DeCoR: Defy Knowledge Forgetting by Predicting Earlier Audio Codes
CoRR(2023)
摘要
Lifelong audio feature extraction involves learning new sound classes incrementally, which is essential for adapting to new data distributions over time. However, optimizing the model only on new data can lead to catastrophic forgetting of previously learned tasks, which undermines the model's ability to perform well over the long term. This paper introduces a new approach to continual audio representation learning called DeCoR. Unlike other methods that store previous data, features, or models, DeCoR indirectly distills knowledge from an earlier model to the latest by predicting quantization indices from a delayed codebook. We demonstrate that DeCoR improves acoustic scene classification accuracy and integrates well with continual self-supervised representation learning. Our approach introduces minimal storage and computation overhead, making it a lightweight and efficient solution for continual learning.
更多查看译文
关键词
defy knowledge forgetting,codes
AI 理解论文
溯源树
样例
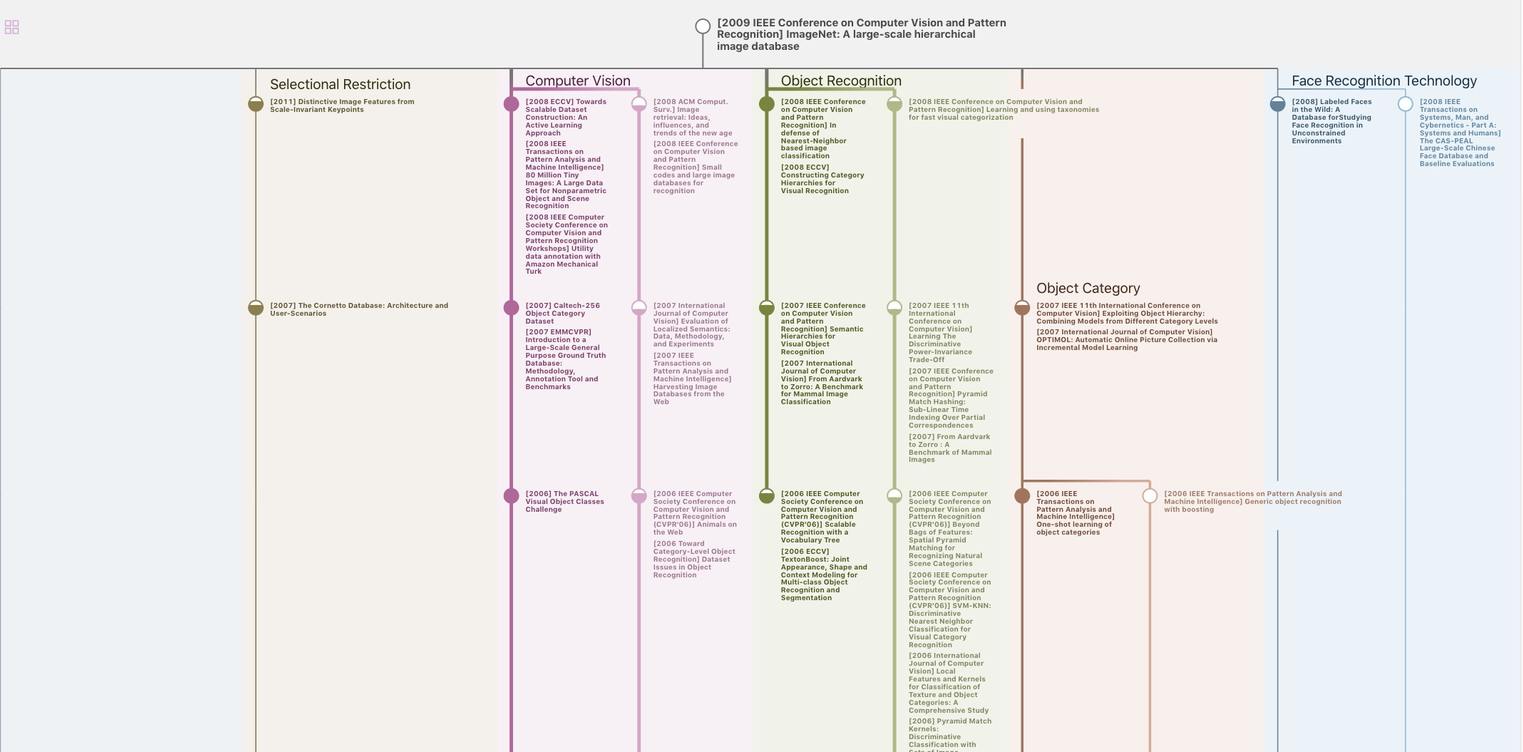
生成溯源树,研究论文发展脉络
Chat Paper
正在生成论文摘要