Zero-Shot Automatic Pronunciation Assessment
CoRR(2023)
摘要
Automatic Pronunciation Assessment (APA) is vital for computer-assisted language learning. Prior methods rely on annotated speech-text data to train Automatic Speech Recognition (ASR) models or speech-score data to train regression models. In this work, we propose a novel zero-shot APA method based on the pre-trained acoustic model, HuBERT. Our method involves encoding speech input and corrupting them via a masking module. We then employ the Transformer encoder and apply k-means clustering to obtain token sequences. Finally, a scoring module is designed to measure the number of wrongly recovered tokens. Experimental results on speechocean762 demonstrate that the proposed method achieves comparable performance to supervised regression baselines and outperforms non-regression baselines in terms of Pearson Correlation Coefficient (PCC). Additionally, we analyze how masking strategies affect the performance of APA.
更多查看译文
关键词
pronunciation,assessment,zero-shot
AI 理解论文
溯源树
样例
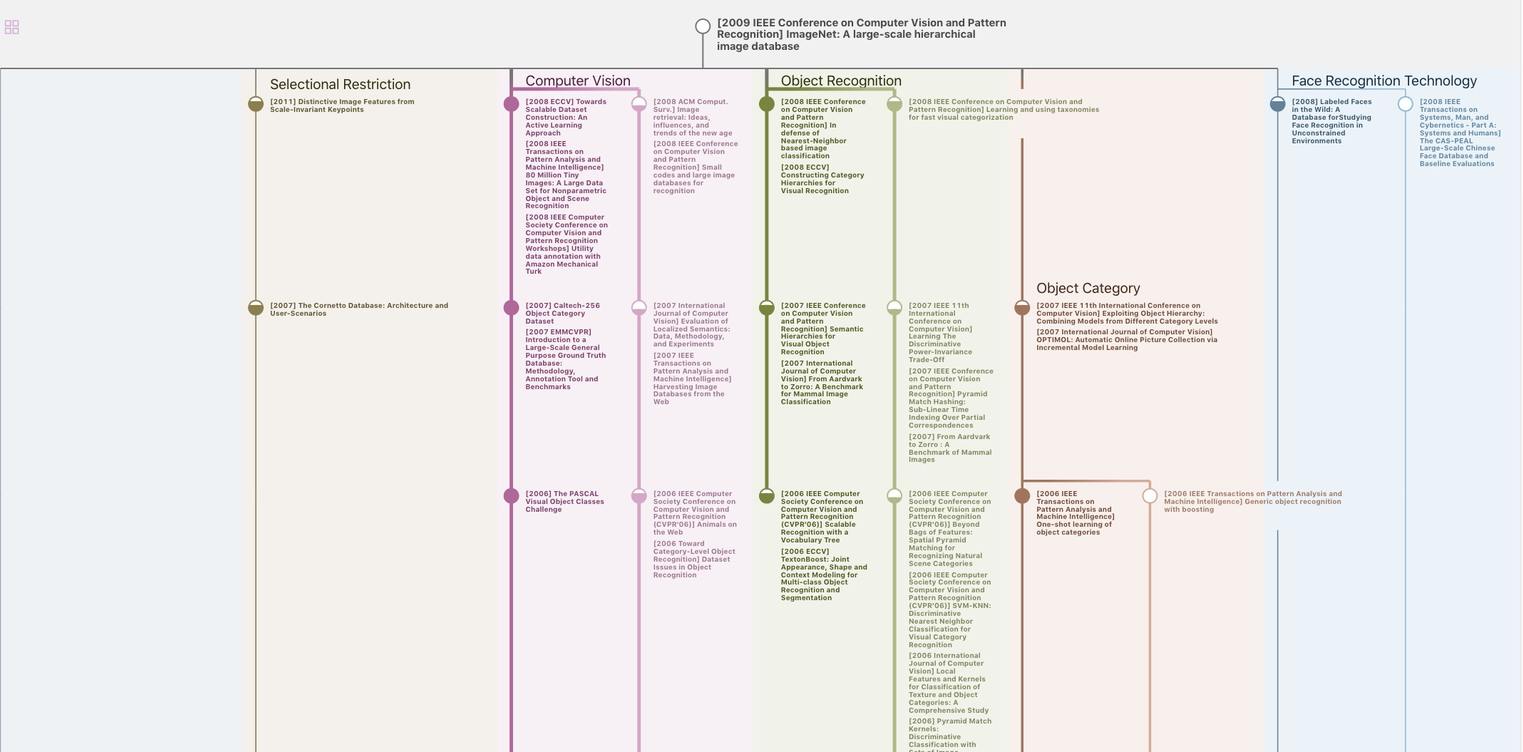
生成溯源树,研究论文发展脉络
Chat Paper
正在生成论文摘要