Video ControlNet: Towards Temporally Consistent Synthetic-to-Real Video Translation Using Conditional Image Diffusion Models
CoRR(2023)
摘要
In this study, we present an efficient and effective approach for achieving temporally consistent synthetic-to-real video translation in videos of varying lengths. Our method leverages off-the-shelf conditional image diffusion models, allowing us to perform multiple synthetic-to-real image generations in parallel. By utilizing the available optical flow information from the synthetic videos, our approach seamlessly enforces temporal consistency among corresponding pixels across frames. This is achieved through joint noise optimization, effectively minimizing spatial and temporal discrepancies. To the best of our knowledge, our proposed method is the first to accomplish diverse and temporally consistent synthetic-to-real video translation using conditional image diffusion models. Furthermore, our approach does not require any training or fine-tuning of the diffusion models. Extensive experiments conducted on various benchmarks for synthetic-to-real video translation demonstrate the effectiveness of our approach, both quantitatively and qualitatively. Finally, we show that our method outperforms other baseline methods in terms of both temporal consistency and visual quality.
更多查看译文
关键词
diffusion,translation,synthetic-to-real
AI 理解论文
溯源树
样例
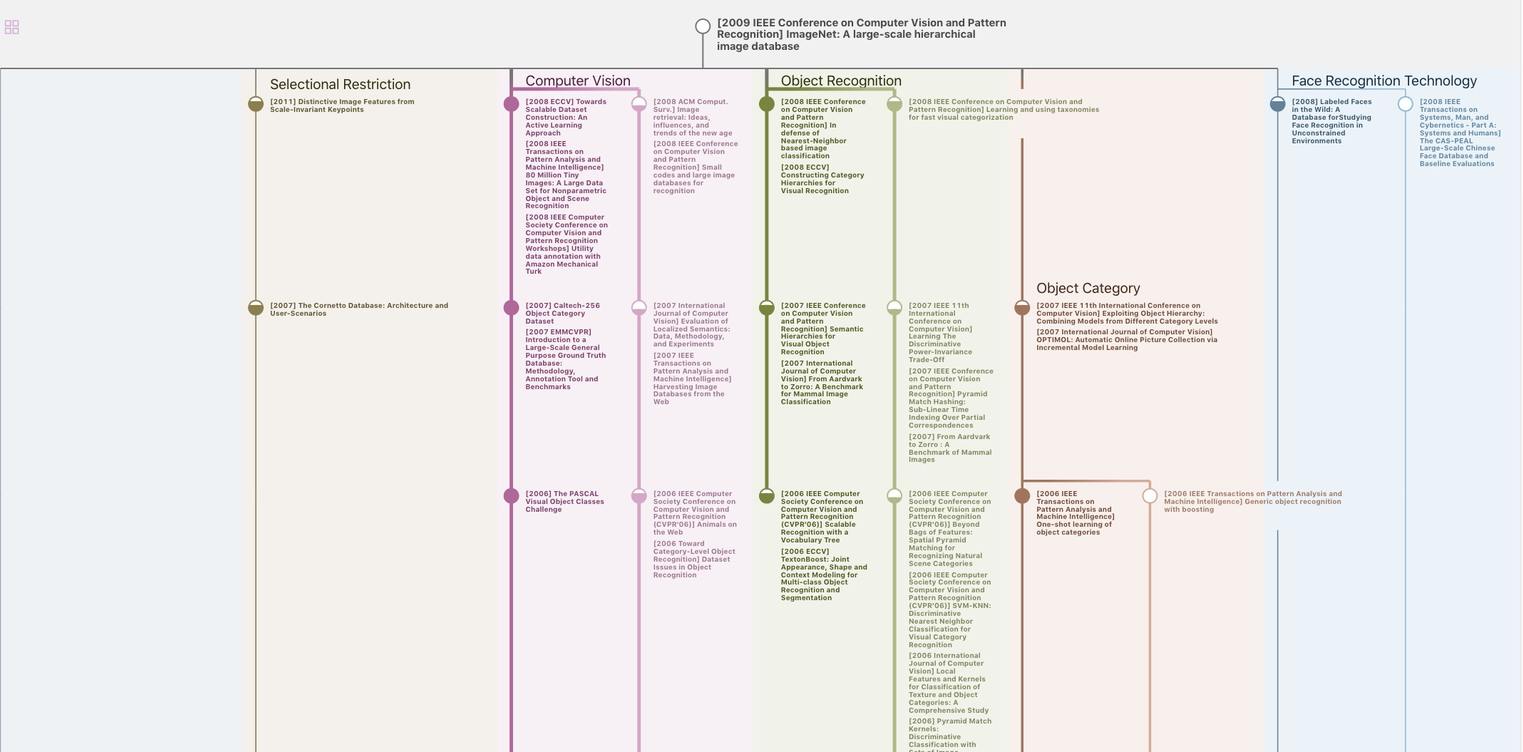
生成溯源树,研究论文发展脉络
Chat Paper
正在生成论文摘要