RLAD: Reinforcement Learning From Pixels for Autonomous Driving in Urban Environments
IEEE TRANSACTIONS ON AUTOMATION SCIENCE AND ENGINEERING(2023)
摘要
Current approaches of applied in urban focus on decoupling the perception training from the driving policy training. The main reason is to avoid training a convolution encoder alongside a policy network, which is known to have issues related to sample efficiency, degenerated feature representations, and catastrophic self-overfitting. However, this paradigm can lead to representations of the environment that are not aligned with the downstream task, which may result in suboptimal performances. To address this limitation, this paper proposes RLAD, the first method applied in the urban ad domain. We propose several techniques to enhance the performance of an rlfp algorithm in this domain, including: 1) an image encoder that leverages both image augmentations and layers; 2) WayConv1D, which is a waypoint encoder that harnesses the 2D geometrical information of the waypoints using 1D convolutions; and 3) an auxiliary loss to increase the significance of the traffic lights in the latent representation of the environment. Experimental results show that RLAD significantly outperforms all state-of-the-art rlfp methods on the NoCrash benchmark. We also present an infraction analysis on the NoCrash-regular benchmark, which indicates that RLAD performs better than all other methods in terms of both collision rate and red light infractions. The source code of RLAD is available at https://github.com/DanielCoelho112/rlad.
更多查看译文
关键词
Training,Task analysis,Reinforcement learning,Autonomous vehicles,Visualization,Convolution,Urban areas,Autonomous driving,reinforcement learning,deep learning,feature representation,deep neural networks
AI 理解论文
溯源树
样例
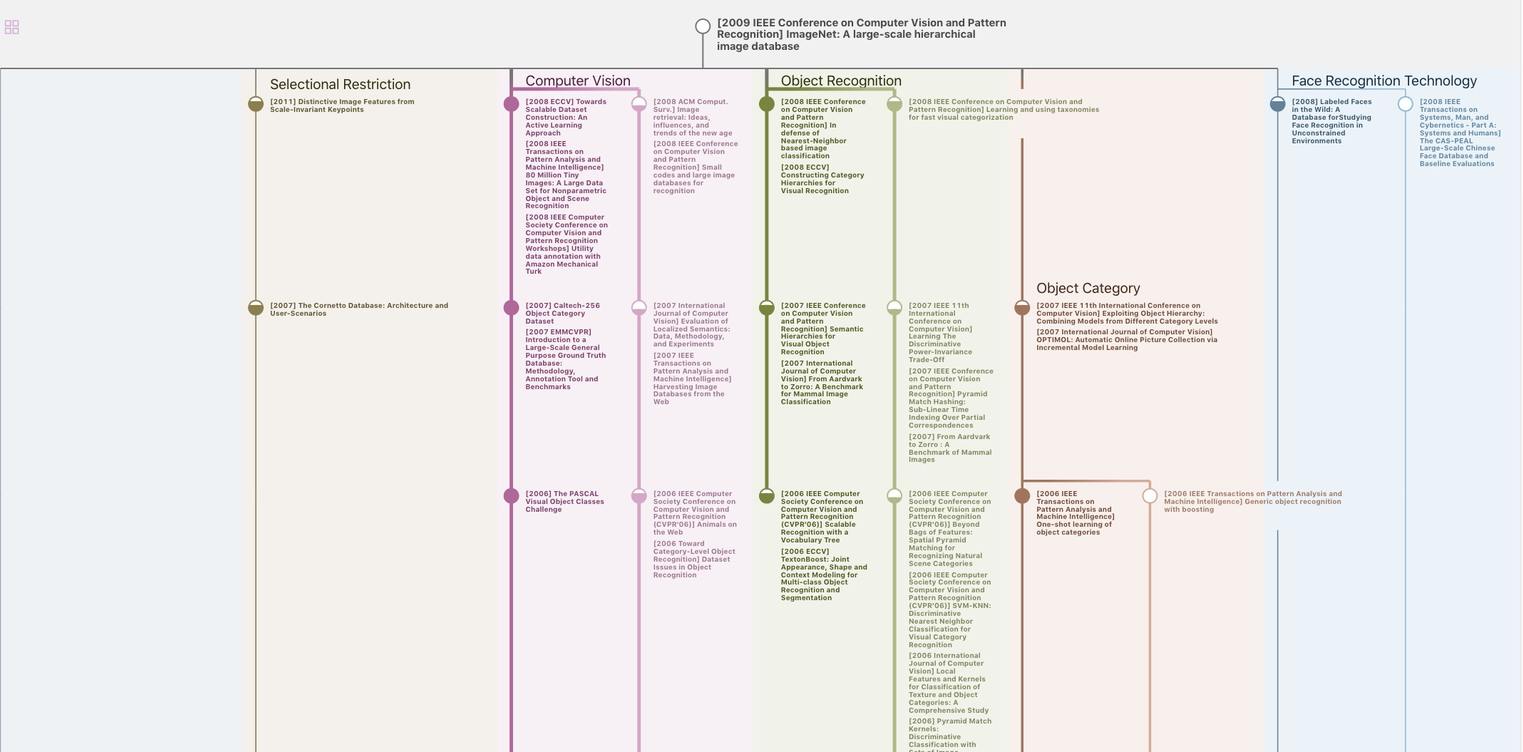
生成溯源树,研究论文发展脉络
Chat Paper
正在生成论文摘要