Modulate Your Spectrum in Self-Supervised Learning
arXiv (Cornell University)(2023)
摘要
Whitening loss provides theoretical guarantee in avoiding feature collapse for self-supervised learning (SSL) using joint embedding architectures. One typical implementation of whitening loss is hard whitening that designs whitening transformation over embedding and imposes the loss on the whitened output. In this paper, we propose spectral transformation (ST) framework to map the spectrum of embedding to a desired distribution during forward pass, and to modulate the spectrum of embedding by implicit gradient update during backward pass. We show that whitening transformation is a special instance of ST by definition, and there exist other instances that can avoid collapse by our empirical investigation. Furthermore, we propose a new instance of ST, called IterNorm with trace loss (INTL). We theoretically prove that INTL can avoid collapse and modulate the spectrum of embedding towards an equal-eigenvalue distribution during the course of optimization. Moreover, INTL achieves 76.6% top-1 accuracy in linear evaluation on ImageNet using ResNet-50, which exceeds the performance of the supervised baseline, and this result is obtained by using a batch size of only 256. Comprehensive experiments show that INTL is a promising SSL method in practice. The code is available at https://github.com/winci-ai/intl.
更多查看译文
关键词
spectrum,learning,self-supervised
AI 理解论文
溯源树
样例
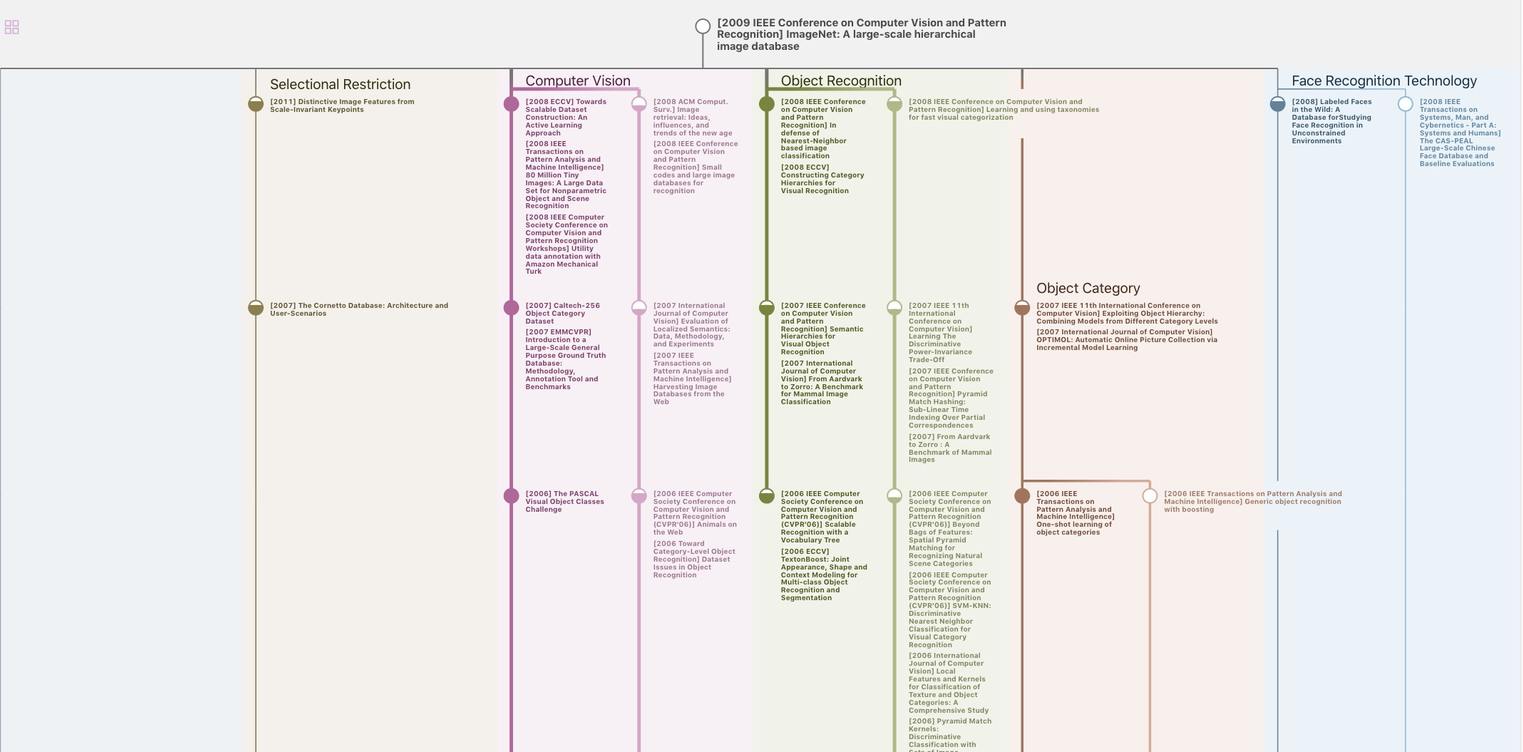
生成溯源树,研究论文发展脉络
Chat Paper
正在生成论文摘要