Quantum chi-squared tomography and mutual information testing
CoRR(2023)
摘要
For quantum state tomography on rank-$r$ dimension-$d$ states, we show that $\widetilde{O}(r^{.5}d^{1.5}/\epsilon) \leq \widetilde{O}(d^2/\epsilon)$ copies suffice for accuracy $\epsilon$ with respect to (Bures) $\chi^2$-divergence, and $\widetilde{O}(rd/\epsilon)$ copies suffice for accuracy $\epsilon$ with respect to quantum relative entropy. The best previous bound was $\widetilde{O}(rd/\epsilon) \leq \widetilde{O}(d^2/\epsilon)$ with respect to infidelity; our results are an improvement since \[ \text{infidelity} \leq \text{relative entropy} \leq \text{$\chi^2$-divergence}.\] For algorithms that are required to use single-copy measurements, we show that $\widetilde{O}(r^{1.5} d^{1.5}/\epsilon) \leq \widetilde{O}(d^3/\epsilon)$ copies suffice for $\chi^2$-divergence, and $\widetilde{O}(r^{2} d/\epsilon)$ suffice for relative entropy. Using this tomography algorithm, we show that $\widetilde{O}(d^{2.5}/\epsilon)$ copies of a $d\times d$-dimensional bipartite state suffice to test if it has quantum mutual information 0 or at least $\epsilon$. As a corollary, we also improve the best known sample complexity for the classical version of mutual information testing to $\widetilde{O}(d/\epsilon)$.
更多查看译文
关键词
quantum,tomography,chi-squared
AI 理解论文
溯源树
样例
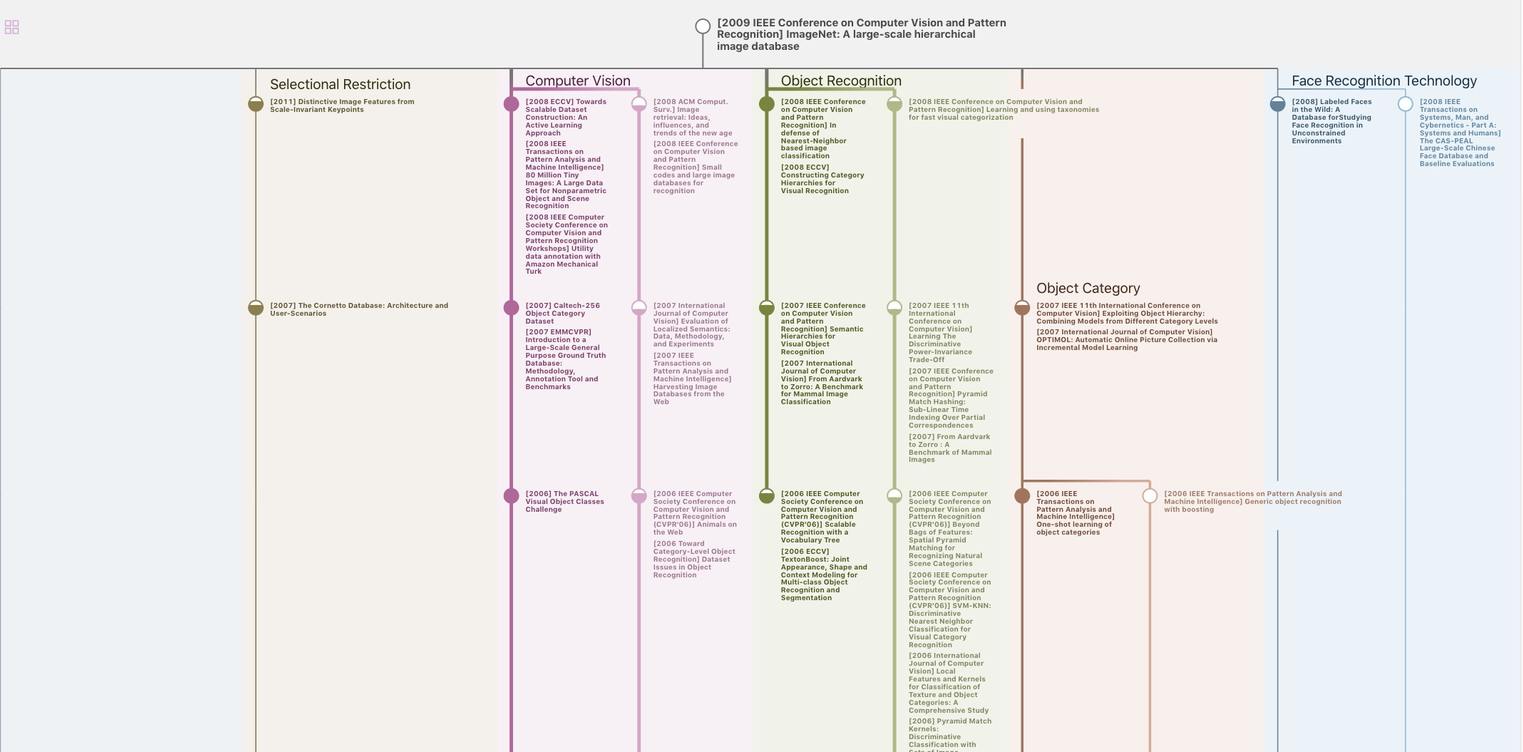
生成溯源树,研究论文发展脉络
Chat Paper
正在生成论文摘要