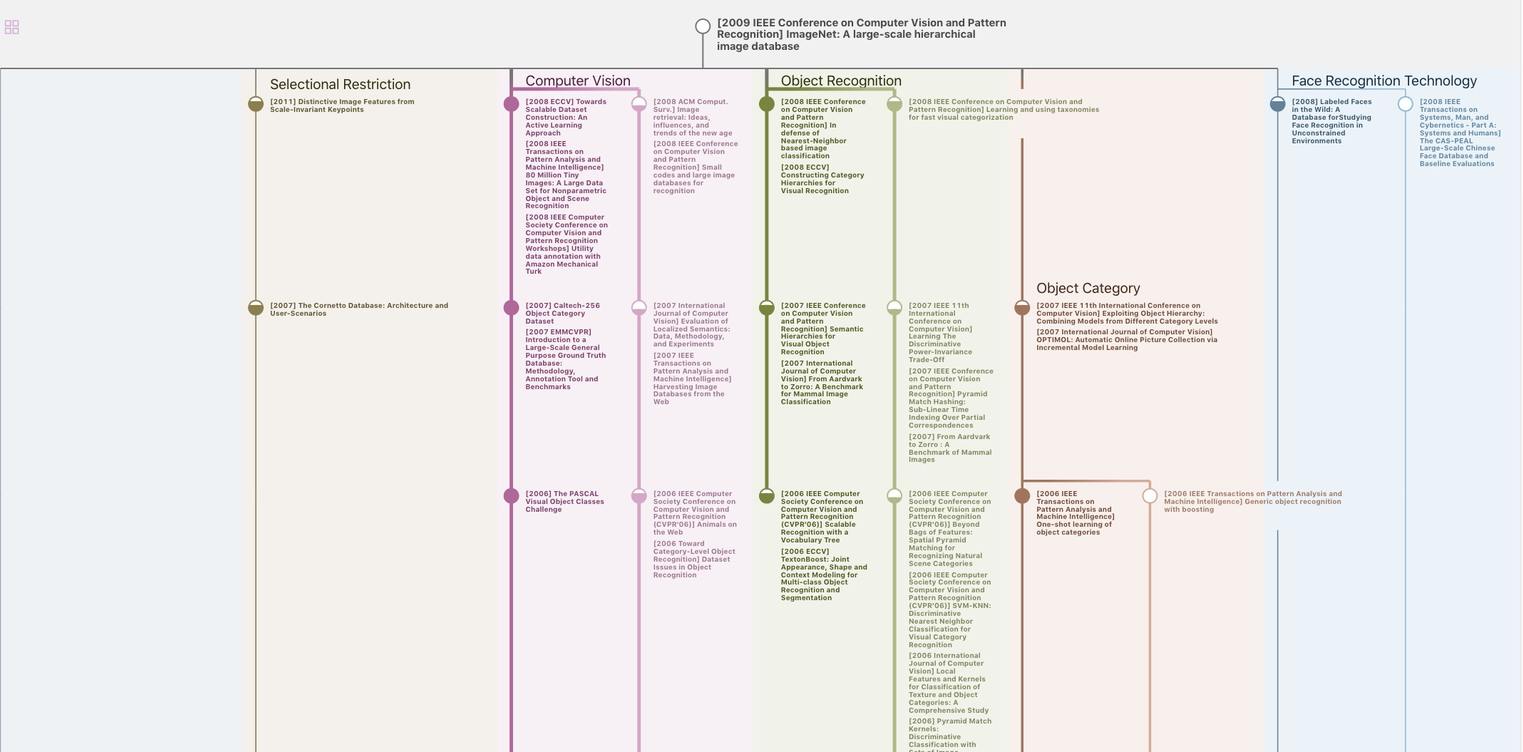
生成溯源树,研究论文发展脉络
Chat Paper
正在生成论文摘要