Counterfactual reasoning: Testing language models' understanding of hypothetical scenarios
conf_acl(2023)
摘要
Current pre-trained language models have enabled remarkable improvements in downstream tasks, but it remains difficult to distinguish effects of statistical correlation from more systematic logical reasoning grounded on the understanding of real world. We tease these factors apart by leveraging counterfactual conditionals, which force language models to predict unusual consequences based on hypothetical propositions. We introduce a set of tests from psycholinguistic experiments, as well as larger-scale controlled datasets, to probe counterfactual predictions from five pre-trained language models. We find that models are consistently able to override real-world knowledge in counterfactual scenarios, and that this effect is more robust in case of stronger baseline world knowledge -- however, we also find that for most models this effect appears largely to be driven by simple lexical cues. When we mitigate effects of both world knowledge and lexical cues to test knowledge of linguistic nuances of counterfactuals, we find that only GPT-3 shows sensitivity to these nuances, though this sensitivity is also non-trivially impacted by lexical associative factors.
更多查看译文
关键词
counterfactual reasoning,hypothetical scenarios,language models,testing
AI 理解论文
溯源树
样例
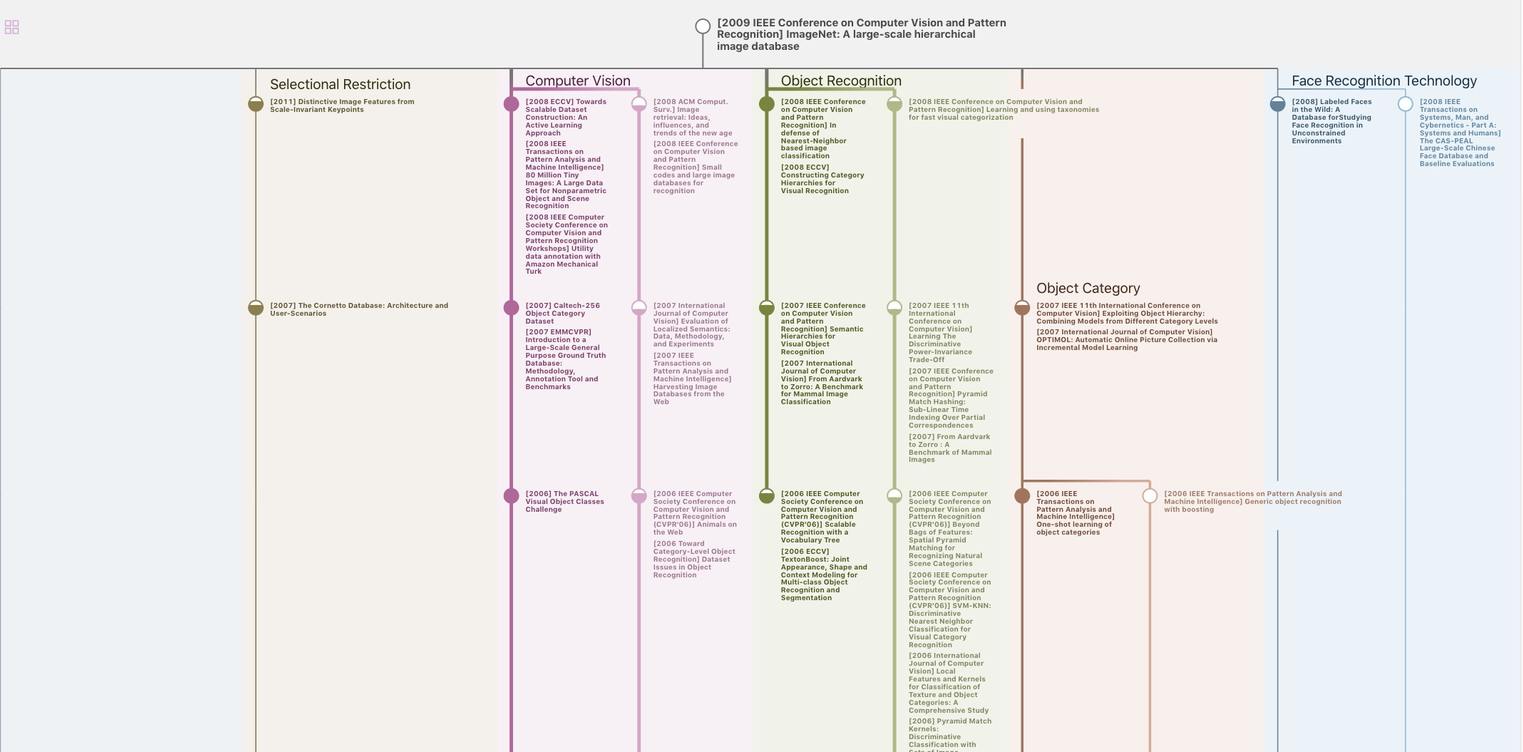
生成溯源树,研究论文发展脉络
Chat Paper
正在生成论文摘要