Demystifying Latschev's Theorem: Manifold Reconstruction from Noisy Data
arxiv(2023)
摘要
For a closed Riemannian manifold $\mathcal{M}$ and a metric space $S$ with a small Gromov$\unicode{x2013}$Hausdorff distance to it, Latschev's theorem guarantees the existence of a sufficiently small scale $\beta>0$ at which the Vietoris$\unicode{x2013}$Rips complex of $S$ is homotopy equivalent to $\mathcal{M}$. Despite being regarded as a stepping stone to the topological reconstruction of Riemannian manifolds from a noisy data, the result is only a qualitative guarantee. Until now, it had been elusive how to quantitatively choose such a proximity scale $\beta$ in order to provide sampling conditions for $S$ to be homotopy equivalent to $\mathcal{M}$. In this paper, we prove a stronger and pragmatic version of Latschev's theorem, facilitating a simple description of $\beta$ using the sectional curvatures and convexity radius of $\mathcal{M}$ as the sampling parameters. Our study also delves into the topological recovery of a closed Euclidean submanifold from the Vietoris$\unicode{x2013}$Rips complexes of a Hausdorff close Euclidean subset. As already known for \v{C}ech complexes, we show that Vietoris$\unicode{x2013}$Rips complexes also provide topologically faithful reconstruction guarantees for submanifolds. In the Euclidean case, our sampling conditions$\unicode{x2014}$using only the reach of the submanifold$\unicode{x2014}$turns out to be much simpler than the previously known reconstruction results using weak feature size and $\mu\unicode{x2013}$reach.
更多查看译文
关键词
manifold reconstruction,noisy data,latschev
AI 理解论文
溯源树
样例
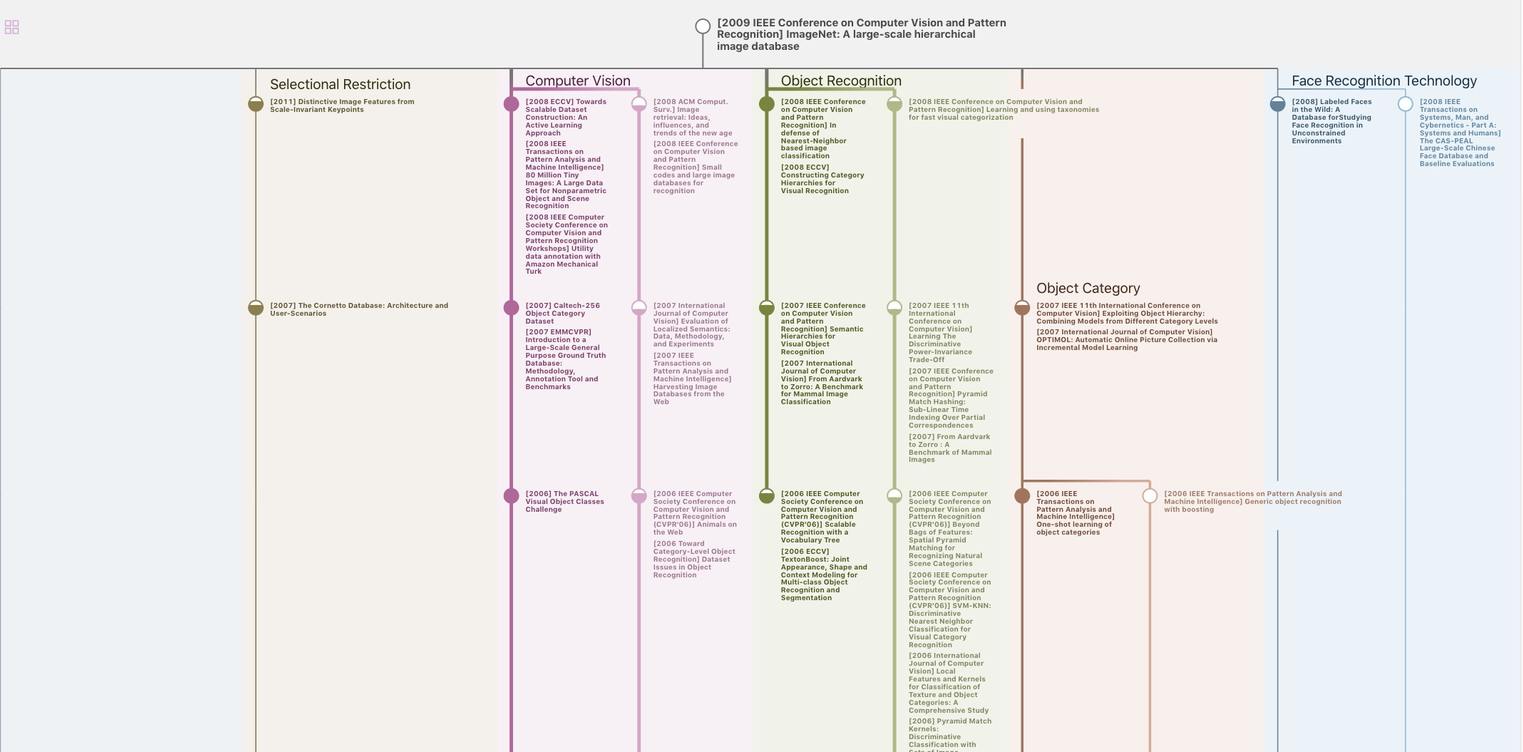
生成溯源树,研究论文发展脉络
Chat Paper
正在生成论文摘要