Example-based Motion Synthesis via Generative Motion Matching
CoRR(2023)
摘要
We present GenMM, a generative model that "mines" as many diverse motions as possible from a single or few example sequences. In stark contrast to existing data-driven methods, which typically require long offline training time, are prone to visual artifacts, and tend to fail on large and complex skeletons, GenMM inherits the training-free nature and the superior quality of the well-known Motion Matching method. GenMM can synthesize a high-quality motion within a fraction of a second, even with highly complex and large skeletal structures. At the heart of our generative framework lies the generative motion matching module, which utilizes the bidirectional visual similarity as a generative cost function to motion matching, and operates in a multi-stage framework to progressively refine a random guess using exemplar motion matches. In addition to diverse motion generation, we show the versatility of our generative framework by extending it to a number of scenarios that are not possible with motion matching alone, including motion completion, key frame-guided generation, infinite looping, and motion reassembly.
更多查看译文
关键词
motion synthesis,generative model,motion matching
AI 理解论文
溯源树
样例
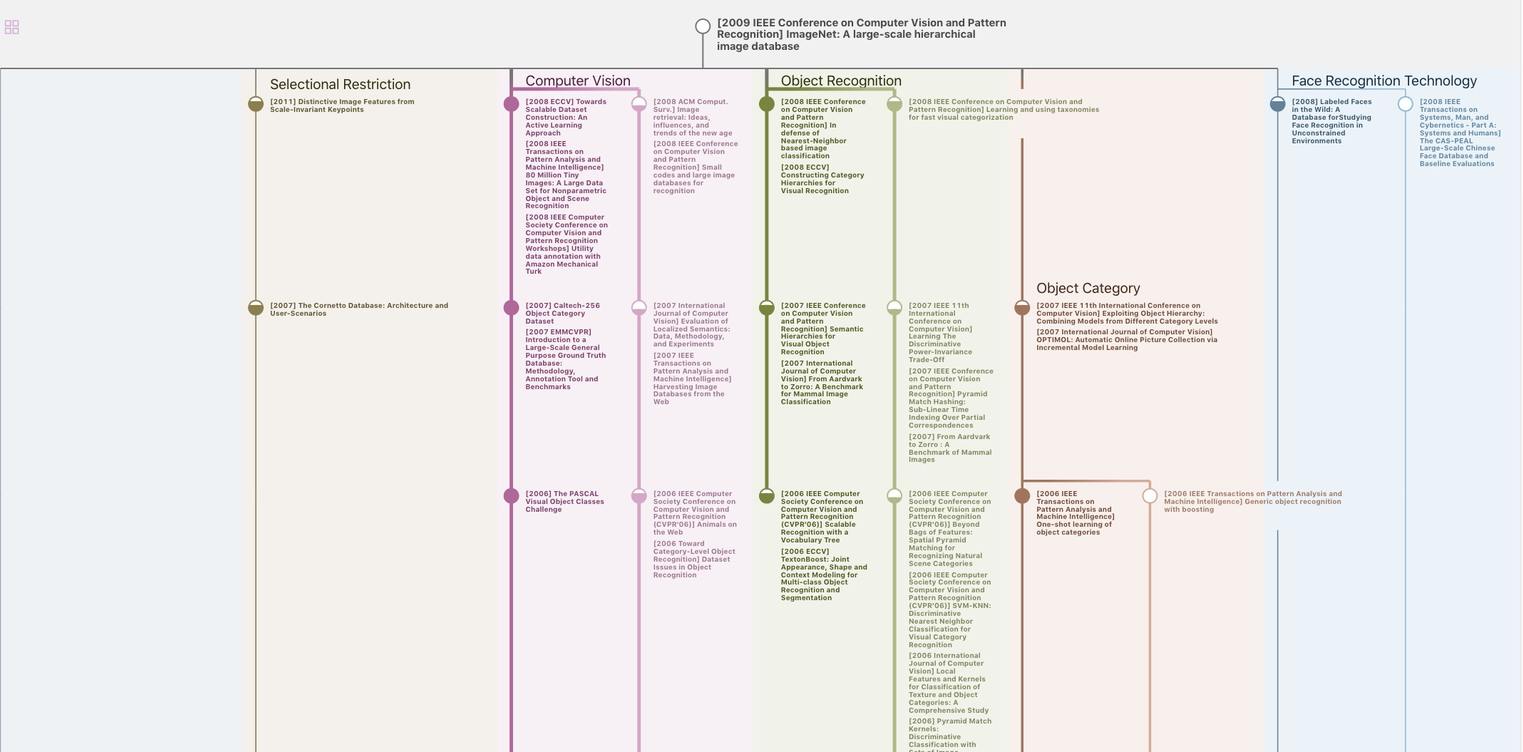
生成溯源树,研究论文发展脉络
Chat Paper
正在生成论文摘要