Automatic Data Augmentation for Domain Adapted Fine-Tuning of Self-Supervised Speech Representations
CoRR(2023)
摘要
Self-Supervised Learning (SSL) has allowed leveraging large amounts of unlabeled speech data to improve the performance of speech recognition models even with small annotated datasets. Despite this, speech SSL representations may fail while facing an acoustic mismatch between the pretraining and target datasets. To address this issue, we propose a novel supervised domain adaptation method, designed for cases exhibiting such a mismatch in acoustic domains. It consists in applying properly calibrated data augmentations on a large clean dataset, bringing it closer to the target domain, and using it as part of an initial fine-tuning stage. Augmentations are automatically selected through the minimization of a conditional-dependence estimator, based on the target dataset. The approach is validated during an oracle experiment with controlled distortions and on two amateur-collected low-resource domains, reaching better performances compared to the baselines in both cases.
更多查看译文
关键词
automatic data augmentation,speech,fine-tuning,self-supervised
AI 理解论文
溯源树
样例
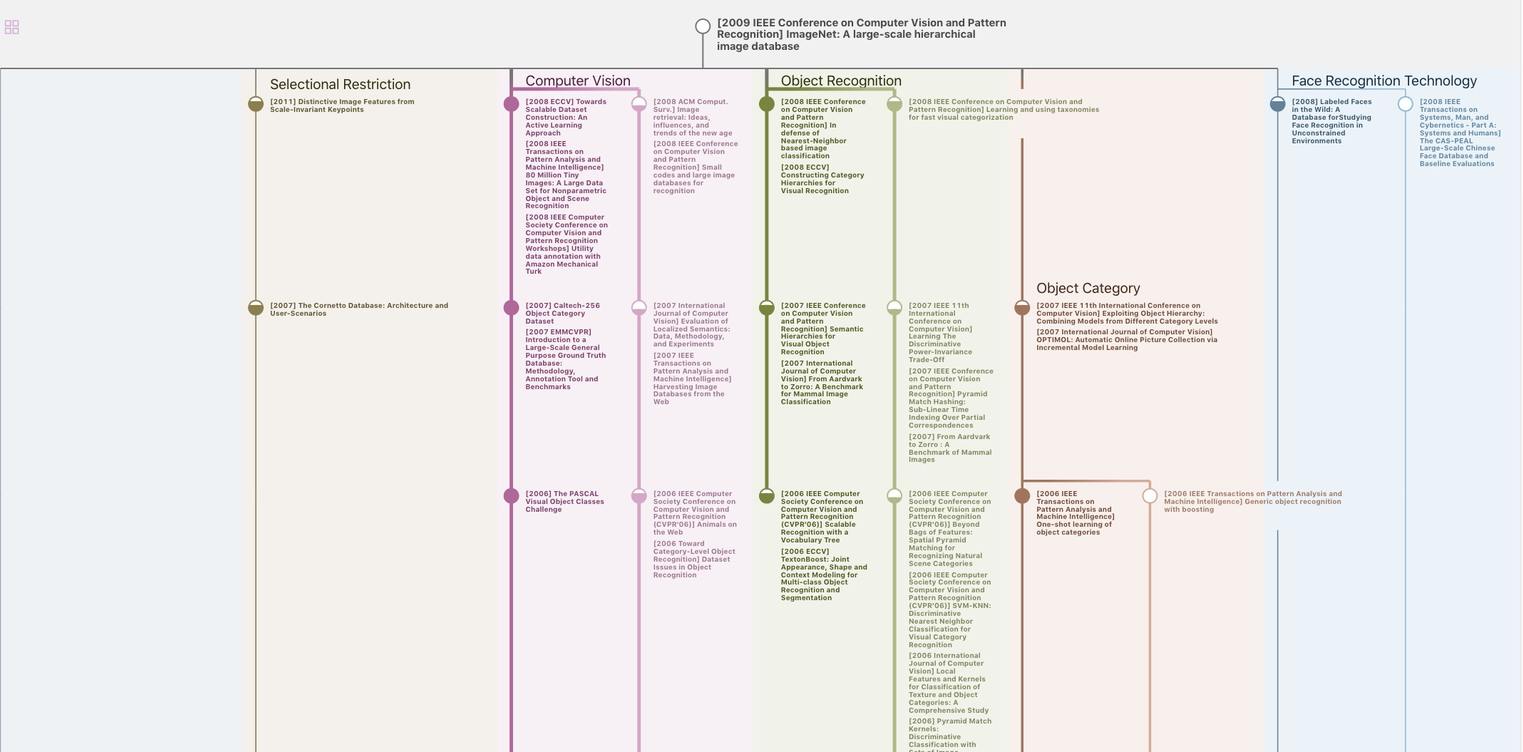
生成溯源树,研究论文发展脉络
Chat Paper
正在生成论文摘要