On the Limitations of Temperature Scaling for Distributions with Overlaps
arXiv (Cornell University)(2023)
摘要
Despite the impressive generalization capabilities of deep neural networks,
they have been repeatedly shown to be overconfident when they are wrong. Fixing
this issue is known as model calibration, and has consequently received much
attention in the form of modified training schemes and post-training
calibration procedures such as temperature scaling. While temperature scaling
is frequently used because of its simplicity, it is often outperformed by
modified training schemes. In this work, we identify a specific bottleneck for
the performance of temperature scaling. We show that for empirical risk
minimizers for a general set of distributions in which the supports of classes
have overlaps, the performance of temperature scaling degrades with the amount
of overlap between classes, and asymptotically becomes no better than random
when there are a large number of classes. On the other hand, we prove that
optimizing a modified form of the empirical risk induced by the Mixup data
augmentation technique can in fact lead to reasonably good calibration
performance, showing that training-time calibration may be necessary in some
situations. We also verify that our theoretical results reflect practice by
showing that Mixup significantly outperforms empirical risk minimization (with
respect to multiple calibration metrics) on image classification benchmarks
with class overlaps introduced in the form of label noise.
更多查看译文
关键词
calibration,temperature scaling,mixup,label noise
AI 理解论文
溯源树
样例
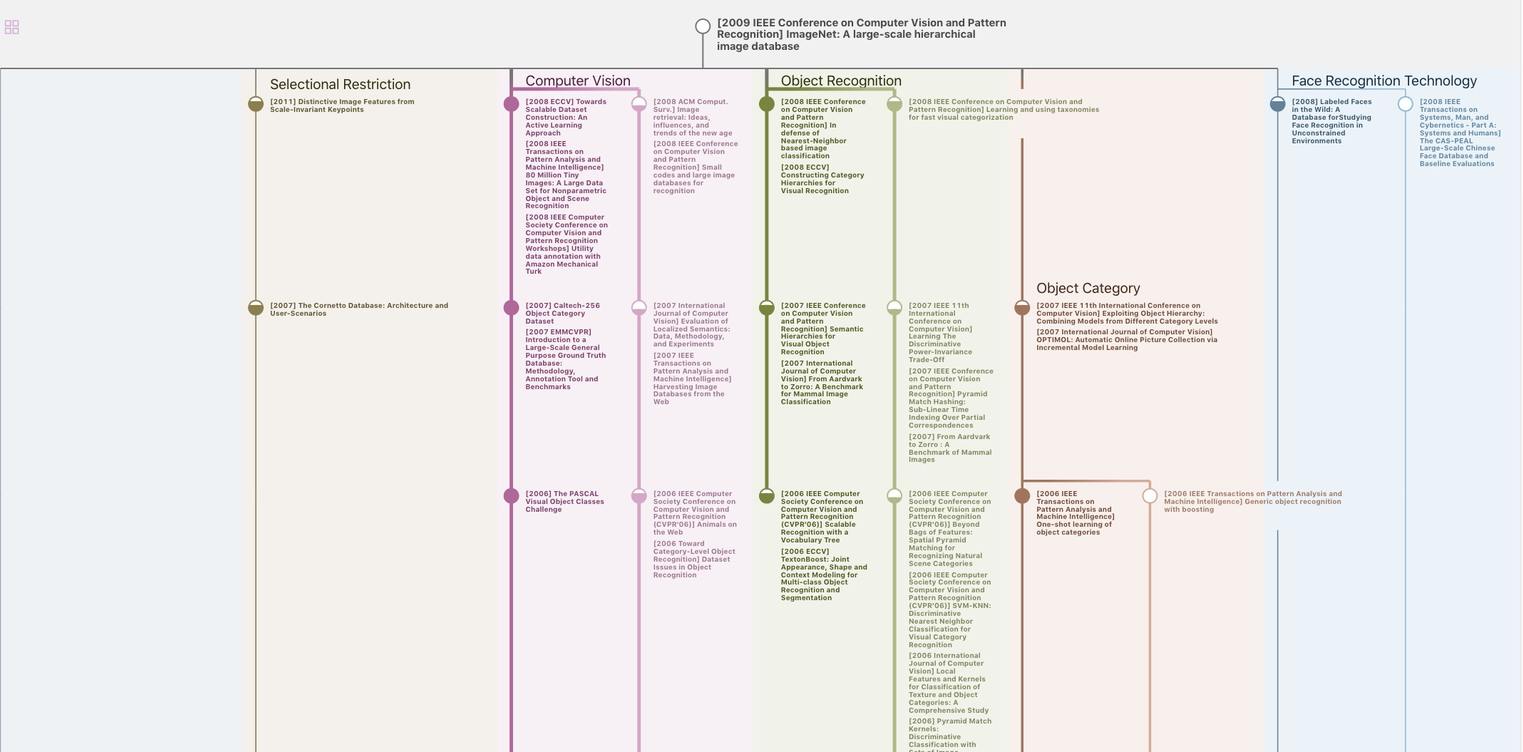
生成溯源树,研究论文发展脉络
Chat Paper
正在生成论文摘要