Learning Multiple Tasks with Non-stationary Interdependencies in Autonomous Robots
AAMAS '23: Proceedings of the 2023 International Conference on Autonomous Agents and Multiagent Systems(2023)
摘要
An important challenge in the field of autonomous open-ended learning is the autonomous learning of interdependent tasks, and in particular when such interdependencies are non-stationary, so that the robot has to modify the acquired knowledge to properly sequence goals that constitute preconditions for other ones. This work proposes a hierarchical robotic architecture to address this type of scenarios, allowing for the autonomous learning of both the skills necessary to achieve the multiple goals, and of the sequences reflecting the relations between them. Moreover, our system is endowed with a mechanism that, on the basis of self-estimated competence over goal achievement, is able to self-tune the exploration-exploitation balance to cope with the non-stationarity of the environment. The architecture is tested using an UR5e robot operating in a scenario where it should autonomously learn to accomplish various manipulation tasks.
更多查看译文
AI 理解论文
溯源树
样例
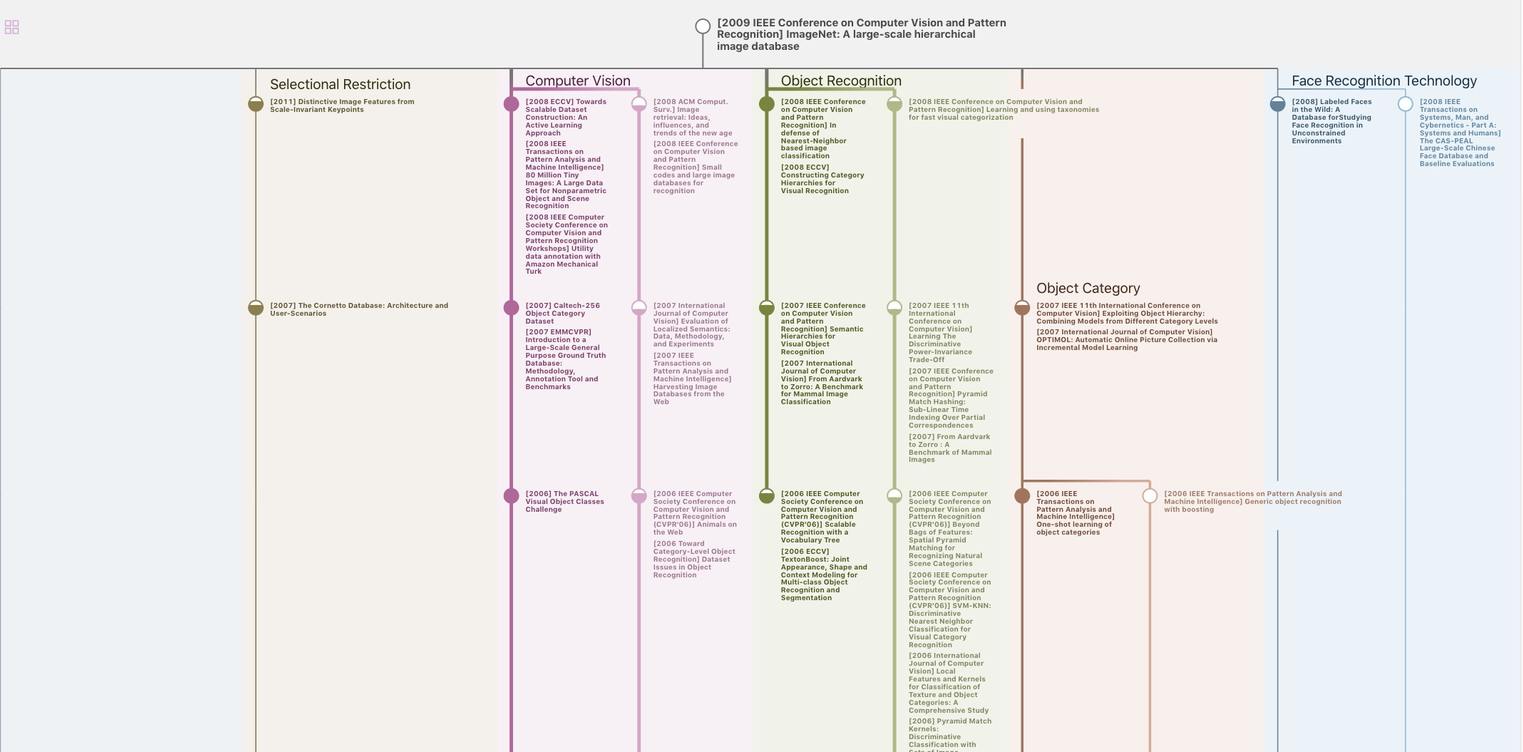
生成溯源树,研究论文发展脉络
Chat Paper
正在生成论文摘要