Efficient Bound of Lipschitz Constant for Convolutional Layers by Gram Iteration
CoRR(2023)
摘要
Since the control of the Lipschitz constant has a great impact on the training stability, generalization, and robustness of neural networks, the estimation of this value is nowadays a real scientific challenge. In this paper we introduce a precise, fast, and differentiable upper bound for the spectral norm of convolutional layers using circulant matrix theory and a new alternative to the Power iteration. Called the Gram iteration, our approach exhibits a superlinear convergence. First, we show through a comprehensive set of experiments that our approach outperforms other state-of-the-art methods in terms of precision, computational cost, and scalability. Then, it proves highly effective for the Lipschitz regularization of convolutional neural networks, with competitive results against concurrent approaches.
更多查看译文
关键词
convolutional layers,lipschitz constant,gram
AI 理解论文
溯源树
样例
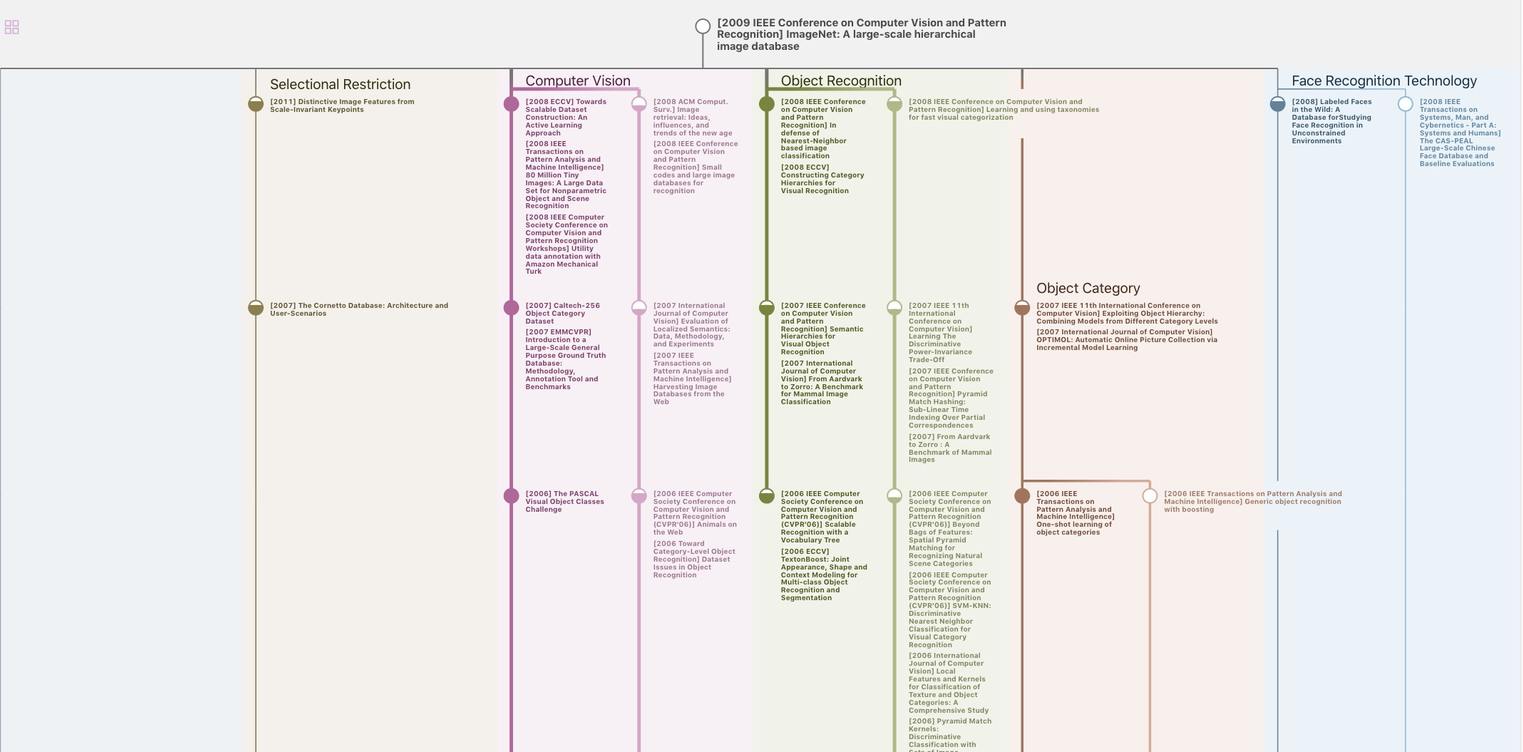
生成溯源树,研究论文发展脉络
Chat Paper
正在生成论文摘要