Quantum-Discrete-Map-Based Recurrent Neural Networks
arXiv (Cornell University)(2023)
摘要
Quantum machine learning is a rapidly growing domain and its potential has been explored for time series prediction and dynamics simulation in existing works. In this study, we propose a quantum-discrete-map-based recurrent neural network (QDM-RNN) to overcome the limitations posed by the circuit depth growing with the length of time series. From a discrete-dynamical perspective, quantum circuits are leveraged to build the discrete map and hence the discrete dynamical system. This approach involves measuring partial qubits to obtain historical information (memory) that is reused in the encoding layer of next time step, and measuring the other qubits to retrieve classical information as output. The nonlinear properties of the quantum discrete map make it appealing for embedding low-dimensional dynamics into higher dimensions, which is consistent with recurrent learning tricks. In numerical simulations, the QDM-RNN is implemented with one-feature datasets of waves and two-feature datasets of dynamics to demonstrate its capability. Our study introduces a new paradigm for quantum machine learning and highlights the potential of quantum computing in nonlinear dynamics.
更多查看译文
关键词
neural networks,quantum-discrete-map-based
AI 理解论文
溯源树
样例
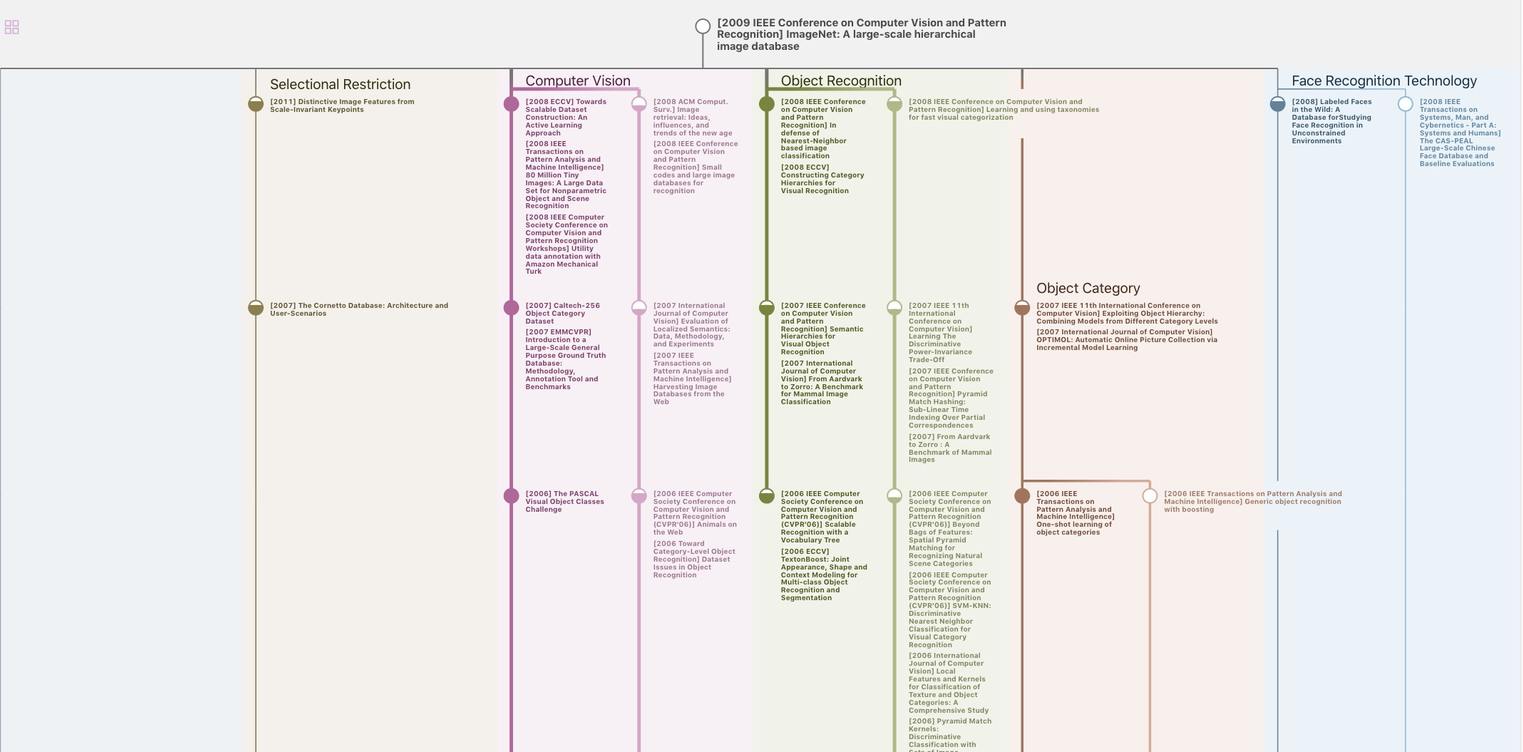
生成溯源树,研究论文发展脉络
Chat Paper
正在生成论文摘要