A Weighted Ensemble Classification Algorithm Based on Nearest Neighbors for Multi-Label Data Stream
ACM Trans. Knowl. Discov. Data(2023)
摘要
With the rapid development of data stream, multi-label algorithms for mining dynamic data become more and more important. At the same time, when data distribution changes, concept drift will occur, which will make the existing classification models lose effectiveness. Ensemble methods have been used for multi-label classification, but few methods consider both the accuracy and diversity of base classifiers. To address the above-mentioned problem, a Weighted Ensemble classification algorithm based on Nearest Neighbors for Multi-Label data stream (WENNML) is proposed. WENNML uses data blocks to train Active candidate Ensemble Classifiers (AEC) and Passive candidate Ensemble Classifiers (PEC). The base classifiers of AEC and PEC are dynamically updated using geometric and diversity weighting methods. When the difference value between the number of current instances and the number of warning instances reaches the passive warning value, the algorithm selects the optimal base classifiers from AEC and PEC according to the subset accuracy and hamming score and puts them into the predictive ensemble classifiers. Experiments are carried out on 12 kinds of datasets with 9 comparison algorithms. The results show that WENNML achieves the best average rankings among the four evaluation metrics.
更多查看译文
关键词
Multi-label,ensemble classification,data stream,dynamic update,concept,drift
AI 理解论文
溯源树
样例
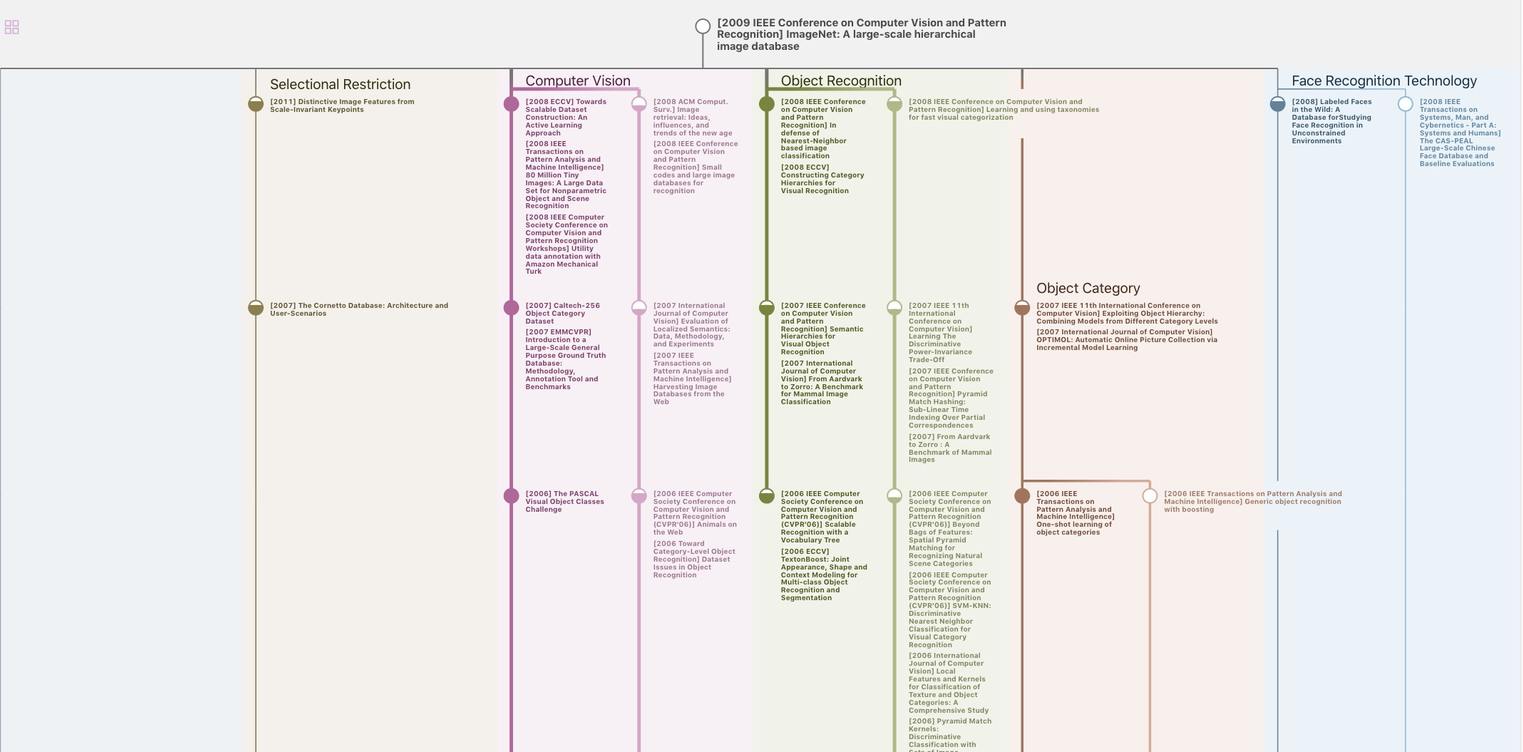
生成溯源树,研究论文发展脉络
Chat Paper
正在生成论文摘要