Masked and Permuted Implicit Context Learning for Scene Text Recognition
IEEE SIGNAL PROCESSING LETTERS(2024)
摘要
Scene Text Recognition (STR) is challenging because of various text styles, shapes, and backgrounds. Although the integration of linguistic information enhances models' performance, existing methods based on either permuted language modeling (PLM) or masked language modeling (MLM) have their drawbacks. PLM's autoregressive decoding lacks foresight into subsequent characters, while MLM overlooks inter-character dependencies. To address these problems, we propose a masked and permuted implicit context learning network for STR, which unifies PLM and MLM within a single decoder, inheriting the advantages of both approaches. We utilize the training procedure of PLM and incorporate word length information into the decoding process to integrate MLM, substituting the undetermined characters with mask tokens. Besides, we employ the perturbation training technique to train a more robust model against potential length prediction errors. Our comprehensive evaluations demonstrate the performance of our model. It achieves superior performance on the popularly used benchmarks and outperforms previous state-of-the-art methods with a substantial improvement of 9.1% on the more challenging Union14M-Benchmark.
更多查看译文
关键词
Decoding,Training,Context modeling,Predictive models,Iterative decoding,Visualization,Benchmark testing,Autoregressive,language modeling,non-autoregressive,OCR,scene text recognition
AI 理解论文
溯源树
样例
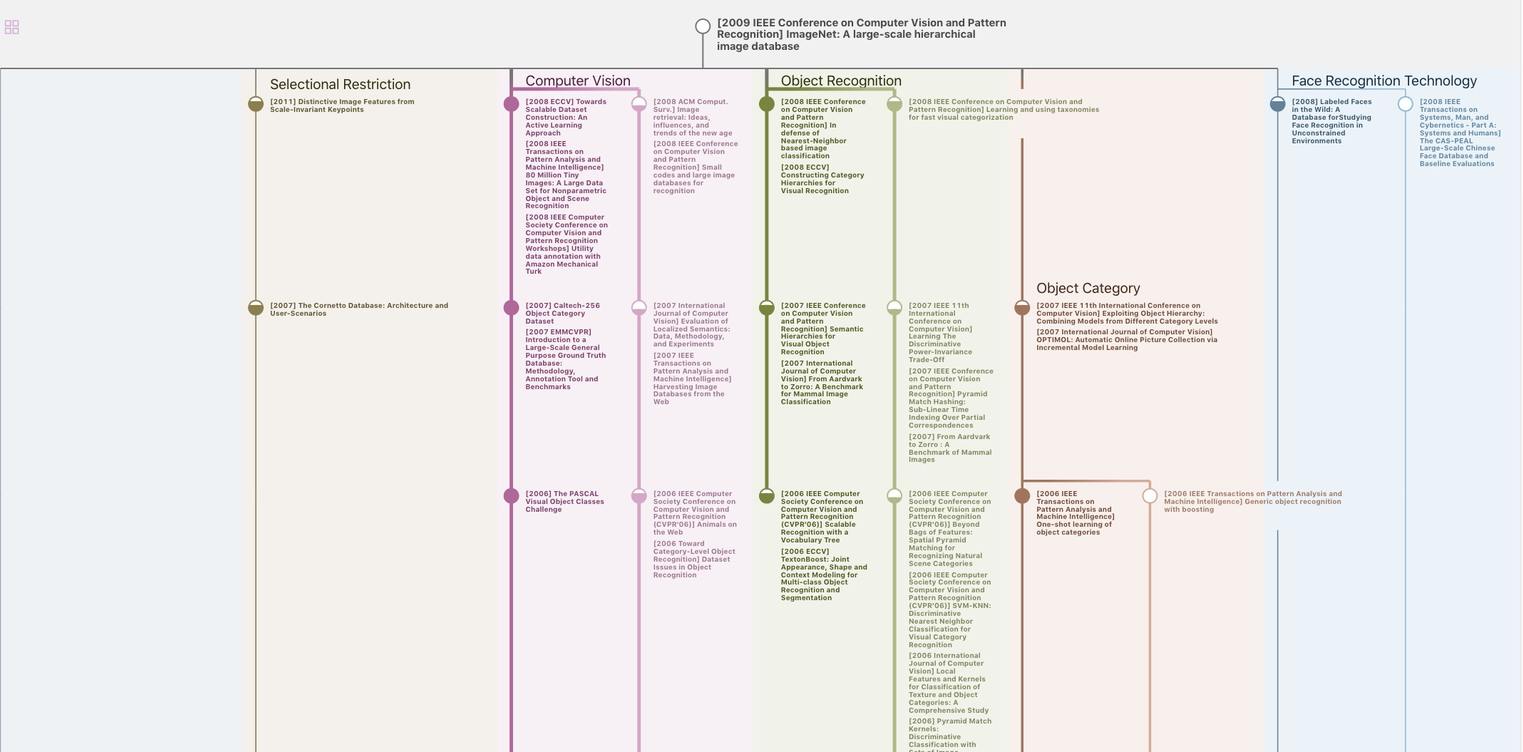
生成溯源树,研究论文发展脉络
Chat Paper
正在生成论文摘要