Expand, Rerank, and Retrieve: Query Reranking for Open-Domain Question Answering
conf_acl(2023)
摘要
We propose EAR, a query Expansion And Reranking approach for improving passage retrieval, with the application to open-domain question answering. EAR first applies a query expansion model to generate a diverse set of queries, and then uses a query reranker to select the ones that could lead to better retrieval results. Motivated by the observation that the best query expansion often is not picked by greedy decoding, EAR trains its reranker to predict the rank orders of the gold passages when issuing the expanded queries to a given retriever. By connecting better the query expansion model and retriever, EAR significantly enhances a traditional sparse retrieval method, BM25. Empirically, EAR improves top-5/20 accuracy by 3-8 and 5-10 points in in-domain and out-of-domain settings, respectively, when compared to a vanilla query expansion model, GAR, and a dense retrieval model, DPR.
更多查看译文
关键词
rerank,answering,query,retrieve,open-domain
AI 理解论文
溯源树
样例
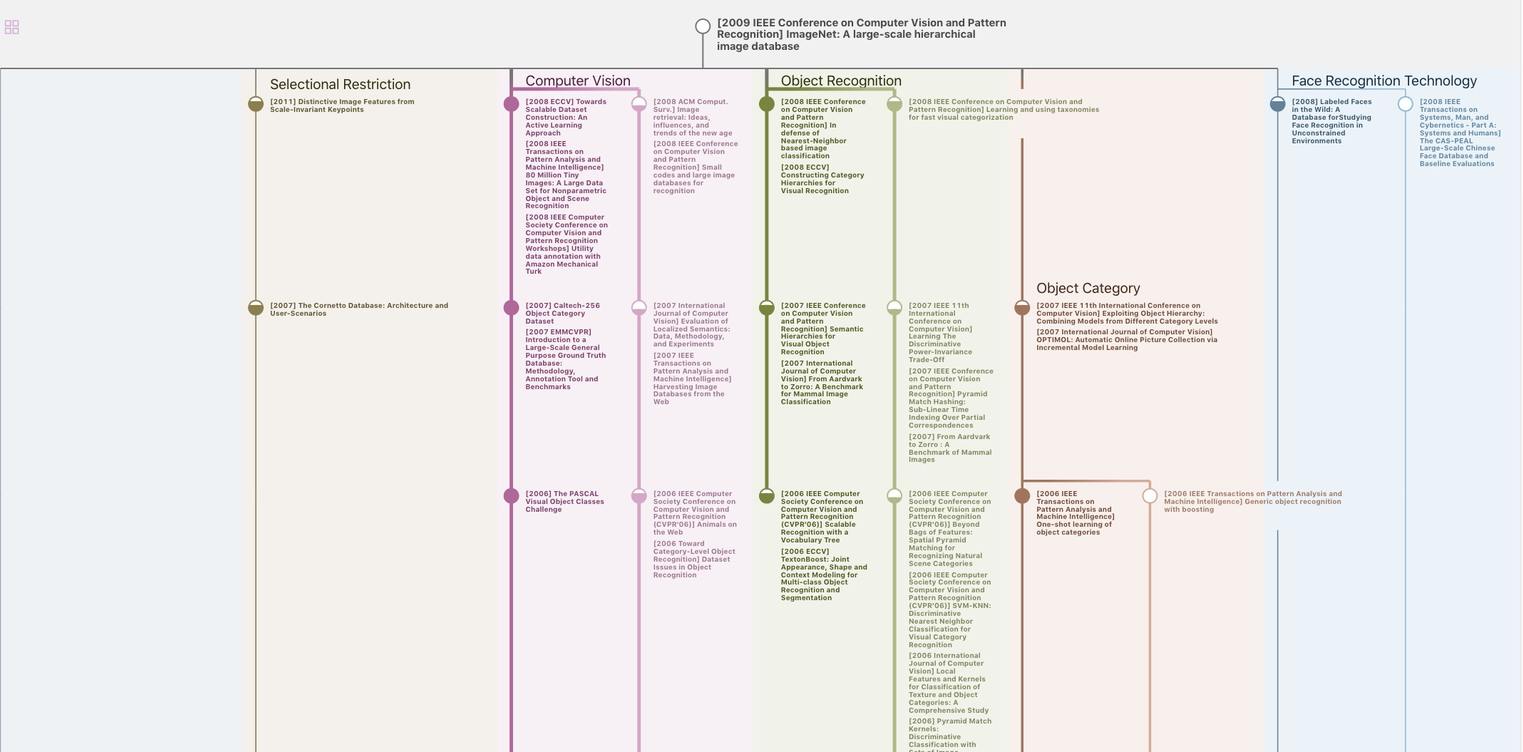
生成溯源树,研究论文发展脉络
Chat Paper
正在生成论文摘要