E-NER: Evidential Deep Learning for Trustworthy Named Entity Recognition
conf_acl(2023)
摘要
Most named entity recognition (NER) systems focus on improving model performance, ignoring the need to quantify model uncertainty, which is critical to the reliability of NER systems in open environments. Evidential deep learning (EDL) has recently been proposed as a promising solution to explicitly model predictive uncertainty for classification tasks. However, directly applying EDL to NER applications faces two challenges, i.e., the problems of sparse entities and OOV/OOD entities in NER tasks. To address these challenges, we propose a trustworthy NER framework named E-NER by introducing two uncertainty-guided loss terms to the conventional EDL, along with a series of uncertainty-guided training strategies. Experiments show that E-NER can be applied to multiple NER paradigms to obtain accurate uncertainty estimation. Furthermore, compared to state-of-the-art baselines, the proposed method achieves a better OOV/OOD detection performance and better generalization ability on OOV entities.
更多查看译文
关键词
trustworthy named entity recognition,evidential deep learning,deep learning,e-ner
AI 理解论文
溯源树
样例
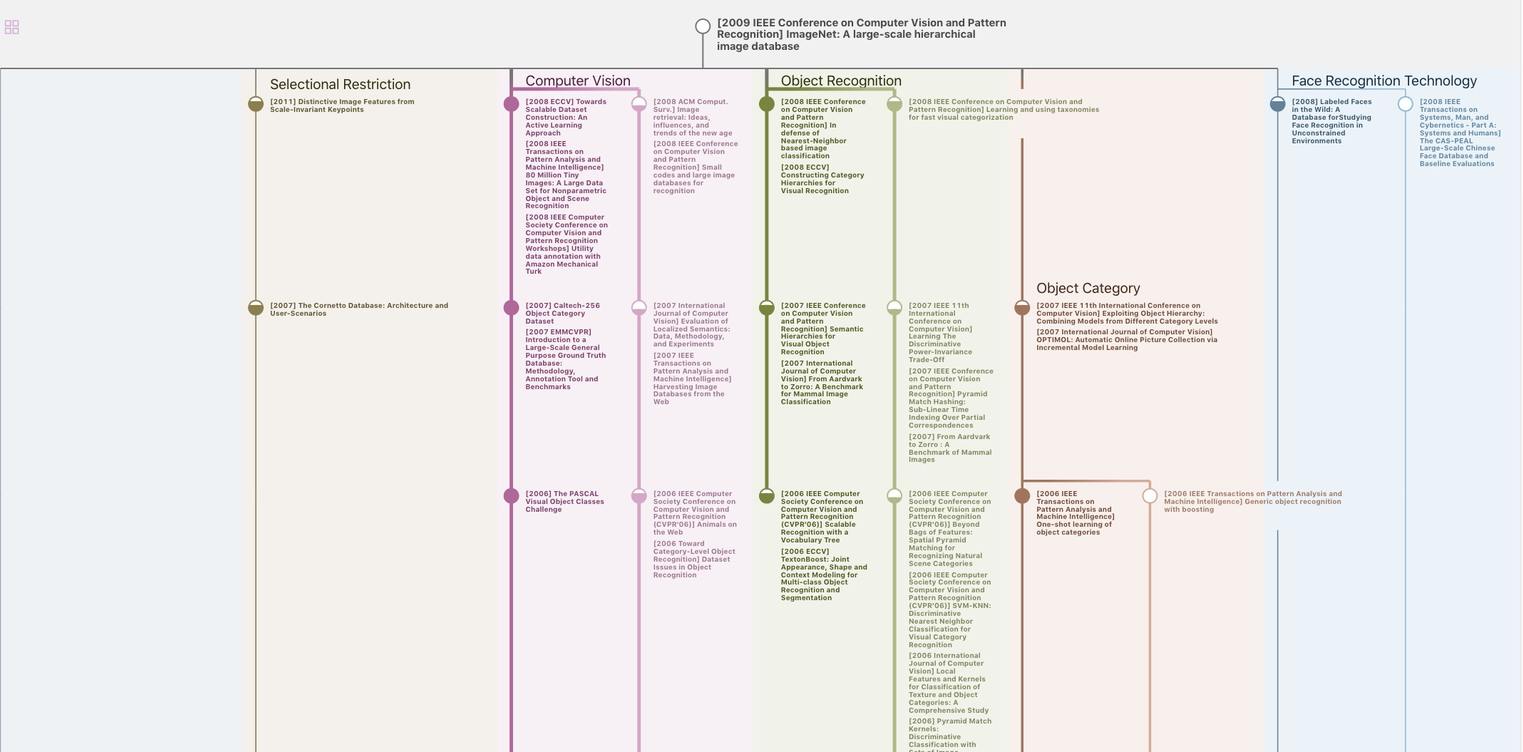
生成溯源树,研究论文发展脉络
Chat Paper
正在生成论文摘要