Communication Efficient Distributed Newton Method with Fast Convergence Rates
PROCEEDINGS OF THE 29TH ACM SIGKDD CONFERENCE ON KNOWLEDGE DISCOVERY AND DATA MINING, KDD 2023(2023)
摘要
We propose a communication and computation efficient second-order method for distributed optimization. For each iteration, our method only requires $\mathcal{O}(d)$ communication complexity, where $d$ is the problem dimension. We also provide theoretical analysis to show the proposed method has the similar convergence rate as the classical second-order optimization algorithms. Concretely, our method can find~$\big(\epsilon, \sqrt{dL\epsilon}\,\big)$-second-order stationary points for nonconvex problem by $\mathcal{O}\big(\sqrt{dL}\,\epsilon^{-3/2}\big)$ iterations, where $L$ is the Lipschitz constant of Hessian. Moreover, it enjoys a local superlinear convergence under the strongly-convex assumption. Experiments on both convex and nonconvex problems show that our proposed method performs significantly better than baselines.
更多查看译文
关键词
Distributed Optimization,Second-Order Methods
AI 理解论文
溯源树
样例
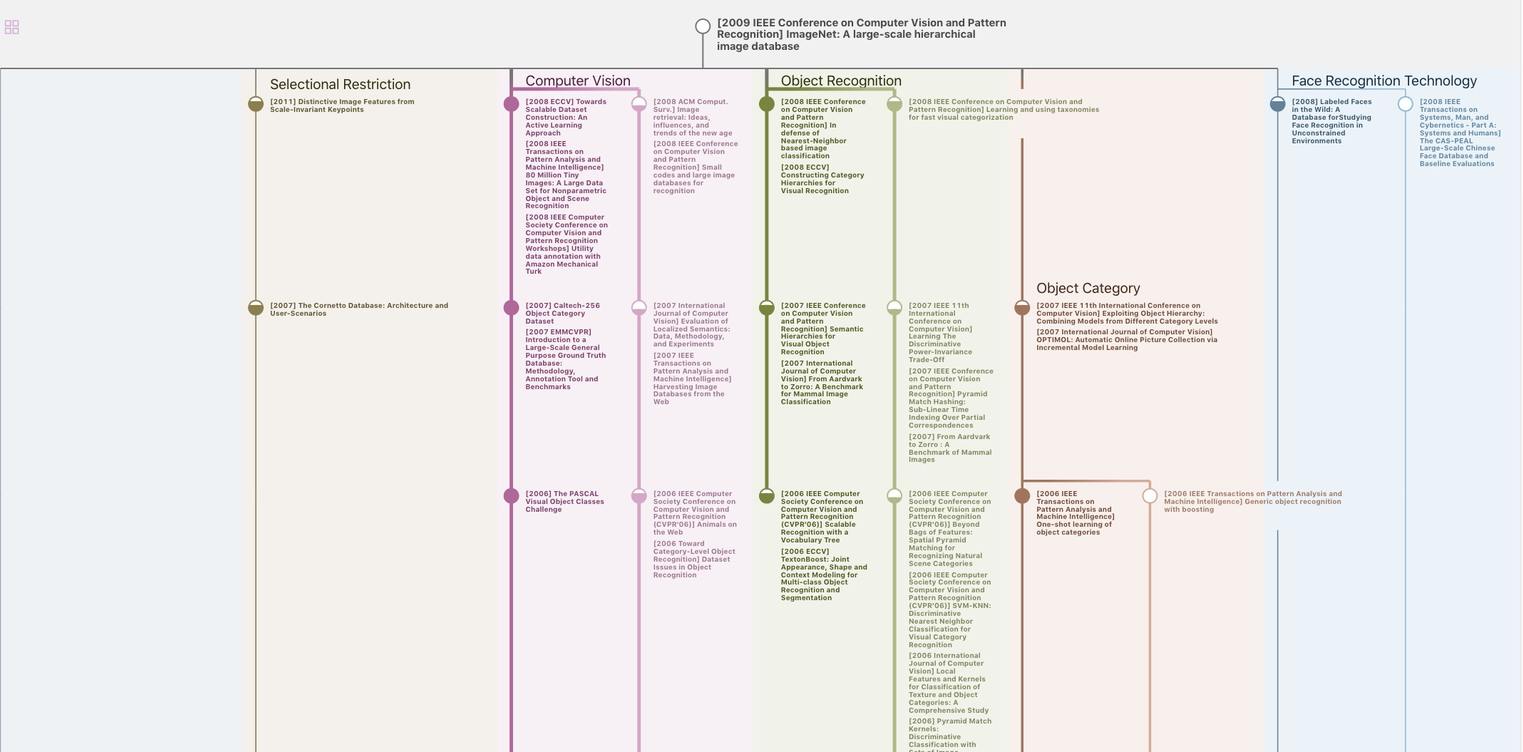
生成溯源树,研究论文发展脉络
Chat Paper
正在生成论文摘要