Large-scale Ridesharing DARP Instances Based on Real Travel Demand
CoRR(2023)
摘要
Accurately predicting the real-life performance of algorithms solving the Dial-a-Ride Problem (DARP) in the context of Mobility on Demand (MoD) systems with ridesharing requires evaluating them on representative instances. However, the benchmarking of state-of-the-art DARP solution methods has been limited to small, artificial instances or outdated non-public instances, hindering direct comparisons. With the rise of large MoD systems and the availability of open travel demand datasets for many US cities, there is now an opportunity to evaluate these algorithms on standardized, realistic, and representative instances. Despite the significant challenges involved in processing obfuscated and diverse datasets, we have developed a methodology using which we have created a comprehensive set of large-scale demand instances based on real-world data. These instances cover diverse use cases, one of which is demonstrated in an evaluation of two established DARP methods: the insertion heuristic and optimal vehicle-group assignment method. We publish the full results of both methods in a standardized format. The results show significant differences between areas in all measured quantities, emphasizing the importance of evaluating methods across different cities.
更多查看译文
关键词
Travel Behaviour,Real Travel,Dial-a-ride Problem,Solution Method,Time Window,Distance Matrix,Maximum Time,Travel Time,York City,Shortest Path,Open Data,Road Network,Boundary Area,Problem Instances,Speed Data,Demand Data,Maximum Delay,Start Location,Heuristic Solution,Set Of Vehicles,Ride-hailing,Destination Location
AI 理解论文
溯源树
样例
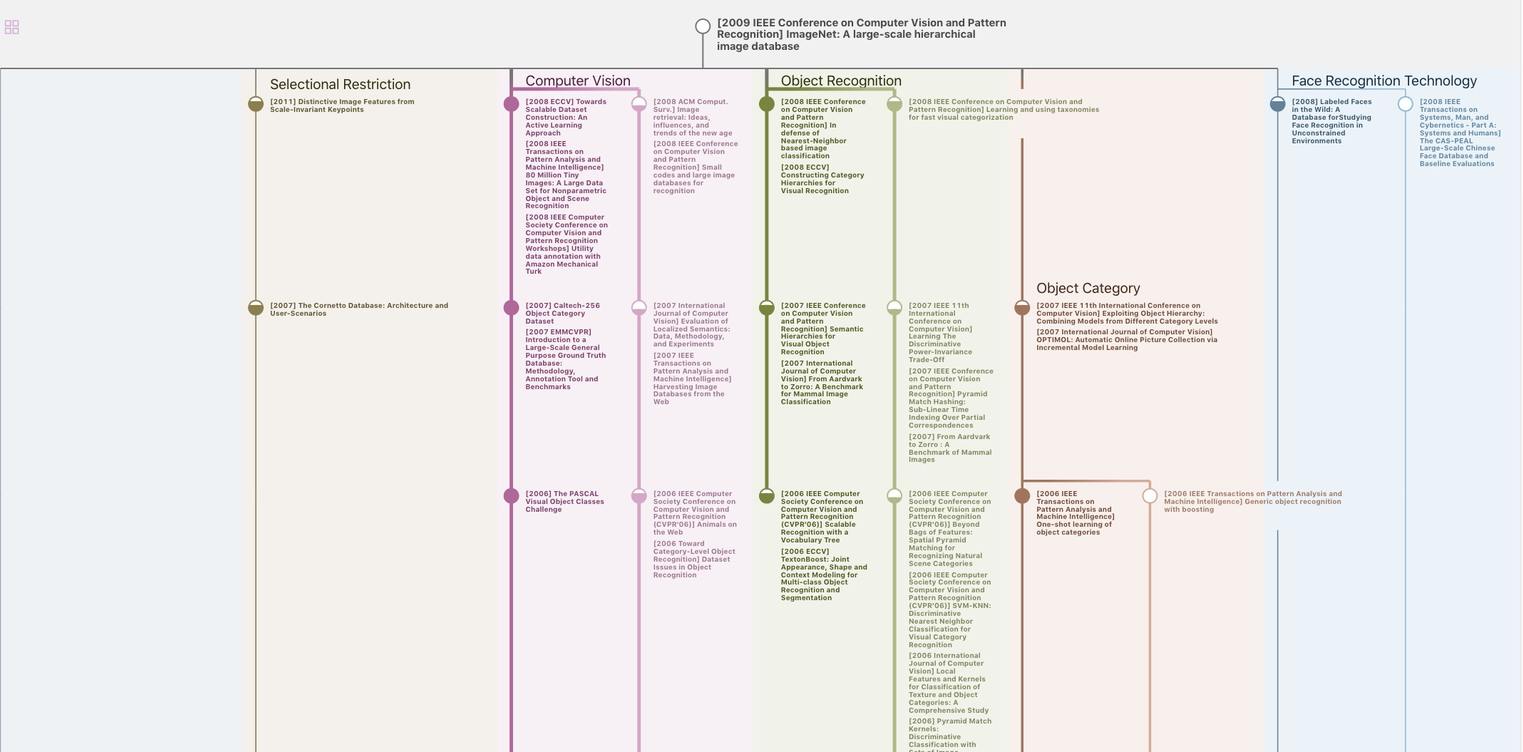
生成溯源树,研究论文发展脉络
Chat Paper
正在生成论文摘要