UNGOML: Automated Classification of unsafe Usages in Go
CoRR(2023)
摘要
The Go programming language offers strong protection from memory corruption. As an escape hatch of these protections, it provides the unsafe package. Previous studies identified that this unsafe package is frequently used in real-world code for several purposes, e.g., serialization or casting types. Due to the variety of these reasons, it may be possible to refactor specific usages to avoid potential vulnerabilities. However, the classification of unsafe usages is challenging and requires the context of the call and the program's structure. In this paper, we present the first automated classifier for unsafe usages in Go, UNGOML, to identify what is done with the unsafe package and why it is used. For UNGOML, we built four custom deep learning classifiers trained on a manually labeled data set. We represent Go code as enriched control-flow graphs (CFGs) and solve the label prediction task with one single-vertex and three context-aware classifiers. All three context-aware classifiers achieve a top-1 accuracy of more than 86% for both dimensions, WHAT and WHY. Furthermore, in a set-valued conformal prediction setting, we achieve accuracies of more than 93% with mean label set sizes of 2 for both dimensions. Thus, UNGOML can be used to efficiently filter unsafe usages for use cases such as refactoring or a security audit. UNGOML: https://github.com/stg-tud/ungoml Artifact: https://dx.doi.org/10.6084/m9.figshare.22293052
更多查看译文
关键词
graph neural networks,Go,unsafe package,classification,API-misuse
AI 理解论文
溯源树
样例
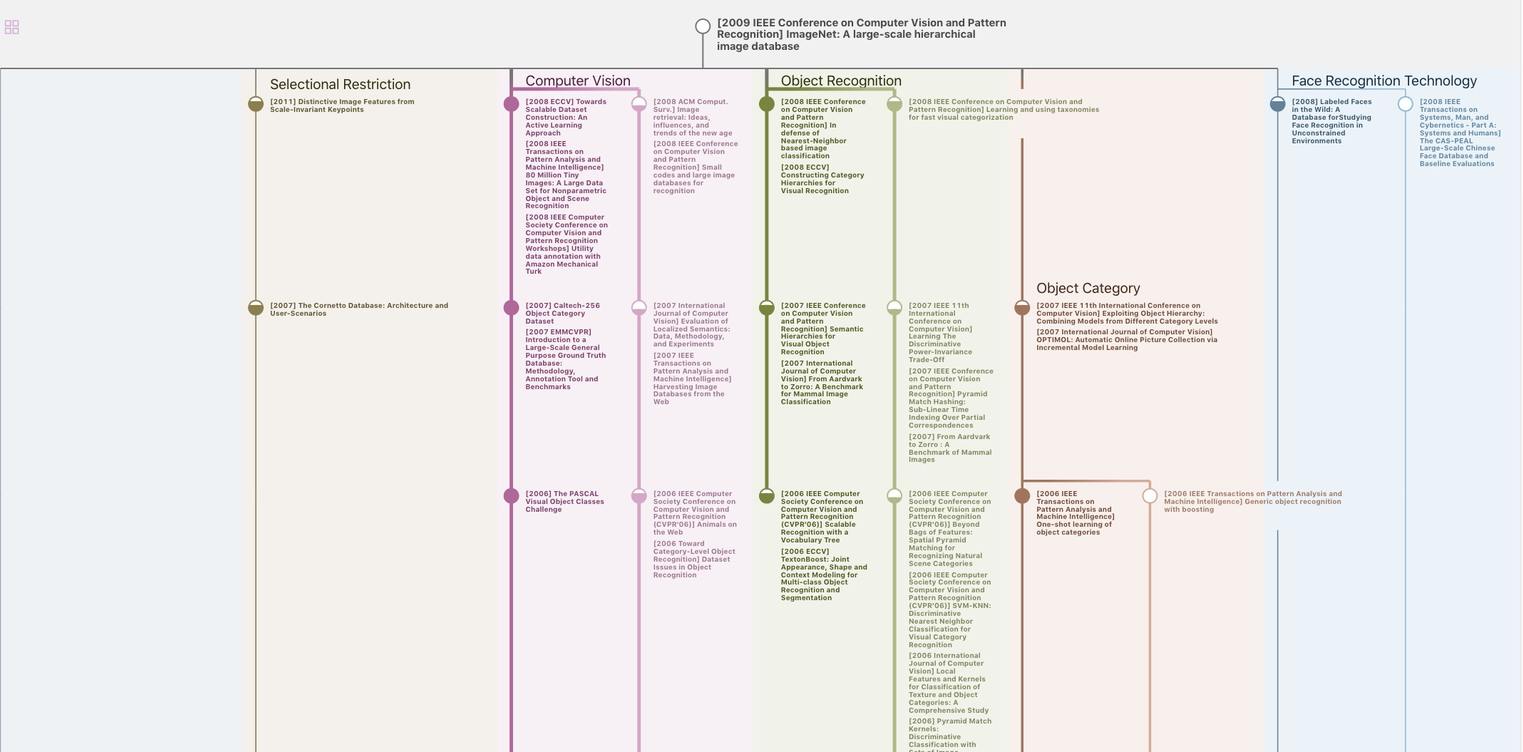
生成溯源树,研究论文发展脉络
Chat Paper
正在生成论文摘要