Cross Encoding as Augmentation: Towards Effective Educational Text Classification
conf_acl(2023)
摘要
Text classification in education, usually called auto-tagging, is the automated process of assigning relevant tags to educational content, such as questions and textbooks. However, auto-tagging suffers from a data scarcity problem, which stems from two major challenges: 1) it possesses a large tag space and 2) it is multi-label. Though a retrieval approach is reportedly good at low-resource scenarios, there have been fewer efforts to directly address the data scarcity problem. To mitigate these issues, here we propose a novel retrieval approach CEAA that provides effective learning in educational text classification. Our main contributions are as follows: 1) we leverage transfer learning from question-answering datasets, and 2) we propose a simple but effective data augmentation method introducing cross-encoder style texts to a bi-encoder architecture for more efficient inference. An extensive set of experiments shows that our proposed method is effective in multi-label scenarios and low-resource tags compared to state-of-the-art models.
更多查看译文
关键词
effective educational text
AI 理解论文
溯源树
样例
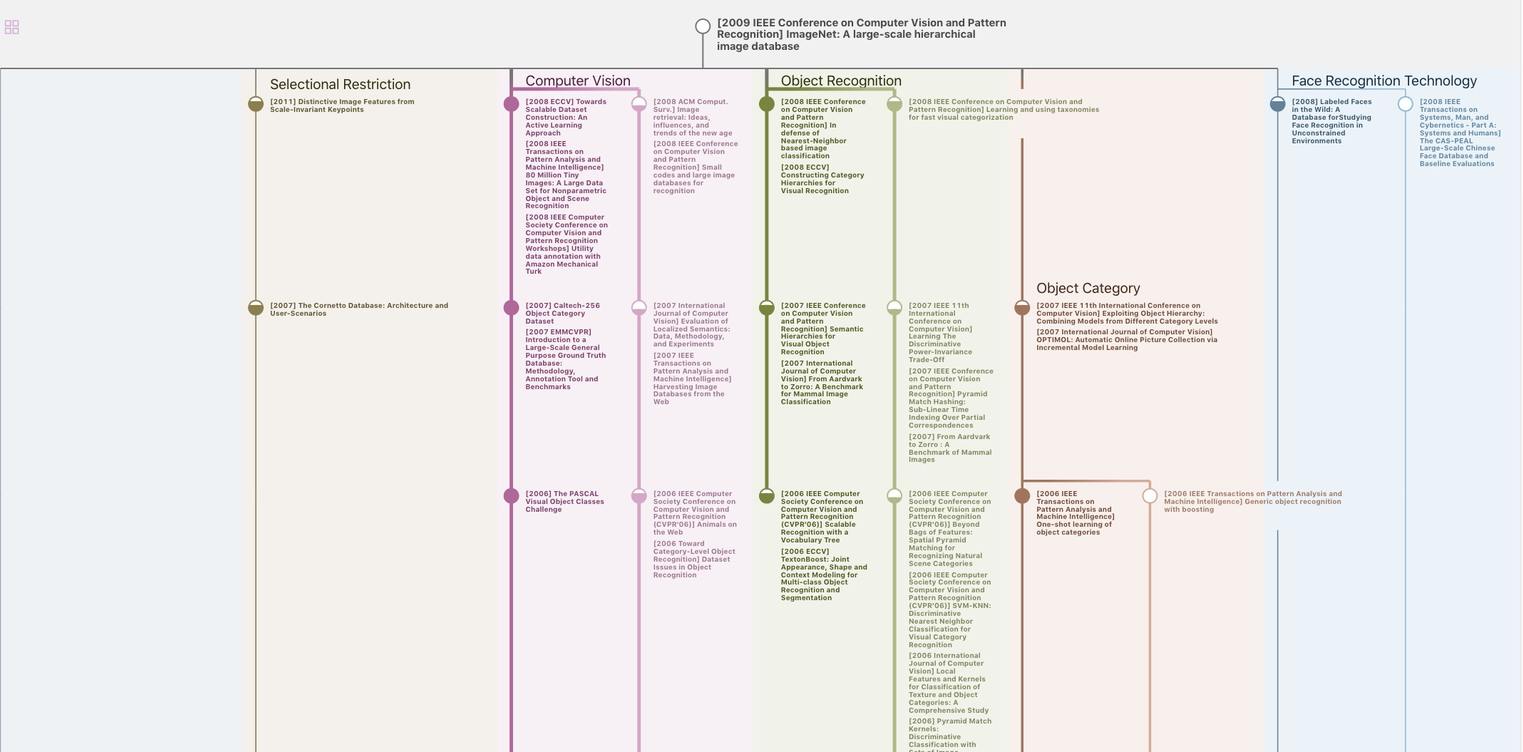
生成溯源树,研究论文发展脉络
Chat Paper
正在生成论文摘要